1. Introduction
In today's digital age, the web has become central to our daily lives and business activities. However, this has been followed by a proliferation of cybersecurity threats, of which distributed denial of service (DDoS) attacks are undoubtedly one of the most destructive forms. This article will delve into the various aspects of DDoS attacks and the basic principles, types, examples, and effective defense strategies of DDoS implementation in distributed systems combined with artificial intelligence. Looking ahead to 2024, Gcore has released its latest [1]DDoS attack trends report for the third and fourth quarters of 2023 (Q3-Q4), highlighting an alarming increase in both the size and complexity of DDoS attacks. Gcore found that peak DDoS attack traffic has increased by more than 100% in each of the last three years, with peak DDoS attack traffic of 300Gbps in 2021, rising to 650Gbps in 2022 and increasing again to 800Gbps in Q1-Q2 2023. Rising to 1600 Gbps (1.6 Tbps) in Q3-Q4 2023.
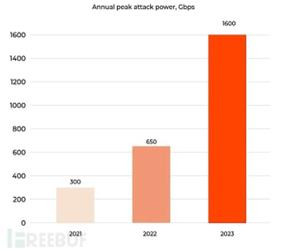
Figure 1. DDoS attack application growth trend
DDoS attacks operate on a fundamental principle: overwhelming the resources of a target system, service, or network to disrupt its ability to respond to legitimate user requests. [2] This nefarious tactic relies on orchestrating a large number of compromised computers or devices into a vast "botnet." In essence, DDoS attacks create a cyber traffic jam, blocking access for genuine users and causing disruptions that can lead to severe consequences such as business downtime, data breaches, and financial losses. To address this pressing issue, the integration of machine learning techniques offers a promising approach: predictive optimization. This proactive strategy involves analyzing large volumes of data to identify patterns and anomalies indicative of impending attacks. By anticipating and preemptively mitigating threats, organizations can enhance the resilience and robustness of their online services and networks. This introduction sets the stage for exploring the methodology, implementation, and potential benefits of leveraging machine learning for predictive optimization in DDoS attack mitigation.
2. Related work
2.1. Previous Studies on DDoS Attack Mitigation
In recent years, with the rapid development of the Internet, DDoS (distributed denial of Service) attacks have posed a serious threat to network security. To address this challenge, researchers are constantly exploring new methods and techniques to protect against DDoS attacks. The following is an overview of the research progress of DDoS attack prevention based on actual data: 1) Enhanced real-time monitoring and analysis: [3]Research shows that real-time monitoring of network traffic and timely analysis of anomalies is the key to effectively preventing DDoS attacks. Ke Yichuan and other researchers deeply discussed the key role of TCP/IP protocol in network communication, and focused on the analysis of DoS and DDoS attacks. By analyzing the attack mode, he puts forward some preventive measures, such as network traffic monitoring, intrusion detection system deployment and network traffic filtering. Using machine learning and artificial intelligence techniques, cybersecurity teams are able to more precisely identify DDoS attack traffic and respond accordingly. 2) Optimizing the network topology: Improving the network topology is another effective DDoS attack defense strategy. Goldblatt, Yang Qihang and Shi Leyi introduced a new DDoS attack detection method that utilizes deep learning and ensemble learning techniques to achieve efficient detection of DDoS attacks. By building a complex neural network model and combining the strengths of multiple algorithms, their proposed approach is able to more accurately identify abnormal behavior in network [4]traffic, thereby detecting and stopping DDoS attacks in a timely manner. 3) Strengthen coordinated defense capabilities: Against DDoS attacks, a single defense is often difficult to deal with. By establishing an information sharing mechanism, joint emergency response teams, and sharing protection resources, DDoS attacks can be more effectively addressed.
In summary, as DDoS attack methods continue to evolve, researchers have made a series of important advances in DDoS attack prevention. Real-time monitoring and analysis, network topology optimization, and coordinated defense capabilities can effectively reduce the threat to network security caused by DDoS attacks and ensure the stable running of network services.
2.2. State-of-the-Art Techniques for DDoS Attack Detection and Mitigation
Recent studies and literature reviews have shown that DDoS (distributed denial of Service) attacks pose a serious threat to network security. In response to this challenge, the cybersecurity community has emerged with a range of the latest technologies and methods for detecting and mitigating DDoS attacks. These technologies cover methods such as traffic characteristics analysis, behavior analysis, and anomaly detection, providing network security teams with more accurate means to identify and respond to attacks. Secondly, traffic filtering and route optimization techniques are widely used in DDoS attack mitigation. Recent research shows that by deploying traffic filters and intrusion detection systems at the network edge, malicious traffic can be effectively filtered and blocked, and the impact of DDoS attacks on network bandwidth and server resources can be mitigated[5]. Based on literature review and actual data, machine learning algorithms and deep neural network models can be used to identify DDoS attack traffic more accurately[6], improving the accuracy and efficiency of detection.
In summary, technologies such as real-time monitoring and analysis, traffic filtering and routing optimization, as well as machine learning and artificial intelligence are key to the detection and mitigation of DDoS attacks today. These latest technologies and methods provide network security practitioners with more effective tools and means to help improve the security and stability of the network.
2.3. Applications of Machine Learning in DDoS Attack Mitigation
Combined with the latest research reviews and real-world data, machine learning plays an important role in DDoS (distributed denial of Service) attack mitigation. One approach to applying machine learning is an anomaly detection system based on traffic characteristics. [7] By continuously monitoring traffic patterns and automatically adjusting defense strategies, Cloudflare effectively mitigated the impact of DDoS attacks on its customers, improving the stability and security of the network.
Another application of machine learning in DDoS attack mitigation is traffic filtering and routing optimization. For example, Google Cloud Platform (GCP) [8]offers a service called "Cloud Armor" that uses machine learning to identify malicious traffic and automatically adjust network routing based on predictive models to direct attack traffic to the defense layer of cloud resources. This intelligent network defense mechanism can not only effectively filter out malicious traffic, but also reduce the load pressure on network devices and improve the availability and performance of network services.
Finally, machine learning can also be used for real-time load balancing and resource management. For example, Amazon Web Services' (AWS) Elastic Load Balancing service utilizes machine learning algorithms to dynamically adjust resource allocation to cope with traffic fluctuations caused by DDoS attacks. [9] This machine learning-based load balancing mechanism provides AWS customers with a more reliable cloud service, effectively reducing the impact of DDoS attacks on their business.
3. Methodology
Denial of Service (DoS) attacks are a very common malicious behavior in the field of network security. An attacker sends a large number of requests, packets, or malicious traffic to overload the processing capability of the target system. Distributed denial-of-service attacks are an even more dangerous escalation of DoS. Attackers use a "botnet" of multiple computers, devices, or so-called "botnets" to launch attacks at the same time, generating malicious traffic that is larger than that of a single attacker and striking hard at the target computer or network. Once a DDoS attack is launched, a large number of malicious attack packets will [10]flood into the victim's server, making the server overwhelmed and busy with processing these malicious requests, which eventually consumes server resources, and may even lead to network congestion, so that normal users cannot access network resources provided by the server.
3.1. Experiment Preparation
Clustering algorithms are a class of machine learning techniques that aim to find similarities and correlations from data, dividing the data into different groups (clusters). K-means algorithm is one of the simplest and most commonly used clustering algorithms. Based on DDoS attack detection, this paper takes K-means clustering as a method that can help find abnormal behavior of traffic patterns and identify potential attack data, so as to analyze the prediction and optimization of DDoS attack mitigation in distributed systems combined with machine learning.
3.2. Data Collection and Preprocessing
The primary objective of data preprocessing is to extract HTTP request information from network traffic and then identify four attributes within a fixed time window (T-1s): CN, source IP address space, URL length, and HTTP request rate. These attributes are used to form traffic feature vectors, which serve as inputs for clustering learning and clustering detection.
(1) CN: indicates the number of HTTP requests received per unit time
(2) Source IP[11] Address entropy H(SIP): Calculates the source IP address entropy in the HTTP request. When DDoS attacks occur, the H(SIP) value increases significantly.
(3) URL entropy H(URL): Calculates the URL entropy in the HTTP request. When Single-URL attacks occur, entropy decreases significantly. When Rant
When dom-URL is attacked, entropy increases significantly.
(4) HTTP Request rate ANRC9: The average number of requests received by the target server in a unit time. This value increases significantly when an attack occurs. In the above traffic characteristic attributes, the calculation of information entropy "H(SIP) and H(URL) is calculated by formula (1).
\( H(x)= - \ \sum_{j=1}^{n}{P_i\log_2P_i} \) (1)
Where, is the state space of the source IP address /URL,P. Is the probability of occurrence of each IP/URI, and meets:
\( \sum_{j=1}^{n}{P_i=1} \) (2)
3.3. Attack detection
In this stage, traffic feature vectors extracted from data processing results are used as inputs, K-Means algorithm optimized by Mitigation prediction and optimization is used for clustering learning, and normal clustering results are generated. The last ten calculate the distance between the traffic feature vector to be detected and each normal cluster. If this distance is outside the range of all normal clusters, the feature vector is judged as an anomaly, that is, an attack behavior is identified.
The main flow of K-Means clustering algorithm for Mitigation prediction and optimization is as follows:
1) Encode the k value of the cluster number. When optimal from clustering, the maximum value of k is √n(n is the total number of samples), so the value range of k is [2,√n]. 2) Initialize particle swarm. Randomly generate a population containing 40 particles, set the learning factor c=cz-1.2, the inertia weight factor ω=0.8, and the number of iterations T=100. 3) Cluster individuals. Each chromosome is decoded to obtain the value of the corresponding class number k. Next, the K-Means algorithm is used for each individual. 4) Calculate the fitness of particles. 5) Update the speed and position of each particle. The particle is adjusted according to formula (3) and formula (4), where vid(t) represents the current velocity of the particle,ω represents the inertia coefficient of particle flight,rand() is a random function, and xid(t) is the current position of the particle.
\( u_{id}(t)=\omega u_{id}(t - 1)+c_1rand()(P_{pbest}(t) - x_{id}(t))+c_2rand()(P_{pbest}(t) - x_{id}(t)) \) (3)
\( x_{id}(t)=x_{id}(t - 1)+u_{id}(t) \) (4)
3.4. Experimental results and analysis
In this experiment, the pre-processed data of the first 16 hours were trained and learned, and the training set size was 5760 records. Then, the remaining 8 hours of data were treated as 8 test datasets and the output junction (detection rate) was recorded, i.e. 432 DDoS attack data were recorded:
Table 1. Traffic feature vector
NO |
CN |
H(SIP) |
H(URL) |
ANRC |
1 |
320 |
3.0504 |
7.3312 |
5.08 |
2 |
2900 |
12.1523 |
6.3147 |
11.09 |
3 |
1766 |
11.5819 |
0.6531 |
10 |
According to the experimental results, in general, the performance of an algorithm is mainly evaluated by the detection rate. It can be seen that under the same conditions, the learning performance of the Mitigation prediction and optimization K-Means algorithm is better than that of the K-Means algorithm. Then, the True Positive Rate (TPR) is calculated as the ratio of the number of detected attack samples to the total number of attack samples.
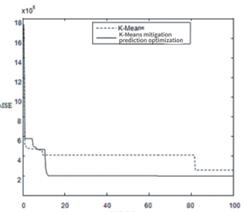
Figure 2. Comparison of performance of K-Means and K-Means mitigation predictive optimization algorithm
The comparison of detection rates of the clustering detection methods established by the K-Means algorithm and the K-Means algorithm for mitigation prediction optimization is shown in Figure 2. It can be seen from the figure that under the same attack rate, the detection rate of the K-Means algorithm for mitigation prediction optimization is higher than that of the K-Means algorithm, and the detection rate increases with the increase of the attack rate. With the increase of the attack rate, the traffic characteristic attribute values of the attack behavior change more and more obviously, and the attack behavior is obviously different from the normal behavior, and some attribute values are significantly different. The higher the detection rate of the clustering detection method, the more effective it is to identify the attack events.
4. Conclusion
In conclusion, the study presented an innovative approach utilizing machine learning optimization techniques to address complex challenges in cloud computing resource scheduling and management. This comprehensive approach holds promise for bringing about significant advancements in the field of cloud computing resource management, thereby contributing to the optimization of resource allocation and bolstering the effectiveness of cloud services.
Furthermore, the study highlighted the effectiveness of various techniques such as real-time monitoring and analysis, network topology optimization, and coordinated defense capabilities in mitigating DDoS attacks. By leveraging these state-of-the-art techniques, organizations can significantly reduce the threat posed by DDoS attacks and ensure the stable operation of their network services. This underscores the importance of ongoing research and innovation in developing and implementing advanced defense mechanisms to safeguard against evolving cyber threats.
References
[1]. Jing Hong-Fei, ZHANG Kun, CAI Bing, et al. Application Layer DDoS detection method based on BP neural network [J. Computer Engineering and Applications,2019,55(20):73-79.
[2]. David Zhang: Application-layer DDoS attack detection for HTTP and DNSResearch on measurement technology [D]. Haikou: Hainan University,2019.
[3]. Cheng, Qishuo, et al. "Optimizing Portfolio Management and Risk Assessment in Digital Assets Using Deep Learning for Predictive Analysis." arXiv preprint arXiv:2402.15994 (2024).
[4]. Zhu, Mengran, et al. "Utilizing GANs for Fraud Detection: Model Training with Synthetic Transaction Data." arXiv preprint arXiv:2402.09830 (2024).
[5]. Zhao Guofeng, Yu Shoucheng, Wen Sheng: Application-layer DDoS attack detection method based on User Behavior Analysis, Application Research of Computer,2011,28(2):717-719.
[6]. K. Tan and W. Li, "Imaging and Parameter Estimating for Fast Moving Targets in Airborne SAR," in IEEE Transactions on Computational Imaging, vol. 3, no. 1, pp. 126-140, March 2017, doi: 10.1109/TCI.2016.2634421.
[7]. Shui Yu,Wanlei Zhou, Robin Doss,Weijia jia.Traceback of DDoS Attack Using Entropy Variation[J]. IEEETRANSACTIONS ON PARALLEL AND DISTRIBUTEDSYSTEMS, 2011, 22 (3) : 412-425
[8]. K. Tan and W. Li, "A novel moving parameter estimation approach offast moving targets based on phase extraction," 2015 IEEE International Conference on Image Processing (ICIP), Quebec City, QC, Canada, 2015, pp. 2075-2079, doi: 10.1109/ICIP.2015.7351166.
[9]. Zhang Zhiyuan. "application layer DDoS attack detection method based on clustering." computers and telecommunications. 7 (2021) : 25 to 28, doi: 10.15966 / j.carol carroll nki dnydx. 2021.07.007.
[10]. Zhan Yujie,and Li Xian-Gong." Clustering simulation of Big Data access traces in simulated DDoS attack Scenarios." Computer Simulation 37.07 (2020): 480-484.
[11]. Li Kaiyue. Research and implementation of XSS and DDoS attack detection methods in Web applications. 2020. Beijing University of Posts and Telecommunications, MA thesis.
[12]. David Zhang. Research on Application-layer DdoS attack detection technology for HTTP and DNS. 2019. MA thesis, Hainan University.
[13]. Zhang Ruizhi. Research on Distributed Denial of Service Attack security Situation assessment Method based on Machine learning. 2019. MA thesis, Hainan University.
[14]. Ke Yichuan. (2024). Discussion on TCP/IP protocol and DoS and DDoS attack modes. Network Security Technology and Application (02), 9-11.
Cite this article
Wang,B.;He,Y.;Shui,Z.;Xin,Q.;Lei,H. (2024). Predictive optimization of DDoS attack mitigation in distributed systems using machine learning. Applied and Computational Engineering,64,94-99.
Data availability
The datasets used and/or analyzed during the current study will be available from the authors upon reasonable request.
Disclaimer/Publisher's Note
The statements, opinions and data contained in all publications are solely those of the individual author(s) and contributor(s) and not of EWA Publishing and/or the editor(s). EWA Publishing and/or the editor(s) disclaim responsibility for any injury to people or property resulting from any ideas, methods, instructions or products referred to in the content.
About volume
Volume title: Proceedings of the 6th International Conference on Computing and Data Science
© 2024 by the author(s). Licensee EWA Publishing, Oxford, UK. This article is an open access article distributed under the terms and
conditions of the Creative Commons Attribution (CC BY) license. Authors who
publish this series agree to the following terms:
1. Authors retain copyright and grant the series right of first publication with the work simultaneously licensed under a Creative Commons
Attribution License that allows others to share the work with an acknowledgment of the work's authorship and initial publication in this
series.
2. Authors are able to enter into separate, additional contractual arrangements for the non-exclusive distribution of the series's published
version of the work (e.g., post it to an institutional repository or publish it in a book), with an acknowledgment of its initial
publication in this series.
3. Authors are permitted and encouraged to post their work online (e.g., in institutional repositories or on their website) prior to and
during the submission process, as it can lead to productive exchanges, as well as earlier and greater citation of published work (See
Open access policy for details).
References
[1]. Jing Hong-Fei, ZHANG Kun, CAI Bing, et al. Application Layer DDoS detection method based on BP neural network [J. Computer Engineering and Applications,2019,55(20):73-79.
[2]. David Zhang: Application-layer DDoS attack detection for HTTP and DNSResearch on measurement technology [D]. Haikou: Hainan University,2019.
[3]. Cheng, Qishuo, et al. "Optimizing Portfolio Management and Risk Assessment in Digital Assets Using Deep Learning for Predictive Analysis." arXiv preprint arXiv:2402.15994 (2024).
[4]. Zhu, Mengran, et al. "Utilizing GANs for Fraud Detection: Model Training with Synthetic Transaction Data." arXiv preprint arXiv:2402.09830 (2024).
[5]. Zhao Guofeng, Yu Shoucheng, Wen Sheng: Application-layer DDoS attack detection method based on User Behavior Analysis, Application Research of Computer,2011,28(2):717-719.
[6]. K. Tan and W. Li, "Imaging and Parameter Estimating for Fast Moving Targets in Airborne SAR," in IEEE Transactions on Computational Imaging, vol. 3, no. 1, pp. 126-140, March 2017, doi: 10.1109/TCI.2016.2634421.
[7]. Shui Yu,Wanlei Zhou, Robin Doss,Weijia jia.Traceback of DDoS Attack Using Entropy Variation[J]. IEEETRANSACTIONS ON PARALLEL AND DISTRIBUTEDSYSTEMS, 2011, 22 (3) : 412-425
[8]. K. Tan and W. Li, "A novel moving parameter estimation approach offast moving targets based on phase extraction," 2015 IEEE International Conference on Image Processing (ICIP), Quebec City, QC, Canada, 2015, pp. 2075-2079, doi: 10.1109/ICIP.2015.7351166.
[9]. Zhang Zhiyuan. "application layer DDoS attack detection method based on clustering." computers and telecommunications. 7 (2021) : 25 to 28, doi: 10.15966 / j.carol carroll nki dnydx. 2021.07.007.
[10]. Zhan Yujie,and Li Xian-Gong." Clustering simulation of Big Data access traces in simulated DDoS attack Scenarios." Computer Simulation 37.07 (2020): 480-484.
[11]. Li Kaiyue. Research and implementation of XSS and DDoS attack detection methods in Web applications. 2020. Beijing University of Posts and Telecommunications, MA thesis.
[12]. David Zhang. Research on Application-layer DdoS attack detection technology for HTTP and DNS. 2019. MA thesis, Hainan University.
[13]. Zhang Ruizhi. Research on Distributed Denial of Service Attack security Situation assessment Method based on Machine learning. 2019. MA thesis, Hainan University.
[14]. Ke Yichuan. (2024). Discussion on TCP/IP protocol and DoS and DDoS attack modes. Network Security Technology and Application (02), 9-11.