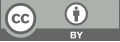
Analysing Melbourne's Property Market Dynamics: A Hedonic Price Prediction Approach Using Real Estate Data
- 1 New York University
* Author to whom correspondence should be addressed.
Abstract
In this article, Melbourne housing prices of existing homes are analysed with focus on many factors which affect property prices. Such factors include existence of spatial characteristics, type of property, mode of sale. By use of the data set, the research identifies broad findings relating to housing market. Such knowledge includes: seclusion of the detached houses, the role of number of rooms, and the role of the size of the land in determining the price. Thus, existence and distribution of these elements in relation to property prices may be revealed by regression analysis, which also shows main predictors and temporal tendencies within this process. The analysis offers insights into housing processes and challenges perceived by those interested in owning a home in the context of persisting growth of urbanization. The study admits its weaknesses, for example that it focused on Melbourne and only used data from the past even though this work makes a huge contribution towards understanding the housing market in Melbourne. Recommendations for future study are also made, with a stress on the fact that the range of variables must be expanded, the social consequences should be analysed, as well as the impact of technology on the housing market. Stakeholders who are interested in addressing housing affordability and sustainability in Melbourne and other metropolitan environments can use this detailed research as a basis to build their efforts
Keywords
Melbourne, House prices, Property values
[1]. Liu, G. (2022). Research on prediction and analysis of real estate market based on the multiple linear regression model. *Scientific Programming, 1–8. https://doi.org/10.1155/2022/5750354
[2]. Gurran, N., & Phibbs, P. (2013). Housing supply and urban planning reform: The recent Australian experience, 2003–2012. *International Journal of Housing Policy, 13*(4), 381–407. https://doi.org/10.1080/14616718.2013.840110
[3]. Phan, T. D. (2018). Housing price prediction using machine learning algorithms: The case of Melbourne city, Australia. In *2018 International Conference on Machine Learning and Data Engineering (iCMLDE)* (pp. 35–42). https://doi.org/10.1109/iCMLDE.2018.00017
[4]. Mouna, L. E., Silkan, H., Haynf, Y., Nann, M. F., & Tekouabou, S. C. K. (2023). A comparative study of urban house price prediction using machine learning algorithms. *E3S Web of Conferences, 418*, 03001. https://doi.org/10.1051/e3sconf/202341803001
[5]. Sari, R., Ewing, B. T., & Aydin, B. (2007). Macroeconomic variables and the housing market in Turkey. *Emerging Markets Finance and Trade, 43*(5), 5–19. https://doi.org/10.2753/REE1540-496X430501
[6]. Rosen, S. (1974). Hedonic prices and implicit markets: Product differentiation in pure competition. *Journal of Political Economy, 82*(1), 34–55. http://www.jstor.org/stable/1830899
[7]. Malpezzi, S. (2002). Hedonic pricing models: A selective and applied review. In T. O’Sullivan & K. Gibb (Eds.), *Housing economics and public policy* (1st ed., pp. 67–89). Wiley. https://doi.org/10.1002/9780470690680.ch5
[8]. Pawson, H., Milligan, V., & Yates, J. (2020). Housing policy in Australia: A reform agenda. In *Housing policy in Australia: A case for system reform* (pp. 339–358). Springer Nature. https://doi.org/10.1007/978-981-15-0780-9_10
[9]. Rey-Blanco, D., Zofío, J. L., & González-Arias, J. (2024). Improving hedonic housing price models by integrating optimal accessibility indices into regression and random forest analyses. *Expert Systems with Applications, 235*, 121059. https://doi.org/10.1016/j.eswa.2023.121059
[10]. Yoo, S., Im, J., & Wagner, J. E. (2012). Variable selection for hedonic model using machine learning approaches: A case study in Onondaga County, NY. *Landscape and Urban Planning, 107*(3), 293–306. https://doi.org/10.1016/j.landurbplan.2012.06.009
[11]. Yazdani, M. (2021). House price determinants and market segmentation in Boulder, Colorado: A hedonic price approach. *arXiv*. https://doi.org/10.48550/arXiv.2108.02442
[12]. Nguyen, M.-L. T. (2020). The hedonic pricing model applied to the housing market. *International Journal of Economics and Business Administration, 8*(3), 416–428. https://ijeba.com/journal/526
[13]. Soltani, A., Heydari, M., Aghaei, F., & Pettit, C. J. (2022). Housing price prediction incorporating spatio-temporal dependency into machine learning algorithms. *Cities, 131*, 103941. https://doi.org/10.1016/j.cities.2022.103941
[14]. Kaggle. (2024). Melbourne housing market. https://www.kaggle.com/datasets/anthonypino/melbourne-housing-market
[15]. Deshpande, A., & Kumar, M. (2018). *Artificial intelligence for big data: Complete guide to automating big data solutions using artificial intelligence techniques*. Packt Publishing Limited.
[16]. Refaat, M. (2007). *Data preparation for data mining using SAS*. Morgan Kaufmann Publishers.
[17]. Weinberg, S. L., Harel, D., & Abramowitz, S. K. (2023). *Statistics using R: An integrative approach* (2nd ed.). Cambridge University Press.
Cite this article
Zhao,X. (2024). Analysing Melbourne's Property Market Dynamics: A Hedonic Price Prediction Approach Using Real Estate Data. Advances in Economics, Management and Political Sciences,143,48-64.
Data availability
The datasets used and/or analyzed during the current study will be available from the authors upon reasonable request.
Disclaimer/Publisher's Note
The statements, opinions and data contained in all publications are solely those of the individual author(s) and contributor(s) and not of EWA Publishing and/or the editor(s). EWA Publishing and/or the editor(s) disclaim responsibility for any injury to people or property resulting from any ideas, methods, instructions or products referred to in the content.
About volume
Volume title: Proceedings of ICFTBA 2024 Workshop: Finance's Role in the Just Transition
© 2024 by the author(s). Licensee EWA Publishing, Oxford, UK. This article is an open access article distributed under the terms and
conditions of the Creative Commons Attribution (CC BY) license. Authors who
publish this series agree to the following terms:
1. Authors retain copyright and grant the series right of first publication with the work simultaneously licensed under a Creative Commons
Attribution License that allows others to share the work with an acknowledgment of the work's authorship and initial publication in this
series.
2. Authors are able to enter into separate, additional contractual arrangements for the non-exclusive distribution of the series's published
version of the work (e.g., post it to an institutional repository or publish it in a book), with an acknowledgment of its initial
publication in this series.
3. Authors are permitted and encouraged to post their work online (e.g., in institutional repositories or on their website) prior to and
during the submission process, as it can lead to productive exchanges, as well as earlier and greater citation of published work (See
Open access policy for details).