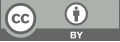
Exploring Multi-Armed Bandit algorithms: Performance analysis in dynamic environments
- 1 University of California San Diego
* Author to whom correspondence should be addressed.
Abstract
The Multi-armed Bandit algorithm, a proficient solver of the exploration-and-exploitation trade-off predicament, furnishes businesses with a robust tool for resource allocation that predominantly aligns with customer preferences. However, varying Multi-armed Bandit algorithm types exhibit dissimilar performance characteristics based on contextual variations. Hence, a series of experiments is imperative, involving alterations to input values across distinct algorithms. Within this study, three specific algorithms were applied, Explore-then-commit (ETC), Upper Confident Bound (UCB) and its asymptotically optimal variant, and Thompson Sampling (TS), to the extensively utilized MovieLens dataset. This application aimed to gauge their effectiveness comprehensively. The algorithms were translated into executable code, and their performance was visually depicted through multiple figures. Through cumulative regret tracking within defined conditions, algorithmic performance was scrutinized, laying the groundwork for subsequent parameter-based comparisons. A dedicated experimentation framework was devised to evaluate the robustness of each algorithm, involving deliberate parameter adjustments and tailored experiments to elucidate distinct performance nuances. The ensuing graphical depictions distinctly illustrated Thompson Sampling's persistent minimal regrets across most scenarios. UCB algorithms displayed steadfast stability. ETC manifested excellent performance with a low number of runs but escalate significantly along the number of runs growing. It also warranting constraints on exploratory phases to mitigate regrets. This investigation underscores the efficacy of Multi-armed Bandit algorithms while elucidating their nuanced behaviors within diverse contextual contingencies.
Keywords
multi-armed bandit algorithm, ETC, UCB, TS
[1]. Saboo A R Kumar V and Park I 2016 Using Big Data to Model Time-Varying Effects for Marketing Resource (Re)Allocation MIS Quarterly, 40(4) p 911–940
[2]. Zhang S Liao P., Ye H. Q., and Zhou Z. 2022 Dynamic Marketing Resource Allocation with Two-Stage Decisions Journal of Theoretical and Applied Electronic Commerce Research Multidisciplinary Digital Publishing Institute https://doi.org/10.3390/jtaer17010017
[3]. Vermorel J. and Mohri M. 2005 Multi-armed Bandit Algorithms and Empirical Evaluation Machine Learning: ECML 2005. ECML 2005 Lecture Notes in Computer Science() vol 3720 https://doi.org/10.1007/11564096_42
[4]. Lattimore, T. and Szepesvári, C. 2020 Bandit Algorithms Cambridge University Press p 90-91 459-461
[5]. Jouini W., Ernst D., Moy C., and Palicot J. 2010 Upper Confidence Bound Based Decision Making Strategies and Dynamic Spectrum Access IEEE International Conference on Communications p 1-5
[6]. Gupta S., Chaudhari S., Joshi G., and Yağan O. 2021 Multi-Armed Bandits With Correlated Arms in IEEE Transactions on Information Theory vol. 67 no. 10 p 6711-6732
[7]. Harper F. M. and Konstan J. A. 2015 The MovieLens Datasets: History and Context. ACM Transactions on Interactive Intelligent Systems (TiiS) 5 4 Article 19 19 pages DOI=http://dx.doi.org/10.1145/2827872
[8]. Henderson P et al 2017 Deep Reinforcement Learning that Matters CoRR abs/1709.06560
[9]. Madhyastha P Jain R 2019 On model stability as a function of random seed arXiv preprint arXiv:1909.10447
[10]. Colas C Sigaud O Oudeyer P Y 2018 How many random seeds? statistical power analysis in deep reinforcement learning experiments arXiv preprint arXiv:1806.08295
Cite this article
Li,L. (2024). Exploring Multi-Armed Bandit algorithms: Performance analysis in dynamic environments. Applied and Computational Engineering,34,252-259.
Data availability
The datasets used and/or analyzed during the current study will be available from the authors upon reasonable request.
Disclaimer/Publisher's Note
The statements, opinions and data contained in all publications are solely those of the individual author(s) and contributor(s) and not of EWA Publishing and/or the editor(s). EWA Publishing and/or the editor(s) disclaim responsibility for any injury to people or property resulting from any ideas, methods, instructions or products referred to in the content.
About volume
Volume title: Proceedings of the 2023 International Conference on Machine Learning and Automation
© 2024 by the author(s). Licensee EWA Publishing, Oxford, UK. This article is an open access article distributed under the terms and
conditions of the Creative Commons Attribution (CC BY) license. Authors who
publish this series agree to the following terms:
1. Authors retain copyright and grant the series right of first publication with the work simultaneously licensed under a Creative Commons
Attribution License that allows others to share the work with an acknowledgment of the work's authorship and initial publication in this
series.
2. Authors are able to enter into separate, additional contractual arrangements for the non-exclusive distribution of the series's published
version of the work (e.g., post it to an institutional repository or publish it in a book), with an acknowledgment of its initial
publication in this series.
3. Authors are permitted and encouraged to post their work online (e.g., in institutional repositories or on their website) prior to and
during the submission process, as it can lead to productive exchanges, as well as earlier and greater citation of published work (See
Open access policy for details).