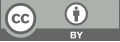
A Review of VWAP Trading Algorithms: Development, Improvements and Limitations
- 1 School of International Studies, Zhejiang University, Hangzhou, Zhejiang, China, 310027
* Author to whom correspondence should be addressed.
Abstract
This study explores the development and evolution of Volume-Weighted Average Price (VWAP) trading strategies in algorithmic trading. As algorithmic trading continues to transform the financial industry, optimizing execution strategies becomes crucial for minimizing trading costs and market impact. This research traces the historical development of VWAP, analyzes its integration into various trading strategies, and evaluates recent improvements in trade execution optimization. This paper first provide an overview of VWAP strategies and their significance in algorithmic trading. Then, it details the implementation of VWAP algorithms, including volume prediction techniques and execution methods. The study compares the performance of traditional VWAP approaches with advanced dynamic strategies, analyzing their effectiveness in different market conditions. Furthermore, a discussion is made on the limitations of conventional VWAP methods and an examination is conducted of recent advancements, including improved volume prediction models and adaptive execution algorithms. This research contributes to the growing field of algorithmic trading and offers valuable practical insights for traders and researchers aiming to optimize VWAP-based trading strategies.
Keywords
Dynamic Trading Strategies, VWAP Trading, Algorithmic Trading, Volume Prediction
[1]. Lehalle, C. A., & Laruelle, S. (Eds.). (2013). Market microstructure in practice. World Scientific.
[2]. Cartea, Á., Jaimungal, S., & Penalva, J. (2015). Algorithmic and high-frequency trading. Cambridge University Press.
[3]. Hendershott, T., Jones, C. M., & Menkveld, A. J. (2011). Does algorithmic trading improve liquidity?. The Journal of Finance, 66(1), 1-33.
[4]. Menkveld, A. J. (2013). High frequency trading and the new market makers. Journal of Financial Markets, 16(4), 712-740.
[5]. Berkowitz, S. A., Logue, D. E., & Noser Jr, E. A. (1988). The total cost of transactions on the NYSE. The Journal of Finance, 43(1), 97-112.
[6]. Madhavan, A. (2002). VWAP strategies. Trading, 2002(1), 32-39.
[7]. Bialkowski, J., Darolles, S., & Le Fol, G. (2008). Improving VWAP strategies: A dynamic volume approach. Journal of Banking & Finance, 32(9), 1709-1722.
[8]. Kakade, S. M., Kearns, M., Mansour, Y., & Ortiz, L. E. (2004, July). Competitive algorithms for VWAP and limit order trading. In Proceedings of the 5th ACM conference on Electronic commerce (pp. 189-198).
[9]. Brownlees, C. T., Cipollini, F., & Gallo, G. M. (2011). Intraday trading volume forecasts. Journal of Financial Econometrics, 9(3), 489-518.
[10]. Almgren, R., & Chriss, N. (2001). Optimal execution of portfolio transactions. Journal of Risk, 3, 5-40.
[11]. Kirilenko, A. A., & Lo, A. W. (2013). Moore's law versus Murphy's law: Algorithmic trading and its discontents. Journal of Economic Perspectives, 27(2), 51-72.
[12]. Gomber, P., Arndt, B., Lutat, M., & Uhle, T. (2011). High-frequency trading. Working Paper.
[13]. Kissell, R., & Malamut, R. (2005). Understanding the profit and loss distribution of trading algorithms. Trading, 2005(1), 41-49.
[14]. Madhavan, A. (2000). Market microstructure: A survey. Journal of financial markets, 3(3), 205-258.
[15]. Nevmyvaka, Y., Feng, Y., & Kearns, M. (2006, June). Reinforcement learning for optimized trade execution. In Proceedings of the 23rd international conference on Machine learning (pp. 673-680).
[16]. Perold, A. F. (1988). The implementation shortfall: Paper versus reality. Journal of Portfolio Management, 14(3), 4.
[17]. Humphery-Jenner, M. (2011). Optimal VWAP trading under noisy conditions. Journal of Banking & Finance, 35(9), 2319-2329.
[18]. Dixon, M., Klabjan, D., & Bang, J. H. (2017). Classification-based financial markets prediction using deep neural networks. Algorithmic Finance, 6(3-4), 67-77.
[19]. Cartea, Á., & Jaimungal, S. (2016). Incorporating order-flow into optimal execution. Mathematics and Financial Economics, 10(3), 339-364.
[20]. Buehler, H., Gonon, L., Teichmann, J., & Wood, B. (2019). Deep hedging. Quantitative Finance, 19(8), 1271-1291.
[21]. O'Hara, M. (2015). High frequency market microstructure. Journal of Financial Economics, 116(2), 257-270.
[22]. Hasbrouck, J., & Saar, G. (2013). Low-latency trading. Journal of Financial Markets, 16(4), 646-679.
[23]. Gomes, C., & Waelbroeck, H. (2015). Is market impact a measure of the information value of trades? Market response to liquidity vs. informed metaorders. Quantitative Finance, 15(5), 773-793.
[24]. Gatheral, J., & Schied, A. (2013). Dynamical models of market impact and algorithms for order execution. In Handbook on Systemic Risk (pp. 579-602). Cambridge University Press.
[25]. Cont, R., Kukanov, A., & Stoikov, S. (2014). The price impact of order book events. Journal of financial econometrics, 12(1), 47-88.
Cite this article
Chen,R. (2024). A Review of VWAP Trading Algorithms: Development, Improvements and Limitations. Advances in Economics, Management and Political Sciences,135,185-191.
Data availability
The datasets used and/or analyzed during the current study will be available from the authors upon reasonable request.
Disclaimer/Publisher's Note
The statements, opinions and data contained in all publications are solely those of the individual author(s) and contributor(s) and not of EWA Publishing and/or the editor(s). EWA Publishing and/or the editor(s) disclaim responsibility for any injury to people or property resulting from any ideas, methods, instructions or products referred to in the content.
About volume
Volume title: Proceedings of the 3rd International Conference on Financial Technology and Business Analysis
© 2024 by the author(s). Licensee EWA Publishing, Oxford, UK. This article is an open access article distributed under the terms and
conditions of the Creative Commons Attribution (CC BY) license. Authors who
publish this series agree to the following terms:
1. Authors retain copyright and grant the series right of first publication with the work simultaneously licensed under a Creative Commons
Attribution License that allows others to share the work with an acknowledgment of the work's authorship and initial publication in this
series.
2. Authors are able to enter into separate, additional contractual arrangements for the non-exclusive distribution of the series's published
version of the work (e.g., post it to an institutional repository or publish it in a book), with an acknowledgment of its initial
publication in this series.
3. Authors are permitted and encouraged to post their work online (e.g., in institutional repositories or on their website) prior to and
during the submission process, as it can lead to productive exchanges, as well as earlier and greater citation of published work (See
Open access policy for details).