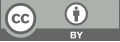
Predictive Models: Regression, Decision Trees, and Clustering
- 1 University of Washington
* Author to whom correspondence should be addressed.
Abstract
This paper explores three fundamental machine learning techniques—linear regression, k-means clustering, and decision trees—and their applications in predictive modeling. In the era of data proliferation, machine learning stands at the intersection of computer science and artificial intelligence, playing a pivotal role in algorithm and model development for enhanced predictions and decision-making. The study delves into the intricacies of these techniques, starting with a focus on linear regression, a supervised learning algorithm for establishing relationships between independent and dependent variables. The process involves data preparation, exploration, feature selection, model building, and evaluation. A practical example demonstrates the application of linear regression in analyzing the relationship between income and happiness. The exploration then extends to k-means clustering, an unsupervised learning algorithm used for grouping unlabeled datasets into distinct clusters. The iterative nature of k-means involves assigning data points to clusters based on centroid proximity, contributing to efficient data exploration. A graphical representation illustrates the step-by-step process of data point grouping and centroid recalibration. The advantages of k-means, including computational efficiency and simplicity, are discussed, along with considerations such as sensitivity to initialization and the manual specification of the number of clusters. The paper concludes with an examination of decision trees, versatile algorithms used for both classification and regression tasks. Decision trees construct hierarchical structures based on features, facilitating straightforward decision-making processes. A practical example illustrates how decision trees assess credit risk based on credit history and loan term. The strengths of decision trees, such as visual representation and non-linear pattern capture, are outlined, alongside considerations like overfitting. In summary, this paper provides insights into the strengths, limitations, and applications of linear regression, k-means clustering, and decision trees. These techniques offer valuable tools in data analysis and prediction, with their effectiveness dependent on specific problem domains and datasets. The study contributes to a comprehensive understanding of these machine learning methods and suggests future research directions, including exploring advanced variations and real-world applications.
Keywords
Machine Learning, Data Science, Predicting Model, Regression, Decision Trees, K-means Clustering, Supervised Learning, Unsupervised Learning, Data Prediction, Computational Efficiency.
[1]. “What Is Machine Learning?” IBM, www.ibm.com/topics/machine-learning. Accessed 21 Sept. 2023.
[2]. Tucci, Linda, and Ed Burns. “What Is Machine Learning and How Does It Work? In-Depth Guide.” Enterprise AI, TechTarget, 15 Sept. 2023, www.techtarget.com/searchenterpriseai/definition/machine-learning-ML.
[3]. K, H., Khanra, S., Rodriguez, R. V., & Jaramillo, J. (2022). Machine Learning for Business Analytics : Real-Time Data Analysis for Decision-Making. Productivity Press. https://doi.org/10.4324/9781003206316
[4]. bernie2436bernie2436 22.9k4949 gold badges151151 silver badges244244 bronze badges, et al. “What Is the Difference between Labeled and Unlabeled Data?” Stack Overflow, 1 Feb. 1960, stackoverflow.com/questions/19170603/what-is-the-difference-between-labeled-and-unlabeled-data.
[5]. “What Is a Decision Tree.” IBM, www.ibm.com/topics/decision-trees. Accessed 8 Oct. 2023.
[6]. “What Is Deep Learning?” IBM, www.ibm.com/topics/deep-learning. Accessed 22 Sept. 2023.
[7]. “Linear Regression for Machine Learning: Intro to ML Algorithms.” Edureka, 2 Aug. 2023, www.edureka.co/blog/linear-regression-for-machine-learning/#advantages.
[8]. “What Is a Machine Learning Pipeline?” What Is a Machine Learning Pipeline?, valohai.com/machine-learning-pipeline/. Accessed 7 Oct. 2023.
[9]. Bevans, Rebecca. “Simple Linear Regression: An Easy Introduction & Examples.” Scribbr, 22 June 2023, www.scribbr.com/statistics/simple-linear-regression/.
[10]. Trevino, Andrea. “Introduction to K-Means Clustering.” Oracle Blogs, blogs.oracle.com/ai-and-datascience/post/introduction-to-k-means-clustering. Accessed 7 Oct. 2023.
[11]. James, Gareth, et al. An Introduction to Statistical Learning: With Applications in R. Springer, 2022.
[12]. 8.5 K-Means Clustering - Github Pages, jermwatt.github.io/machine_learning_refined/notes/8_Linear_unsupervised_learning/8_5_Kmeans.html. Accessed 8 Oct. 2023.
[13]. Author: Fatih Karabiber Ph.D. in Computer Engineering, et al. “Cosine Similarity.” Learn Data Science - Tutorials, Books, Courses, and More, www.learndatasci.com/glossary/cosine-similarity/. Accessed 8 Oct. 2023.
[14]. Manhattan Distance, xlinux.nist.gov/dads/HTML/manhattanDistance.html. Accessed 8 Oct. 2023.
[15]. “K-Means Pros & Cons.” HolyPython.Com, 25 Mar. 2023, holypython.com/k-means/k-means-pros-cons/.
[16]. “Decision Tree Algorithm, Explained.” KDnuggets, www.kdnuggets.com/2020/01/decision-tree-algorithm-explained.html. Accessed 9 Oct. 2023.
[17]. Team, Towards AI. “Decision Trees Explained with a Practical Example.” Towards AI, 6 Jan. 2023, towardsai.net/p/programming/decision-trees-explained-with-a-practical-example-fe47872d3b53.
Cite this article
Huang,X. (2024). Predictive Models: Regression, Decision Trees, and Clustering. Applied and Computational Engineering,79,124-133.
Data availability
The datasets used and/or analyzed during the current study will be available from the authors upon reasonable request.
Disclaimer/Publisher's Note
The statements, opinions and data contained in all publications are solely those of the individual author(s) and contributor(s) and not of EWA Publishing and/or the editor(s). EWA Publishing and/or the editor(s) disclaim responsibility for any injury to people or property resulting from any ideas, methods, instructions or products referred to in the content.
About volume
Volume title: Proceedings of the 4th International Conference on Signal Processing and Machine Learning
© 2024 by the author(s). Licensee EWA Publishing, Oxford, UK. This article is an open access article distributed under the terms and
conditions of the Creative Commons Attribution (CC BY) license. Authors who
publish this series agree to the following terms:
1. Authors retain copyright and grant the series right of first publication with the work simultaneously licensed under a Creative Commons
Attribution License that allows others to share the work with an acknowledgment of the work's authorship and initial publication in this
series.
2. Authors are able to enter into separate, additional contractual arrangements for the non-exclusive distribution of the series's published
version of the work (e.g., post it to an institutional repository or publish it in a book), with an acknowledgment of its initial
publication in this series.
3. Authors are permitted and encouraged to post their work online (e.g., in institutional repositories or on their website) prior to and
during the submission process, as it can lead to productive exchanges, as well as earlier and greater citation of published work (See
Open access policy for details).