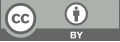
Severity’s prediction of car accidents in PA and model comparison
- 1 Lehigh University
- 2 University of California San Diego
* Author to whom correspondence should be addressed.
Abstract
This study aims to provide an analytical and predictive framework for understanding and forecasting the probabilities of varying severities of traffic accidents in Pennsylvania, based on the US Accident dataset dated up to March 23. Initially, the dataset and variable descriptions are provided for comprehensive understanding. During the data preprocessing phase, variables undergo a thorough examination for missing values, and suitable imputation methods are selected for data completeness. Further, feature selection and data cleansing are executed to prepare the dataset for model training. The study predominantly utilizes three machine learning algorithms, Logistic Regression, Random Forest, and Support Vector Machine, to construct predictive models. The performance of these models is meticulously evaluated for accuracy and compared through specific data sampling techniques. Overfitting checks, feature importance elucidation, and in-depth discussions on model performance variations are also included. By navigating through this multifaceted analysis, the study aims to shed light on the strengths and weaknesses of different modeling approaches for this particular problem context, thereby providing valuable insights for future research endeavors.
Keywords
Traffic accidents, Machine learning, Severity prediction, Feature selection, Pennsylvania
[1]. Mair, Carolyn, et al. "An investigation of machine learning based prediction systems." Journal of systems and software 53.1 (2000): 23-29.
[2]. Pradhan, Biswajeet, et al. "Modeling traffic accident severity using neural networks and support vector machines." Laser Scanning Systems in Highway and Safety Assessment: Analysis of Highway Geometry and Safety Using LiDAR (2020): 111-117.
[3]. Strobl, Carolin, James Malley, and Gerhard Tutz. "An introduction to recursive partitioning: rationale, application, and characteristics of classification and regression trees, bagging, and random forests." Psychological methods 14.4 (2009): 323.
[4]. Dhepe, Y. (2023). Project-4 US Accidents Data EDA. GitHub. Retrieved September 20, 2023, from https://www.kaggle.com/code/yuvrajdhepe/project-4-us-accidents-data-eda/notebook
[5]. Moosavi, S. (2023). US Accidents (2016 - 2023): A Countrywide Traffic Accident Dataset (2016 - 2023). Kaggle. Retrieved from https://www.kaggle.com/datasets/sobhanmoosavi/us-accidents
[6]. Oke, J., W. B. Akinkunmi, and S. O. Etebefia. "Use of correlation, tolerance and variance inflation factor for multicollinearity test." GSJ 7.5 (2019).
[7]. Kim, Tae Kyun. "Understanding one-way ANOVA using conceptual figures." Korean journal of anesthesiology 70.1 (2017): 22-26.
[8]. Seni, Giovanni, and John Elder. Ensemble methods in data mining: improving accuracy through combining predictions. Morgan & Claypool Publishers, 2010.
[9]. Moosavi, S., Samavatian, M. H., Parthasarathy, S., & Ramnath, R. (2019). A Countrywide Traffic Accident Dataset. arXiv preprint arXiv:1906.05409. Retrieved September 20, 2023, from https://doi.org/10.48550/arXiv.1906.05409
[10]. Ebrahimi-Khusfi, Zohre, Ali Reza Nafarzadegan, and Fatemeh Dargahian. "Predicting the number of dusty days around the desert wetlands in southeastern Iran using feature selection and machine learning techniques." Ecological Indicators 125 (2021): 107499.
Cite this article
Chu,W.;Qu,X. (2024). Severity’s prediction of car accidents in PA and model comparison. Applied and Computational Engineering,52,215-226.
Data availability
The datasets used and/or analyzed during the current study will be available from the authors upon reasonable request.
Disclaimer/Publisher's Note
The statements, opinions and data contained in all publications are solely those of the individual author(s) and contributor(s) and not of EWA Publishing and/or the editor(s). EWA Publishing and/or the editor(s) disclaim responsibility for any injury to people or property resulting from any ideas, methods, instructions or products referred to in the content.
About volume
Volume title: Proceedings of the 4th International Conference on Signal Processing and Machine Learning
© 2024 by the author(s). Licensee EWA Publishing, Oxford, UK. This article is an open access article distributed under the terms and
conditions of the Creative Commons Attribution (CC BY) license. Authors who
publish this series agree to the following terms:
1. Authors retain copyright and grant the series right of first publication with the work simultaneously licensed under a Creative Commons
Attribution License that allows others to share the work with an acknowledgment of the work's authorship and initial publication in this
series.
2. Authors are able to enter into separate, additional contractual arrangements for the non-exclusive distribution of the series's published
version of the work (e.g., post it to an institutional repository or publish it in a book), with an acknowledgment of its initial
publication in this series.
3. Authors are permitted and encouraged to post their work online (e.g., in institutional repositories or on their website) prior to and
during the submission process, as it can lead to productive exchanges, as well as earlier and greater citation of published work (See
Open access policy for details).