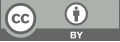
Chord sense: Enhancing stylistic Chord Progression generation with fine-tuned transformers
- 1 The School of Arts and Sciences, University of Rochester, Rochester 14627, the United States
* Author to whom correspondence should be addressed.
Abstract
Chord Progressions (CP) constitute a fundamental element within musical compositions. Skillful application of harmonies can captivate audiences through the colors and emotions they elicit. While existing research has predominantly focused on generating stylistically coherent CPs and accompaniments, relatively few studies have delved into the optimization of generating specific CPs of interest across diverse harmonic contexts. On this basis, this study aims to address this gap by fine-tuning a foundational CP model using datasets generated through three distinct strategies. Subsequently, the performances of the strategies are compared using both existing and novel evaluation metrics. According to the analysis, the results reveal that the model fine-tuned using the third strategy demonstrates proficiency in producing the target CPs across diverse contexts and modes of generation in a musically coherent manner. This approach opens up avenues for creative learning and sharing of stylistic chord progressions through exchanging customized fine-tuned chord models.
Keywords
Chord Progressions, transformer, style transfer, fine-tuning
[1]. Pasler J 2012 Notes vol 69(2) pp 197–216.
[2]. Hedges T, Roy P and Pachet F 2014 Journal of New Music Research vol 43(3) pp 276–290.
[3]. Morris D, Simon I and Basu S 2008 AAAI Conference on Artificial Intelligence vol 3.
[4]. Chuan C H and Chew E 2011 Computer Music Journal vol 35(4) pp 64–82.
[5]. Choi K, Fazekas G and Sandler M 2016 Text-based LSTM networks for Automatic Music Composition arXiv:1604.05358, 2016.
[6]. Carsault T, Nika J, Esling P and Assayag G 2021 Electronics vol 10(21) pp 2634.
[7]. Huang C Z A, Duvenaud D and Gajos K Z 2016 Proceedings of the 21st International Conference on Intelligent User Interfaces (IUI '16) pp 241–250.
[8]. Vaswani A, Shazeer N, Parmar N, Uszkoreit J, Jones L, Gomez A N, Kaiser L and Polosukhin I 2017 Advances in Neural Information Processing Systems pp 5998-6008.
[9]. Li S and Sung Y 2023 Mathematics vol 11(5) p 1111.
[10]. Chen T, Fukayama S, Goto M and Su L 2020 International Society for Music Information Retrieval Conference pp 360-367.
[11]. Dalmazzo D, Déguernel K, Sturm B L T 2024 Artificial Intelligence in Music Sound Art and Design EvoMUSART 2024 Lecture Notes in Computer Science vol 14633.
[12]. Fukayama S, Yoshii K and Goto M 2013 International Society for Music Information Retrieval Conference vol 457-462.
[13]. de Berardinis J, Meroño-Peñuela A, Poltronieri A et al 2023 Scientific Data vol 10(1) p 641.
[14]. Wu S L and Yang Y H 2020 The Jazz Transformer on the Front Line: Exploring the Shortcomings of AI-composed Music through Quantitative Measures arXiv preprint arXiv:2008.01307, 2020.
[15]. Steinbeis N, Koelsch S and Sloboda J 2006 Journal of cognitive neuroscience vol 18 pp 1380-1393.
[16]. Sarasso P, Perna P, Barbieri P et al 2021 Psychon Bull Rev vol 28 pp 1623–1637.
Cite this article
Ye,L. (2024). Chord sense: Enhancing stylistic Chord Progression generation with fine-tuned transformers. Applied and Computational Engineering,68,359-367.
Data availability
The datasets used and/or analyzed during the current study will be available from the authors upon reasonable request.
Disclaimer/Publisher's Note
The statements, opinions and data contained in all publications are solely those of the individual author(s) and contributor(s) and not of EWA Publishing and/or the editor(s). EWA Publishing and/or the editor(s) disclaim responsibility for any injury to people or property resulting from any ideas, methods, instructions or products referred to in the content.
About volume
Volume title: Proceedings of the 6th International Conference on Computing and Data Science
© 2024 by the author(s). Licensee EWA Publishing, Oxford, UK. This article is an open access article distributed under the terms and
conditions of the Creative Commons Attribution (CC BY) license. Authors who
publish this series agree to the following terms:
1. Authors retain copyright and grant the series right of first publication with the work simultaneously licensed under a Creative Commons
Attribution License that allows others to share the work with an acknowledgment of the work's authorship and initial publication in this
series.
2. Authors are able to enter into separate, additional contractual arrangements for the non-exclusive distribution of the series's published
version of the work (e.g., post it to an institutional repository or publish it in a book), with an acknowledgment of its initial
publication in this series.
3. Authors are permitted and encouraged to post their work online (e.g., in institutional repositories or on their website) prior to and
during the submission process, as it can lead to productive exchanges, as well as earlier and greater citation of published work (See
Open access policy for details).