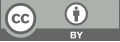
Exploring AI's Role in Enhancing Risk Assessment Models in Financial Quantitative Trading
- 1 Shenzhen CIMC Industry & City Development Group Co., Ltd.
* Author to whom correspondence should be addressed.
Abstract
The application of Artificial Intelligence (AI) algorithms to financial risk management has fundamentally transformed the nature of risk and the tools being used to control and manage it. This paper examines how AI is deployed to manage market risk, including volatility forecasting, portfolio optimisation, stress testing, and sentiment analysis. While many of these models are well-accepted across the industry and underpin many front-office and risk-management processes, they are often limited in dynamic market environments where traditional linearity doesn’t exist and real-time data is king. AI has brought advanced tools that can be applied to large and complex data sets, and that adapt quickly as market conditions change. Reinforcement learning algorithms are now deployed to optimise portfolio weights dynamically. Natural-language processing is also used to augment sentiment analysis, which can be leveraged to assess potential risk. Other forms of AI can be deployed to address challenges that come with more advanced AI models. Explainable AI models provide the opportunity to produce financial outcomes that are more transparent and less opaque while also helping to validate regulatory compliance. Analyzing the applications of AI case studies sheds light on why financial professionals must continue to embrace AI tools, many of which are designed to help them manage risk in an increasingly volatile market environment.
Keywords
artificial intelligence, financial risk management, volatility prediction, portfolio optimization
[1]. El Hajj, M., & Hammoud, J. (2023). Unveiling the influence of artificial intelligence and machine learning on financial markets: A comprehensive analysis of AI applications in trading, risk management, and financial operations. Journal of Risk and Financial Management, 16(10), 434.
[2]. Giudici, P., Centurelli, M., & Turchetta, S. (2024). Artificial Intelligence risk measurement. Expert Systems with Applications, 235, 121220.
[3]. Vaswani, A., Shazeer, N., Parmar, N., Uszkoreit, J., Jones, L., Gomez, A. N., ... & Polosukhin, I. (2017). Attention is all you need. NIPS. arXiv preprint arXiv:1706.03762.
[4]. Schuett, J. (2023). Risk management in the artificial intelligence act. European Journal of Risk Regulation, 1–19.
[5]. Wong, L.-W., et al. (2024). Artificial intelligence-driven risk management for enhancing supply chain agility: A deep-learning-based dual-stage PLS-SEM-ANN analysis. International Journal of Production Research, 62(15), 5535–5555.
[6]. Doumpos, M., et al. (2023). Operational research and artificial intelligence methods in banking. European Journal of Operational Research, 306(1), 1–16.
[7]. Saffari, G. (2024). Ranking of business risks by artificial intelligence and multi-criteria decision making. International Journal of Industrial Engineering and Operational Research, 6(2), 1–16.
[8]. Javaid, H. A. (2024). AI-driven predictive analytics in finance: Transforming risk assessment and decision-making. Advances in Computer Sciences, 7(1).
[9]. Giudici, P., & Raffinetti, E. (2023). SAFE artificial intelligence in finance. Finance Research Letters, 56, 104088.
[10]. Hong, H. (2024). Construction and application of financial risk early warning model based on machine learning. Journal of Electrical Systems, 20(7s), 3016–3030.
Cite this article
Leng,R. (2024). Exploring AI's Role in Enhancing Risk Assessment Models in Financial Quantitative Trading. Journal of Applied Economics and Policy Studies,12,1-5.
Data availability
The datasets used and/or analyzed during the current study will be available from the authors upon reasonable request.
Disclaimer/Publisher's Note
The statements, opinions and data contained in all publications are solely those of the individual author(s) and contributor(s) and not of EWA Publishing and/or the editor(s). EWA Publishing and/or the editor(s) disclaim responsibility for any injury to people or property resulting from any ideas, methods, instructions or products referred to in the content.
About volume
Journal:Journal of Applied Economics and Policy Studies
© 2024 by the author(s). Licensee EWA Publishing, Oxford, UK. This article is an open access article distributed under the terms and
conditions of the Creative Commons Attribution (CC BY) license. Authors who
publish this series agree to the following terms:
1. Authors retain copyright and grant the series right of first publication with the work simultaneously licensed under a Creative Commons
Attribution License that allows others to share the work with an acknowledgment of the work's authorship and initial publication in this
series.
2. Authors are able to enter into separate, additional contractual arrangements for the non-exclusive distribution of the series's published
version of the work (e.g., post it to an institutional repository or publish it in a book), with an acknowledgment of its initial
publication in this series.
3. Authors are permitted and encouraged to post their work online (e.g., in institutional repositories or on their website) prior to and
during the submission process, as it can lead to productive exchanges, as well as earlier and greater citation of published work (See
Open access policy for details).