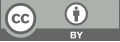
Fake News Detection and Analysis
- 1 University of Melbourne
- 2 Shihezi University
- 3 Chongqing University
- 4 University of Southern California
* Author to whom correspondence should be addressed.
Abstract
The popularized use of social media accelerates the spreading of fake news. The overwhelming amount of fake news was a severe social issue during the 2016 presidential election and the first outbreak of Coronavirus in 2020. As controlling the spread of fake news is not practically workable, the detection of fake news is significantly valuable to solve this issue. In this paper, we conduct experiments to discover the effect of contextualized embedding of news content on counterfeit news detection. We also explore the features of fake news through two aspects: clickbait and sentiment.
Keywords
fake news detection, deep learning, contextualized embedding
[1]. K. Shu, A. Sliva, S. Wang, J. Tang, and H. Liu, “Fake news detection on social media: A data mining perspective,” ACM SIGKDD explorations newsletter, vol. 19, no. 1, pp. 22–36, 2017.
[2]. E. Jardine, “Beware fake news,” Governing Cyberspace during a Crisis in Trust essay. Waterloo, ON: CIGI. www. cigionline. org/articles/beware-fake-news, 2019.
[3]. P. N. Howard, G. Bolsover, B. Kollanyi, S. Bradshaw, and L.-M. Neudert, “Junk news and bots during the us election: What were michigan voters sharing over twitter,” CompProp, OII, Data Memo, vol. 1, 2017.
[4]. D. W. Otter, J. R. Medina, and J. K. Kalita, “A survey of the usages of deep learning for natural language processing,” IEEE Trans. Neural Netw. Learn. Syst., vol. 32, no. 2, pp. 604–624, Feb. 2021.
[5]. N. Kalchbrenner, E. Grefenstette, and P. Blunsom, “A convolutional neural network for modelling sentences,” arXiv preprint arXiv:1404.2188, 2014.
[6]. L. Yao, C. Mao, and Y. Luo, “Graph convolutional networks for text classification,” in Proceedings of the AAAI conference on artificial intelligence, vol. 33, no. 01, 2019, pp. 7370–7377.
[7]. F. Monti, F. Frasca, D. Eynard, D. Mannion, and M. M. Bronstein, “Fake news detection on social media using geometric deep learning,” arXiv preprint arXiv:1902.06673, 2019.
[8]. R. Oshikawa, J. Qian, and W. Y. Wang, “A survey on natural language processing for fake news detection,” arXiv preprint arXiv:1811.00770, 2018.
[9]. W. Shishah, “Fake news detection using bert model with joint learning,” Arabian Journal for Science and Engineering, vol. 46, no. 9, pp. 9115–9127, 2021.
[10]. I. Rish et al., “An empirical study of the naive bayes classifier,” in IJCAI 2001 workshop on empirical methods in artificial intelligence, vol. 3, no. 22, 2001, pp. 41–46.
[11]. L. Jiang, C. Li, S. Wang, and L. Zhang, “Deep feature weighting for naive bayes and its application to text classification,” Engineering Applications of Artificial Intelligence, vol. 52, pp. 26–39, 2016.
[12]. A. M. Kibriya, E. Frank, B. Pfahringer, and G. Holmes, “Multinomial naive bayes for text categorization revisited,” in Australasian Joint Conference on Artificial Intelligence. Springer, 2004, pp. 488–499.
[13]. S.-B. Kim, K.-S. Han, H.-C. Rim, and S. H. Myaeng, “Some effective techniques for naive bayes text classification,” IEEE transactions on knowledge and data engineering, vol. 18, no. 11, pp. 1457–1466, 2006.
[14]. W. Zhang and F. Gao, “An improvement to naive bayes for text classification,” Procedia Engineering, vol. 15, pp. 2160–2164, 2011.
[15]. A. H. Wang, “Don’t follow me: Spam detection in twitter,” in 2010 international conference on security and cryptography (SECRYPT). IEEE, 2010, pp. 1–10.
[16]. K. Chakraborty, S. Bhatia, S. Bhattacharyya, J. Platos, R. Bag, and A. E. Hassanien, “Sentiment analysis of covid-19 tweets by deep learning classifiers—a study to show how popularity is affecting accuracy in social media,” Applied Soft Computing, vol. 97, p. 106754, 2020.
[17]. H. Ahmed, I. Traore, and S. Saad, “Detecting opinion spams and fake news using text classification,” Security and Privacy, vol. 1, no. 1, p. e9, 2018.
[18]. H. Ahmed, I. Traore, and S. Saad, “Detection of online fake news using n-gram analysis and machine learning techniques,” in International conference on intelligent, secure, and dependable systems in distributed and cloud environments. Springer, 2017, pp. 127–138.
[19]. G. Singh, B. Kumar, L. Gaur, and A. Tyagi, “Comparison between multinomial and bernoulli naïve bayes for text classification,” in 2019 International Conference on Automation, Computational and Technology Management (ICACTM). IEEE, 2019, pp. 593–596.
[20]. J. Devlin, M.-W. Chang, K. Lee, and K. Toutanova, “Bert: Pre-training of deep bidirectional transformers for language understanding,” arXiv preprint arXiv:1810.04805, 2018.
[21]. Y. Liu, M. Ott, N. Goyal, J. Du, M. Joshi, D. Chen, O. Levy, M. Lewis, L. Zettlemoyer, and V. Stoyanov, “Roberta: A robustly optimized bert pretraining approach,” arXiv preprint arXiv:1907.11692, 2019.
[22]. J. Chung, C. Gulcehre, K. Cho, and Y. Bengio, “Empirical evaluation of gated recurrent neural networks on sequence modeling,” arXiv preprint arXiv:1412.3555, 2014.
[23]. C. Raffel, N. Shazeer, A. Roberts, K. Lee, S. Narang, M. Matena, Y. Zhou, W. Li, P. J. Liu et al., “Exploring the limits of transfer learning with a unified text-to-text transformer.” J. Mach. Learn. Res., vol. 21, no. 140, pp. 1–67, 2020.
[24]. P. Bourgonje, J. Moreno Schneider, and G. Rehm, “From clickbait to fake news detection: An approach based on detecting the stance of headlines to articles,” in Proceedings of the 2017 EMNLP Workshop: Natural Language Processing meets Journalism. Copenhagen, Denmark: Association for Computational Linguistics, Sep. 2017, pp. 84–89. [Online]. Available: https://aclanthology.org/W17-4215
[25]. S. Bowman and C. Willis, We media. How audiences are shaping the future of news and information, 66, 2003.
[26]. D. Gillmor, We the media: Grassroots journalism by the people, for the people. Reilly Media, Inc, 2006.
[27]. J. M. Scacco and A. Muddiman, “Investigating the influence of “clickbait” news headlines,” Engaging News Project Report, 2016.
[28]. A. Chakraborty, B. Paranjape, S. Kakarla, and N. Ganguly, “Stop clickbait: Detecting and preventing clickbaits in online news media,” in 2016 IEEE/ACM International Conference on Advances in Social Networks Analysis and Mining (ASONAM). IEEE, Aug. 2016.
[29]. M. A. Alonso, D. Vilares, C. Gómez-Rodríguez, and J. Vilares, “Sentiment analysis for fake news detection,” Electronics (Basel), vol. 10, no. 11, p. 1348, Jun. 2021.
[30]. O. Ajao, D. Bhowmik, and S. Zargari, “Sentiment aware fake news detection on online social networks,” in ICASSP 2019 - 2019 IEEE International Conference on Acoustics, Speech and Signal Processing (ICASSP). IEEE, May 2019.
[31]. K. E. McIntyre and R. Gibson, “Positive news makes readers feel good: A “silver-lining” approach to negative news can attract audiences,” South. Commun. J., vol. 81, no. 5, pp. 304–315, Oct. 2016.
Cite this article
Zhang,B.;Dai,C.;Deng,Z.;Jiang,Z. (2023). Fake News Detection and Analysis. Communications in Humanities Research,7,22-30.
Data availability
The datasets used and/or analyzed during the current study will be available from the authors upon reasonable request.
Disclaimer/Publisher's Note
The statements, opinions and data contained in all publications are solely those of the individual author(s) and contributor(s) and not of EWA Publishing and/or the editor(s). EWA Publishing and/or the editor(s) disclaim responsibility for any injury to people or property resulting from any ideas, methods, instructions or products referred to in the content.
About volume
Volume title: Proceedings of the 4th International Conference on Educational Innovation and Philosophical Inquiries
© 2024 by the author(s). Licensee EWA Publishing, Oxford, UK. This article is an open access article distributed under the terms and
conditions of the Creative Commons Attribution (CC BY) license. Authors who
publish this series agree to the following terms:
1. Authors retain copyright and grant the series right of first publication with the work simultaneously licensed under a Creative Commons
Attribution License that allows others to share the work with an acknowledgment of the work's authorship and initial publication in this
series.
2. Authors are able to enter into separate, additional contractual arrangements for the non-exclusive distribution of the series's published
version of the work (e.g., post it to an institutional repository or publish it in a book), with an acknowledgment of its initial
publication in this series.
3. Authors are permitted and encouraged to post their work online (e.g., in institutional repositories or on their website) prior to and
during the submission process, as it can lead to productive exchanges, as well as earlier and greater citation of published work (See
Open access policy for details).