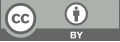
Music genre classification: Machine Learning on GTZAN
- 1 Jilin University Zhuhai Campus
- 2 Nanjing University of Information Science and Technology
- 3 Southwest Jiaotong University
- 4 Valley Catholic High School
* Author to whom correspondence should be addressed.
Abstract
This paper explores music genre classification, aiming to enhance existing methodologies. As a crucial aspect of music information retrieval, genre classification facilitates organization and recommendation in music databases and streaming services. Our research, inspired by a Kaggle project, examines the background of music genre classification and introduces improvements. The study focuses on data preparation techniques and a novel methodology using Support Vector Machines (SVM). Utilizing the GTZAN dataset, we applied data segmentation and feature extraction, employing machine learning algorithms like Logistic Regression, Random Forest, and SVM. A significant innovation is our segmentation technique based on music's beats per minute (BPM), designed to preserve rhythmic structure, believed to be essential for accurate classification. We explored various feature extraction methods to boost classifier performance. Experimental results showed the 3-second segmented dataset performed better with SVM's linear kernel. Additionally, a 4-beat segmentation experiment suggested that finer segmentation captures richer audio features, potentially improving classification accuracy. The paper concludes with findings and future research directions, including dataset expansion, advanced segmentation based on musical theory, deep learning applications, and developing real-time classification systems.
Keywords
Music Genre Classification, Kaggle, GTZAN Dataset, Support Vector Machines, Data Segmentation, Feature Extraction.
[1]. Gupta, S., Marwah, S., & Briskilal, J. (2022). AI Music Generator. Journal of Pharmaceutical Negative Results, 67-71.
[2]. Bahuleyan, H. (2018). Music genre classification using machine learning techniques. arXiv preprint arXiv:1804.01149.
[3]. Ghildiyal, A., Singh, K., & Sharma, S. (2020, November). Music genre classification using machine learning. In 2020 4th international conference on electronics, communication and aerospace technology (ICECA) (pp. 1368-1372). IEEE.
[4]. Lau, D. S., & Ajoodha, R. (2022). Music genre classification: A comparative study between deep learning and traditional machine learning approaches. In Proceedings of Sixth International Congress on Information and Communication Technology: ICICT 2021, London, Volume 4 (pp. 239-247). Springer Singapore.
[5]. Xu, J., Qu, W., Li, D., & Zhang, C. (2022, December). The Music Generation Road from Statistical Method to Deep Learning. In 2022 IEEE 8th International Conference on Computer and Communications (ICCC) (pp. 1628-1633). IEEE.
[6]. Deepaisarn, S., Chokphantavee, S., Chokphantavee, S., Prathipasen, P., Buaruk, S., & Sornlertlamvanich, V. (2023). NLP-based music processing for composer classification. Scientific Reports, 13(1), 13228.
Cite this article
Zhao,Z.;Xie,Z.;Fu,J.;Tian,X. (2024). Music genre classification: Machine Learning on GTZAN. Applied and Computational Engineering,79,219-233.
Data availability
The datasets used and/or analyzed during the current study will be available from the authors upon reasonable request.
Disclaimer/Publisher's Note
The statements, opinions and data contained in all publications are solely those of the individual author(s) and contributor(s) and not of EWA Publishing and/or the editor(s). EWA Publishing and/or the editor(s) disclaim responsibility for any injury to people or property resulting from any ideas, methods, instructions or products referred to in the content.
About volume
Volume title: Proceedings of the 4th International Conference on Signal Processing and Machine Learning
© 2024 by the author(s). Licensee EWA Publishing, Oxford, UK. This article is an open access article distributed under the terms and
conditions of the Creative Commons Attribution (CC BY) license. Authors who
publish this series agree to the following terms:
1. Authors retain copyright and grant the series right of first publication with the work simultaneously licensed under a Creative Commons
Attribution License that allows others to share the work with an acknowledgment of the work's authorship and initial publication in this
series.
2. Authors are able to enter into separate, additional contractual arrangements for the non-exclusive distribution of the series's published
version of the work (e.g., post it to an institutional repository or publish it in a book), with an acknowledgment of its initial
publication in this series.
3. Authors are permitted and encouraged to post their work online (e.g., in institutional repositories or on their website) prior to and
during the submission process, as it can lead to productive exchanges, as well as earlier and greater citation of published work (See
Open access policy for details).