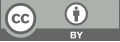
Intelligent classification and personalized recommendation of E-commerce products based on machine learning
- 1 Computer Science and Engineering , Santa Clara University
- 2 Computer Science,Northeastern University
- 3 Electrical & Computer Engineering,New York University
- 4 Computer Information System, Colorado state university
- 5 Management Information Systems, University of Pittsburgh
* Author to whom correspondence should be addressed.
Abstract
With the rapid evolution of the Internet and the exponential proliferation of information, users encounter information overload and the conundrum of choice. Personalized recommendation systems play a pivotal role in alleviating this burden by aiding users in filtering and selecting information tailored to their preferences and requirements. This paper undertakes a comparative analysis between the operational mechanisms of traditional e-commerce commodity classification systems and personalized recommendation systems. It delineates the significance and application of personalized recommendation systems across e-commerce, content information, and media domains. Furthermore, it delves into the challenges confronting personalized recommendation systems in e-commerce, including data privacy, algorithmic bias, scalability, and the cold start problem. Strategies to address these challenges are elucidated. Subsequently, the paper outlines a personalized recommendation system leveraging the BERT model and nearest neighbor algorithm, specifically tailored to address the exigencies of the eBay e-commerce platform. The efficacy of this recommendation system is substantiated through manual evaluation, and a practical application operational guide and structured output recommendation results are furnished to ensure the system's operability and scalability.
Keywords
personalized recommendation system, E-commerce, data privacy, BERT model
[1]. Zhang, Yuchen, et al. "Taxonomy discovery for personalized recommendation." Proceedings of the 7th ACM international conference on Web search and data mining. 2014.
[2]. Tian, Yonghong, et al. "College library personalized recommendation system based on hybrid recommendation algorithm." procedia cirp 83 (2019): 490-494.
[3]. Song, Xiaodan, et al. "Personalized recommendation driven by information flow." Proceedings of the 29th annual international ACM SIGIR conference on Research and development in information retrieval. 2006.
[4]. Cheng, Qishuo, et al. "Optimizing Portfolio Management and Risk Assessment in Digital Assets Using Deep Learning for Predictive Analysis." arXiv preprint arXiv:2402.15994 (2024).
[5]. Wu, Jiang, et al. "Data Pipeline Training: Integrating AutoML to Optimize the Data Flow of Machine Learning Models." arXiv preprint arXiv:2402.12916 (2024).
[6]. Shepitsen, Andriy, et al. "Personalized recommendation in social tagging systems using hierarchical clustering." Proceedings of the 2008 ACM conference on Recommender systems. 2008.
[7]. Zhou, Y., Tan, K., Shen, X., & He, Z. (2024). A Protein Structure Prediction Approach Leveraging Transformer and CNN Integration. arXiv preprint arXiv:2402.19095.
[8]. Qian, Xueming, et al. "Personalized recommendation combining user interest and social circle." IEEE transactions on knowledge and data engineering 26.7 (2013): 1763-1777.
[9]. Zhang, Denghui, et al. "E-BERT: A phrase and product knowledge enhanced language model for e-commerce." arXiv preprint arXiv:2009.02835 (2020).
[10]. Wang, Yong, et al. "Construction and application of artificial intelligence crowdsourcing map based on multi-track GPS data." arXiv preprint arXiv:2402.15796 (2024).
[11]. Ma, Haowei. "Automatic positioning system of medical service robot based on binocular vision." 2021 3rd International Symposium on Robotics & Intelligent Manufacturing Technology (ISRIMT). IEEE, 2021.
[12]. Zheng, Jiajian, et al. "The Random Forest Model for Analyzing and Forecasting the US Stock Market in the Context of Smart Finance." arXiv preprint arXiv:2402.17194 (2024).
[13]. Yang, Le, et al. "AI-Driven Anonymization: Protecting Personal Data Privacy While Leveraging Machine Learning." arXiv preprint arXiv:2402.17191 (2024).
[14]. Zuo, Yi, et al. "Personalized recommendation based on evolutionary multi-objective optimization [research frontier]." IEEE Computational Intelligence Magazine 10.1 (2015): 52-62.
[15]. Lei, Yaguo, Zhengjia He, and Yanyang Zi. "Application of an intelligent classification method to mechanical fault diagnosis." Expert Systems with Applications 36.6 (2009): 9941-9948.
[16]. Liu, Na, et al. "A novel intelligent classification model for breast cancer diagnosis." Information Processing & Management 56.3 (2019): 609-623.
[17]. Yacin Sikkandar, Mohamed, et al. "Deep learning based an automated skin lesion segmentation and intelligent classification model." Journal of ambient intelligence and humanized computing 12 (2021): 3245-3255.
[18]. Chavez-Badiola, Alejandro, et al. "Embryo Ranking Intelligent Classification Algorithm (ERICA): artificial intelligence clinical assistant predicting embryo ploidy and implantation." Reproductive biomedicine online 41.4 (2020): 585-593.
[19]. Haowei, M., Ebrahimi, S., Mansouri, S., Abdullaev, S. S., Alsaab, H. O., & Hassan, Z. F. (2023). CRISPR/Cas-based nanobiosensors: A reinforced approach for specific and sensitive recognition of mycotoxins. Food Bioscience, 56, 103110.
Cite this article
Xu,K.;Zhou,H.;Zheng,H.;Zhu,M.;Xin,Q. (2024). Intelligent classification and personalized recommendation of E-commerce products based on machine learning. Applied and Computational Engineering,64,147-153.
Data availability
The datasets used and/or analyzed during the current study will be available from the authors upon reasonable request.
Disclaimer/Publisher's Note
The statements, opinions and data contained in all publications are solely those of the individual author(s) and contributor(s) and not of EWA Publishing and/or the editor(s). EWA Publishing and/or the editor(s) disclaim responsibility for any injury to people or property resulting from any ideas, methods, instructions or products referred to in the content.
About volume
Volume title: Proceedings of the 6th International Conference on Computing and Data Science
© 2024 by the author(s). Licensee EWA Publishing, Oxford, UK. This article is an open access article distributed under the terms and
conditions of the Creative Commons Attribution (CC BY) license. Authors who
publish this series agree to the following terms:
1. Authors retain copyright and grant the series right of first publication with the work simultaneously licensed under a Creative Commons
Attribution License that allows others to share the work with an acknowledgment of the work's authorship and initial publication in this
series.
2. Authors are able to enter into separate, additional contractual arrangements for the non-exclusive distribution of the series's published
version of the work (e.g., post it to an institutional repository or publish it in a book), with an acknowledgment of its initial
publication in this series.
3. Authors are permitted and encouraged to post their work online (e.g., in institutional repositories or on their website) prior to and
during the submission process, as it can lead to productive exchanges, as well as earlier and greater citation of published work (See
Open access policy for details).