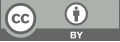
Bird image classification based on improved ResNet-152 image classification model
- 1 Macau University of Science and Technology
* Author to whom correspondence should be addressed.
Abstract
Bird image classification is an important research direction in the field of computer vision, and its main purpose is to automatically identify bird images through computers to achieve bird classification, recognition and monitoring. Birds are an important part of the planet's biodiversity and have an important impact on the balance of ecosystems and human survival. ResNet-152 is a deep convolutional neural network model, which is the deepest model in the ResNet family, with a depth of 152 layers. The model mainly solves the problems of gradient vanishing and gradient explosion in deep neural networks through residual learning, and improves the accuracy and generalization ability of the network. The ResNet-152 model has achieved good results in the fields of image classification, object detection and semantic segmentation. For the bird classification dataset BIRDS 525 SPECIES-IMAGE CLASSIFICATION, the ResNet-152 model was used for classification, and excellent results were obtained. The classification accuracy reached 96.5%, the accuracy reached 98.0%, the recall rate was 94.6%, the f1 score was 94.7%, and the AUC reached 95.6%. These indicators indicate that the ResNet-152 model has high classification accuracy and generalization ability for bird classification datasets. At the same time, it also shows that the ResNet-152 model has a good application prospect in processing large-scale image classification tasks.
Keywords
Image classification, ResNet-152, AUC
[1]. Thien-Nu H ,Daehee K . Supervised contrastive ResNet and transfer learning for the in-vehicle intrusion detection system[J]. Expert Systems With Applications,2024,238(PE).
[2]. Serena S ,Ashish S ,Sreeram V P , et al. A refined ResNet18 architecture with Swish activation function for Diabetic Retinopathy classification[J]. Biomedical Signal Processing and Control,2024,88(PA).
[3]. Enrico C ,Alessio S ,Matteo L , et al. A Robust Initialization of Residual Blocks for Effective ResNet Training Without Batch Normalization.[J]. IEEE transactions on neural networks and learning systems,2023,PP.
[4]. Enrico C ,Alessio S ,Matteo L , et al. A Robust Initialization of Residual Blocks for Effective ResNet Training Without Batch Normalization.[J]. IEEE transactions on neural networks and learning systems,2023,PP.
[5]. A. V ,S. S . Resnet-Unet-FSOA based cranial nerve segmentation and medial axis extraction using MRI images[J]. The Imaging Science Journal,2023,71(8).
[6]. Esraa H ,Shamim M H ,Abeer S , et al. A quantum convolutional network and ResNet (50)-based classification architecture for the MNIST medical dataset[J]. Biomedical Signal Processing and Control,2024,87(PB).
[7]. Wiku L K ,Pingan W ,Hyun-Ho N , et al. Airborne hyperspectral imaging for early diagnosis of kimchi cabbage downy mildew using 3D-ResNet and leaf segmentation[J]. Computers and Electronics in Agriculture,2023,214.
[8]. Tiejun C ,SungJune B . Library-Based Raman Spectral Identification Using Multi-Input Hybrid ResNet.[J]. ACS omega,2023,8(40).
[9]. Li X ,Xu X ,He X , et al. Intelligent Crack Detection Method Based on GM-ResNet[J]. Sensors,2023,23(20).
[10]. Asel S ,Mo K ,Andrii K , et al. Hybrid quantum ResNet for car classification and its hyperparameter optimization[J]. Quantum Machine Intelligence,2023,5(2).
Cite this article
Song,H. (2024). Bird image classification based on improved ResNet-152 image classification model. Applied and Computational Engineering,54,206-212.
Data availability
The datasets used and/or analyzed during the current study will be available from the authors upon reasonable request.
Disclaimer/Publisher's Note
The statements, opinions and data contained in all publications are solely those of the individual author(s) and contributor(s) and not of EWA Publishing and/or the editor(s). EWA Publishing and/or the editor(s) disclaim responsibility for any injury to people or property resulting from any ideas, methods, instructions or products referred to in the content.
About volume
Volume title: Proceedings of the 4th International Conference on Signal Processing and Machine Learning
© 2024 by the author(s). Licensee EWA Publishing, Oxford, UK. This article is an open access article distributed under the terms and
conditions of the Creative Commons Attribution (CC BY) license. Authors who
publish this series agree to the following terms:
1. Authors retain copyright and grant the series right of first publication with the work simultaneously licensed under a Creative Commons
Attribution License that allows others to share the work with an acknowledgment of the work's authorship and initial publication in this
series.
2. Authors are able to enter into separate, additional contractual arrangements for the non-exclusive distribution of the series's published
version of the work (e.g., post it to an institutional repository or publish it in a book), with an acknowledgment of its initial
publication in this series.
3. Authors are permitted and encouraged to post their work online (e.g., in institutional repositories or on their website) prior to and
during the submission process, as it can lead to productive exchanges, as well as earlier and greater citation of published work (See
Open access policy for details).