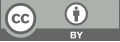
To Study the Dynamics of Demand and Consumption Patterns of Wellness Tea in Different Provinces of China
- 1 Chongqing Normal University
* Author to whom correspondence should be addressed.
Abstract
In the present social context where health awareness is on the rise Chinese consumers’ appetite for healthy beverages is experiencing a rapid upward trend. Wellness tea beverages, which integrate traditional wellness concepts with modern beverage culture, are increasingly popular among consumers due to their potential health advantages.. This report offers an in-depth analysis of the dynamic changes in demand and consumption patterns of wellness tea across different provinces in China. By comprehensively dissecting the sales volume, price, and consumption behaviors of branded wellness tea in various cities and stores on the Taobao platform, it aims to disclose market trends and construct a regression forecast model for sales volume. The results indicate that the price factor (both pre-coupon and post-coupon prices) is the most decisive element influencing sales volume. Geographical regional disparities also exert a certain impact on sales volume, while macroeconomic indicators such as per capita GDP have a relatively minor impact. These discoveries not only provide decision support for market participants in the wellness tea industry, but also provide valuable reference information for policymakers, thereby facilitating the healthy progression of the industry and further tapping into the market potential.
Keywords
Wellness Tea, Volume Forecasting, Price Factors, Geographic Regions, Consumer Behavior
[1]. Montgomery D C, Peck E A, Vining G G. Introduction to linear regression analysis[M]. John Wiley & Sons, 2021.
[2]. Tranmer M, Elliot M. Multiple linear regression[J]. The Cathie Marsh Centre for Census and Survey Research (CCSR), 2008, 5(5):1-5.
[3]. Zhang L, WeiX, LuJ, et al. Lasso regression: From explanation to prediction[J]. Advances in Psychological Science, 2020, 28(10):1777.
[4]. Marill K A. Advanced statistics: linear regression, part ii: multiple linear regression[J]. Academic emergency medicine, 2004, 11(1):94-102.
[5]. Dong G, Liu H. Feature engineering for machine learning and data analytics[M]. CRC press, 2018.
[6]. Ranstam J, Cook J A. Lasso regression[J]. Journal of British Surgery, 2018, 105(10): 1348-1348.
[7]. ArashiM, RoozbehM, HamzahN A, et al. Ridge regression and its applications in genetic studies[J]. Plos one, 2021, 16(4):e0245376.
[8]. Segal M R. Machine learning benchmarks and random forest regression[J]. 2004.
[9]. Prettenhofer P, Louppe G. Gradient boosted regression trees in scikit-learn[C]//PyData 2014. 2014.
[10]. Zien A, Krämer N, Sonnenburg S, et al. The feature importance ranking measure[C]// Machine Learning and Knowledge Discovery in Databases: European Conference, ECML PKDD 2009, Bled, Slovenia, September 7-11, 2009, Proceedings, Part II 20. Springer, 2009: 694-709.
Cite this article
Ding,Y. (2025). To Study the Dynamics of Demand and Consumption Patterns of Wellness Tea in Different Provinces of China. Lecture Notes in Education Psychology and Public Media,81,79-85.
Data availability
The datasets used and/or analyzed during the current study will be available from the authors upon reasonable request.
Disclaimer/Publisher's Note
The statements, opinions and data contained in all publications are solely those of the individual author(s) and contributor(s) and not of EWA Publishing and/or the editor(s). EWA Publishing and/or the editor(s) disclaim responsibility for any injury to people or property resulting from any ideas, methods, instructions or products referred to in the content.
About volume
Volume title: Proceedings of the 3rd International Conference on Social Psychology and Humanity Studies
© 2024 by the author(s). Licensee EWA Publishing, Oxford, UK. This article is an open access article distributed under the terms and
conditions of the Creative Commons Attribution (CC BY) license. Authors who
publish this series agree to the following terms:
1. Authors retain copyright and grant the series right of first publication with the work simultaneously licensed under a Creative Commons
Attribution License that allows others to share the work with an acknowledgment of the work's authorship and initial publication in this
series.
2. Authors are able to enter into separate, additional contractual arrangements for the non-exclusive distribution of the series's published
version of the work (e.g., post it to an institutional repository or publish it in a book), with an acknowledgment of its initial
publication in this series.
3. Authors are permitted and encouraged to post their work online (e.g., in institutional repositories or on their website) prior to and
during the submission process, as it can lead to productive exchanges, as well as earlier and greater citation of published work (See
Open access policy for details).