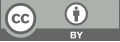
Student Loan: Topic Modelling with Twitter Data
- 1 Dimensions International School
- 2 UWC changshu
- 3 The Winchendon School
* Author to whom correspondence should be addressed.
Abstract
The study is about citizens’ opinions on student loans by analyzing Twitter reactions to Biden’s student loan cancellation project using the machine-driven classification of open-ended response (MDCOR) and found it saved research time, increased efficiency, and ensured authenticity and objectivity of data. After putting data into the application, we found that using five analysis topics is appropriate. The topic’s content can be predicted by seeking the relevant word for each case. The analysis of five issues related to student loans shows mixed opinions about the impact of loan forgiveness, with some key terms such as “predatory” and “donation” being significant. At the same time, some topics are not directly related to the issue.
Keywords
student loan, topic modeling, text mining, twitter
[1]. Office of US Department of Education. (n.d.). Federal student loans for college or career school are an investment in your future. Federal Student Aid. Retrieved April 15, 2023, from https://studentaid.gov/understand-aid/types/loans
[2]. Canché, M. S. G. (2023). Machine-driven classification of open-ended responses (MDCOR): An analytic framework and no-code, free software application to classify longitudinal and cross-sectional text responses in survey and social media research. Expert Systems with Applications, 215, 119265.
[3]. IQVIA company. (n.d.). What is text mining, text analytics, and Natural Language Processing? What is Text Mining, Text Analytics and Natural Language Processing? Linguamatics. Retrieved April 15, 2023, from https://www.linguamatics.com/what-text-mining-text-analytics-and-natural-language-processing
[4]. Robinson, J. S. and D. (n.d.). 6 topic modeling: Text mining with R. 6 Topic modeling | Text Mining with R. Retrieved April 15, 2023, from https://www.tidytextmining.com/topicmodeling.html
[5]. Phat Jotikabukkana. (n.d.). Social media text classification by enhancing well-formed text trained ... Retrieved April 14, 2023, from https://www.researchgate.net/publication/316030904_Social_Media_Text_Classification_by_Enhancing_Well-Formed_Text_Trained_Model
Cite this article
Su,P.;Wang,T.;Pan,Y. (2023). Student Loan: Topic Modelling with Twitter Data. Communications in Humanities Research,11,52-58.
Data availability
The datasets used and/or analyzed during the current study will be available from the authors upon reasonable request.
Disclaimer/Publisher's Note
The statements, opinions and data contained in all publications are solely those of the individual author(s) and contributor(s) and not of EWA Publishing and/or the editor(s). EWA Publishing and/or the editor(s) disclaim responsibility for any injury to people or property resulting from any ideas, methods, instructions or products referred to in the content.
About volume
Volume title: Proceedings of the 4th International Conference on Educational Innovation and Philosophical Inquiries
© 2024 by the author(s). Licensee EWA Publishing, Oxford, UK. This article is an open access article distributed under the terms and
conditions of the Creative Commons Attribution (CC BY) license. Authors who
publish this series agree to the following terms:
1. Authors retain copyright and grant the series right of first publication with the work simultaneously licensed under a Creative Commons
Attribution License that allows others to share the work with an acknowledgment of the work's authorship and initial publication in this
series.
2. Authors are able to enter into separate, additional contractual arrangements for the non-exclusive distribution of the series's published
version of the work (e.g., post it to an institutional repository or publish it in a book), with an acknowledgment of its initial
publication in this series.
3. Authors are permitted and encouraged to post their work online (e.g., in institutional repositories or on their website) prior to and
during the submission process, as it can lead to productive exchanges, as well as earlier and greater citation of published work (See
Open access policy for details).