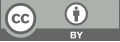
Application analysis of financial data mining in investment decision
- 1 School of Fuzhou University, 2 Wulong Jiangbei Avenue, Fuzhou University Town, Fuzhou City, Fujian Province
* Author to whom correspondence should be addressed.
Abstract
Amidst the escalating complexities that define the contemporary financial market and the rapid proliferation of information, traditional methods of formulating investment decisions confront increasingly formidable challenges. In response to these intricate dynamics, the realm of financial data mining has emerged as a prominent avenue of scholarly investigation within the investment domain. This paper's fundamental objective is to conduct a comprehensive retrospective analysis of the diverse applications of financial data mining in the context of investment decision-making.This scholarly pursuit entails a meticulous synthesis of existing academic inquiries, concurrently proposing potential avenues for future advancements in this field. By undertaking this academic endeavor, the paper strives to make substantive contributions to the refinement of methodologies essential for adeptly navigating the multifaceted landscape of modern investments. As the financial landscape continues to evolve, this study aspires to offer insights that not only enhance the efficacy of investment strategies but also foster a deeper understanding of the intricate interplay between data mining techniques and decision-making processes. Through the synthesis of empirical findings and theoretical perspectives, this paper seeks to underscore the pertinence of leveraging data-driven approaches in investment practices, thereby promoting a more informed and sophisticated investment landscape.
Keywords
Data Mining; Machine Learning; Deep Learning; Time Series Model
[1]. Zhu, T. and Luo, S. (2015) Application of data mining technology in university management decision. Computer age, (3): 39 - 40.
[2]. Gong, G. (2016) Discussion on the application mode of data mining in the management decision of university students. Information and computer, (3): 135 - 136.
[3]. Yan, F. (2018) Empirical analysis of Chinese value stock investment. Time finance, 709(27): 162 - 166.
[4]. Jin, Y. (2017) Stock market analysis and prediction based on data mining technology. Jilin University of Finance and Economics.
[5]. Zhang, W. (2022) Stock time series prediction based on long short-term memory neural network. Information and Computers (Theory), (9): 68 - 72.
[6]. Chakole, J. and Kurhekar, M. (2020) Trend Following Deep Q-Learning Strategy for Stock Trading. Expert Systems, 37.
[7]. He, Q. (2022) Deep reinforcement learning stock trading strategies combined with trends. Computer science and applications, (3): 673 - 681.
[8]. Tang, Y. (2022) Research on optimal portfolio based on graph Convolutional neural network. Lanzhou University of Finance and Economics.
[9]. Wang, D. and Zhou, Y. (2023) Can machine learning methods identify the probability of systemic financial risk in China? Financial market research, 134: 48 - 58.
[10]. Fan, X. (2006) Behind prosperity -- the essence, measurement and management of financial systemic risk. China Finance Press, Beijing.
[11]. Laeven L. and Valencia F. (2013) Systemic banking crises database. IMF Economic Review, 61(2): 225 - 270.
[12]. Wang, T. and Yang, W. (2021) A review of text sentiment analysis methods. Computer engineering and applications, (12): 11 - 24.
[13]. Xiong, Y. (2021) Research on multi-channel investor sentiment analysis for stock market. Southwest University of Finance and Economics.
[14]. Tao, J. (2022) Research on high-transfer stock prediction based on three-stage feature selection and Enhanced GBDT-Logit: A case study of China A-share market. Zhejiang Gongshang University.
Cite this article
Zeng,R. (2024). Application analysis of financial data mining in investment decision. Applied and Computational Engineering,44,1-7.
Data availability
The datasets used and/or analyzed during the current study will be available from the authors upon reasonable request.
Disclaimer/Publisher's Note
The statements, opinions and data contained in all publications are solely those of the individual author(s) and contributor(s) and not of EWA Publishing and/or the editor(s). EWA Publishing and/or the editor(s) disclaim responsibility for any injury to people or property resulting from any ideas, methods, instructions or products referred to in the content.
About volume
Volume title: Proceedings of the 2023 International Conference on Machine Learning and Automation
© 2024 by the author(s). Licensee EWA Publishing, Oxford, UK. This article is an open access article distributed under the terms and
conditions of the Creative Commons Attribution (CC BY) license. Authors who
publish this series agree to the following terms:
1. Authors retain copyright and grant the series right of first publication with the work simultaneously licensed under a Creative Commons
Attribution License that allows others to share the work with an acknowledgment of the work's authorship and initial publication in this
series.
2. Authors are able to enter into separate, additional contractual arrangements for the non-exclusive distribution of the series's published
version of the work (e.g., post it to an institutional repository or publish it in a book), with an acknowledgment of its initial
publication in this series.
3. Authors are permitted and encouraged to post their work online (e.g., in institutional repositories or on their website) prior to and
during the submission process, as it can lead to productive exchanges, as well as earlier and greater citation of published work (See
Open access policy for details).