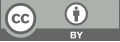
Enhancing Bank Credit Card Transaction Fraud Detection with Machine Learning Techniques
- 1 University of Maryland
* Author to whom correspondence should be addressed.
Abstract
Because of the increasing prevalence and sophistication of credit card theft, standard detection measures frequently fail. This study investigates the use of several machine learning algorithms to improve fraud detection in bank credit card transactions. The research aims to develop enhanced fraud detection systems by employing an integrated strategy involving data preprocessing, the application of various classification algorithms, and performance evaluation. The study thoroughly examines algorithms such as Random Forest, Logistic Regression, and Neural Networks, focusing on their predictive capabilities and practical applications. The findings indicate that machine learning provides a robust framework for increasing the accuracy and efficiency of fraud detection systems, consequently assisting financial institutions in protecting customer transactions and strengthening security measures. Moreover, by prioritizing robust model selection and feature engineering, banks can significantly enhance their fraud detection performance. This research could greatly influence how financial institutions handle fraud, potentially reducing losses, improving operational efficiency, and securing transaction environments. The insights gained from this study are also valuable for informing broader financial security strategies and guiding future research in the field.
Keywords
Credit Card Fraud, Machine Learning, Random Forest, Neural Network, XGBoost
[1]. uSwitch. (2019, December 29). Credit card fraud: the biggest card frauds in history. Retrieved from https://www.uswitch.com/credit-cards/guides/credit-card-fraud-the-biggest-card-frauds-in-history/
[2]. Yeh, I., & Lien, C. (2009). The comparisons of data mining techniques for the predictive accuracy of probability of default of credit card clients. Expert Systems With Applications, 36(2), 2473–2480. https://doi.org/10.1016/j.eswa.2007.12.020
[3]. Hastie, T., Tibshirani, R., & Friedman, J. H. (2009). The elements of statistical learning. In Springer series in statistics. https://doi.org/10.1007/978-0-387-84858-7
[4]. Lucas, Y., & Jurgovsky, J. (2010). Credit Card Fraud Detection Using Machine Learning: A survey. https://ar5iv.labs.arxiv.org/html/2010.06479#S1.SS2.SSS1
[5]. Credit card fraud Detection. (2018). [Dataset]. Kaggle. https://www.kaggle.com/datasets/mlg-ulb/creditcardfraud/data
[6]. Jolliffe IT, Cadima J. 2016Principal component analysis: a review andrecent developments. Phil.Trans.R.Soc.A374:20150202. http://dx.doi.org/10.1098/rsta.2015.0202
[7]. Kaplan, S. (n.d.). Choosing between data standardization vs normalization: Key considerations [Ensure optimal model. EML. https://enjoymachinelearning.com/blog/data-standardization-vs-normalization-in-data-science/
[8]. Saputra, A., & Suharjito, S. (2019). Fraud Detection using Machine Learning in e-Commerce. International Journal of Advanced Computer Science and Applications/International Journal of Advanced Computer Science & Applications, 10(9). https://doi.org/10.14569/ijacsa.2019.0100943
[9]. Krishna, M., & Praveenchandar, J. (2022). Comparative Analysis of Credit Card Fraud Detection using Logistic regression with Random Forest towards an Increase in Accuracy of Prediction. 2022 International Conference on Edge Computing and Applications (ICECAA). https://doi.org/10.1109/icecaa55415.2022.9936488
[10]. Chen, T., & Guestrin, C. (2016). XGBOOST: a scalable tree boosting System. https://arxiv.org/pdf/1603.02754
Cite this article
Zhu,Y. (2024). Enhancing Bank Credit Card Transaction Fraud Detection with Machine Learning Techniques. Advances in Economics, Management and Political Sciences,122,71-81.
Data availability
The datasets used and/or analyzed during the current study will be available from the authors upon reasonable request.
Disclaimer/Publisher's Note
The statements, opinions and data contained in all publications are solely those of the individual author(s) and contributor(s) and not of EWA Publishing and/or the editor(s). EWA Publishing and/or the editor(s) disclaim responsibility for any injury to people or property resulting from any ideas, methods, instructions or products referred to in the content.
About volume
Volume title: Proceedings of the 8th International Conference on Economic Management and Green Development
© 2024 by the author(s). Licensee EWA Publishing, Oxford, UK. This article is an open access article distributed under the terms and
conditions of the Creative Commons Attribution (CC BY) license. Authors who
publish this series agree to the following terms:
1. Authors retain copyright and grant the series right of first publication with the work simultaneously licensed under a Creative Commons
Attribution License that allows others to share the work with an acknowledgment of the work's authorship and initial publication in this
series.
2. Authors are able to enter into separate, additional contractual arrangements for the non-exclusive distribution of the series's published
version of the work (e.g., post it to an institutional repository or publish it in a book), with an acknowledgment of its initial
publication in this series.
3. Authors are permitted and encouraged to post their work online (e.g., in institutional repositories or on their website) prior to and
during the submission process, as it can lead to productive exchanges, as well as earlier and greater citation of published work (See
Open access policy for details).