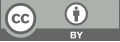
Research on credit risk assessment optimization based on machine learning
- 1 University of Michigan-Ann Arbor,Ann Arbor, MI, USA, 48109
- 2 Pepperdine University, 24255 Pacific Coast Hwy, Malibu, CA 90263
- 3 Columbia University, New York, 10027, USA
- 4 Peking University, Peking China, 100091
* Author to whom correspondence should be addressed.
Abstract
Credit business is a vital part of the bank's core business, which has an extremely important impact on the bank's income and development. In the operation of credit business, credit risk assessment is particularly crucial, and accurate risk assessment can minimize risks while maximizing the bank's returns. We propose a method to optimize credit risk assessment using machine learning techniques. In this work, we employ a random forest machine learning model to process and analyze large amounts of loan application data. By using correlation analysis, information enrichment, etc., the characteristics that have the most impact on credit risk assessment are screened. Subsequently, the model was constructed using a random forest algorithm. Random forests improve the generalization ability and accuracy of the model by building multiple decision trees and introducing randomness between these trees. In the experimental analysis part, we compare the performance of various models on the German credit dataset, and the results show that the deep learning model outperforms the traditional machine learning model in most indicators, verifying the effectiveness of our method.
Keywords
Credit Risk Assessment, Machine Learning, Random Forest, Correlation Analysis, Optimization
[1]. Lappas, Pantelis Z., and Athanasios N. Yannacopoulos. "A machine learning approach combining expert knowledge with genetic algorithms in feature selection for credit risk assessment." Applied Soft Computing 107 (2021): 107391.
[2]. Estran, Remy, Antoine Souchaud, and David Abitbol. "Using a genetic algorithm to optimize an expert credit rating model." Expert Systems with Applications 203 (2022): 117506.
[3]. Batchu, Ravi Kumar. "Artificial Intelligence in Credit Risk Assessment: Enhancing Accuracy and Efficiency." International Transactions in Artificial Intelligence 7.7 (2023): 1-24.
[4]. Qin, Chao, et al. "XGBoost optimized by adaptive particle swarm optimization for credit scoring." Mathematical Problems in Engineering 2021 (2021): 1-18.
[5]. Shi, Si, et al. "Machine learning-driven credit risk: a systemic review." Neural Computing and Applications 34.17 (2022): 14327-14339.
[6]. Breeden, Joseph. "A survey of machine learning in credit risk." Journal of Credit Risk 17.3 (2021).
[7]. Sohn, So Young, and Yoon Seong Kim. "Behavioral credit scoring model for technology-based firms that considers uncertain financial ratios obtained from relationship banking." Small Business Economics 41 (2013): 931-943.
[8]. Abid, Lobna, Afif Masmoudi, and Sonia Zouari-Ghorbel. "The consumer loan’s payment default predictive model: an application of the logistic regression and the discriminant analysis in a Tunisian commercial bank." Journal of the Knowledge Economy 9 (2018): 948-962.
[9]. Zhang Chenghu, Li Yulin, and Wu Ming. "Research and Empirical Analysis of Personal Credit Scoring Model Based on Discriminant Analysis." Journal of Dalian University of Technology: Social Sciences 1 (2009): 6-10.
[10]. Lecamwasam, Harish S., et al. "The flow regimes and the pressure‐flow relationship in the canine urethra." Neurourology and urodynamics 18.5 (1999): 521-541.
Cite this article
Zhang,X.;Xu,L.;Li,N.;Zou,J. (2024). Research on credit risk assessment optimization based on machine learning. Applied and Computational Engineering,69,173-178.
Data availability
The datasets used and/or analyzed during the current study will be available from the authors upon reasonable request.
Disclaimer/Publisher's Note
The statements, opinions and data contained in all publications are solely those of the individual author(s) and contributor(s) and not of EWA Publishing and/or the editor(s). EWA Publishing and/or the editor(s) disclaim responsibility for any injury to people or property resulting from any ideas, methods, instructions or products referred to in the content.
About volume
Volume title: Proceedings of the 6th International Conference on Computing and Data Science
© 2024 by the author(s). Licensee EWA Publishing, Oxford, UK. This article is an open access article distributed under the terms and
conditions of the Creative Commons Attribution (CC BY) license. Authors who
publish this series agree to the following terms:
1. Authors retain copyright and grant the series right of first publication with the work simultaneously licensed under a Creative Commons
Attribution License that allows others to share the work with an acknowledgment of the work's authorship and initial publication in this
series.
2. Authors are able to enter into separate, additional contractual arrangements for the non-exclusive distribution of the series's published
version of the work (e.g., post it to an institutional repository or publish it in a book), with an acknowledgment of its initial
publication in this series.
3. Authors are permitted and encouraged to post their work online (e.g., in institutional repositories or on their website) prior to and
during the submission process, as it can lead to productive exchanges, as well as earlier and greater citation of published work (See
Open access policy for details).