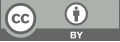
Predicting drug-drug interactions using heterogeneous graph neural networks: HGNN-DDI
- 1 Northeastern University
- 2 University College Dublin
- 3 McGill University
* Author to whom correspondence should be addressed.
Abstract
This research centers on predicting drug-drug interactions (DDIs) using a novel approach involving graph neural networks (GNNs) with integrated attention mechanisms. In this method, drugs and proteins are depicted as nodes within a heterogeneous graph. This graph is characterized by different types of edges symbolizing not only DDIs but also drug-protein interactions (DPIs) and protein-protein interactions (PPIs). To analyze the chemical structures of drugs, we employ a pretrained model named ChemBERTa, which utilizes simplified molecular input line entry system (SMILES) strings. The similarity between drug structures based on their SMILES strings is determined using the RDkit tool. Our model is designed to establish and link heterogeneous graph neural networks, taking into account the DPIs and PPIs as key input data. For the final prediction of interaction types between various drugs, we use the Multi-Layer Perception (MLP) technique. This model's primary objective is to enhance the accuracy of DDI predictions by factoring in additional data on both drug-protein and protein-protein interactions. The forecasted DDIs are presented with associated probabilities, offering valuable insights to healthcare professionals. These insights are crucial for assessing the potential risks and advantages of combining different drugs, particularly for patients with diseases at different stages of progression.
Keywords
Graph Neural Network, Drug-drug Interaction, Machine Learning, Heterogeneous Graph Learning
[1]. W. Hu, W. Zhang, Y. Zhou, Y. Luo, X. Sun, H. Xu, S. Shi, T. Li, Y. Xu, Q. Yang, Y. Qiu, F. Zhu, H. Dai, Mecddi: Clarified drug–drug interaction mechanism facilitating rational drug use and potential drug–drug interaction pre- diction, Journal of Chemical Information and Modeling 63 (5) (2023) 1626–1636. doi:10.1021/acs.jcim. 2c01656.
[2]. I. Cascorbi, Drug interactions—principles, examples and clinical consequences, Deutsches A¨ rzteblatt International109 (2012) 546–556. doi:10.3238/arztebl.2012.0546.
[3]. L. A. Kondili, G. B. Gaeta, D. Ieluzzi, A. L. Zignego, M. Monti, A. Gori, A. Soria, G. Raimondo, R. Filomia, A. Di Leo, et al, Real-life data on potential drug-drug interactions in patients with chronic hepatitis c viral infection undergoing antiviral therapy with interferon-free daas in the piter cohort study, PloS One 12 (2) (2017). doi: 10.1371/journal.pone.0172159.
[4]. J. Niu, R. M. Straubinger, D. E. Mager, Pharmacodynamic drug–drug interactions, Clinical Pharmacology and Therapeutics 105 (6) (2019) 1395–1406. doi:10.1002/cpt.1434.
[5]. J. E. Zwart-van Rijkom, E. V. Uijtendaal, M. J. Ten Berg, W. W. Van Solinge, A. C. Egberts, Frequency and nature of drug–drug interactions in a dutch university hospital, British journal of clinical pharmacology 68 (2) (2009) 187–193.
[6]. V. Gligorijevic´, P. D. Renfrew, T. Kosciolek, J. K. Leman, D. Berenberg, T. Vatanen, C. Chandler, B. C. Tay- lor, I. M. Fisk, H. Vlamakis, R. J. Xavier, R. Knight, K. Cho, R. Bonneau, Structure-based protein function prediction using graph convolutional networks, Nature communications 12 (1) (2021) 3168. doi:10.1038/ s41467-021-23303-9.
[7]. X. Lin, Z. Quan, Z.-J. Wang, T. Ma, X. Zeng, Kgnn: Knowledge graph neural network for drug-drug interaction prediction, Proceedings of the Twenty-Ninth International Joint Conference on Artificial Intelligence (2020). doi: 10.24963/ijcai.2020/380.
[8]. Y. Chen, T. Ma, X. Yang, J. Wang, B. Song, X. Zeng, Muffin: multi-scale feature fusion for drug–drug interaction prediction, Bioinformatics 37 (17) (2021) 2651–2658. doi:10.1093/bioinformatics/btab169.
[9]. H. He, G. Chen, C. Yu-Chian Chen, 3dgt-ddi: 3d graph and text based neural network for drug-drug interaction prediction, Briefings in bioinformatics 23 (3) (2022). doi:10.1093/bib/bbac134.
[10]. J. Y. Ryu, H. U. Kim, S. Y. Lee, Deep learning improves prediction of drug–drug and drug–food interactions, Proceedings of the National Academy of Sciences 115 (18) (2018). doi:10.1073/pnas.1803294115.
[11]. M. Herrero-Zazo, I. Segura-Bedmar, P. Mart´ınez, R. Declerck, The ddi corpus: An annotated corpus with phar- macological substances and drug–drug interactions, Journal of Biomedical Informatics 46 (5) (2013) 914–920. doi:10.1016/j.jbi.2013.07.011.
[12]. M. Lovric´, J. M. Molero, R. Kern, Pyspark and rdkit: Moving towards big data in cheminformatics, Molecular Informatics 38 (6) (2019). doi:10.1002/minf.201800082.
[13]. P. Chandak, PrimeKG (2022). doi:10.7910/DVN/IXA7BM. https://doi.org/10.7910/DVN/IXA7BM
[14]. S. Chithrananda, G. Grand, B. Ramsundar, Chemberta: Large-scale self-supervised pretraining for molecular prop- erty prediction (2020). arXiv:2010.09885.
[15]. Z. Lin, H. Akin, R. Rao, B. Hie, Z. Zhu, et al, Evolutionary-scale prediction of atomic level protein structure with a language model, bioRxiv (2022). arXiv:https://www.biorxiv.org/content/early/2022/10/31/2022. 07.20.500902.full.pdf, doi:10.1101/2022.07.20.500902. https://www.biorxiv.org/content/early/2022/10/31/2022.07.20.500902
[16]. Y. Feng, S. Zhang, Prediction of drug-drug interaction using an attention-based graph neural network on drug molecular graphs, Molecules (Basel, Switzerland) 27 (9) (2022) 3004. doi:10.3390/molecules27093004.
[17]. K. Shao, Y. Zhang, Y. Wen, Z. Zhang, S. He, X. Bo, Dti-heta: Prediction of drug–target interactions based on gcn and gat on heterogeneous graph, Briefings in Bioinformatics 23 (3) (2022). doi:10.1093/bib/bbac109.
[18]. A. Rossi, D. Barbosa, D. Firmani, A. Matinata, P. Merialdo, Knowledge graph embedding for link prediction: A comparative analysis, ACM Transactions on Knowledge Discovery from Data 15 (2) (2021) 1–49. doi:10.1145/ 3424672.
[19]. B. Tanoori, M. Z. Jahromi, E. G. Mansoori, Drug-target continuous binding affinity prediction using multiple sources of information, Expert Systems with Applications 186 (2021) 115810. doi:10.1016/j.eswa.2021. 115810.
Cite this article
Liu,H.;Li,S.;Yu,Z. (2024). Predicting drug-drug interactions using heterogeneous graph neural networks: HGNN-DDI. Applied and Computational Engineering,79,77-89.
Data availability
The datasets used and/or analyzed during the current study will be available from the authors upon reasonable request.
Disclaimer/Publisher's Note
The statements, opinions and data contained in all publications are solely those of the individual author(s) and contributor(s) and not of EWA Publishing and/or the editor(s). EWA Publishing and/or the editor(s) disclaim responsibility for any injury to people or property resulting from any ideas, methods, instructions or products referred to in the content.
About volume
Volume title: Proceedings of the 4th International Conference on Signal Processing and Machine Learning
© 2024 by the author(s). Licensee EWA Publishing, Oxford, UK. This article is an open access article distributed under the terms and
conditions of the Creative Commons Attribution (CC BY) license. Authors who
publish this series agree to the following terms:
1. Authors retain copyright and grant the series right of first publication with the work simultaneously licensed under a Creative Commons
Attribution License that allows others to share the work with an acknowledgment of the work's authorship and initial publication in this
series.
2. Authors are able to enter into separate, additional contractual arrangements for the non-exclusive distribution of the series's published
version of the work (e.g., post it to an institutional repository or publish it in a book), with an acknowledgment of its initial
publication in this series.
3. Authors are permitted and encouraged to post their work online (e.g., in institutional repositories or on their website) prior to and
during the submission process, as it can lead to productive exchanges, as well as earlier and greater citation of published work (See
Open access policy for details).