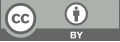
Word frequency statistics based on MapReduce on serverless platforms
- 1 School of Advanced Technology, Xi'an Jiaotong-Liverpool University, Suzhou, China
- 2 School of Mathematics and Statistics, Xuzhou University of Technology, Xuzhou, China
- 3 School of Communications Engineering, Xidian University, Xi'an, China
- 4 School of Computer Science, Chongqing University, Chongqing, China
* Author to whom correspondence should be addressed.
Abstract
This paper investigates the application of serverless computing in conjunction with the MapReduce framework, particularly in machine learning (ML) tasks. The MapReduce programming model has been widely used to process large-scale datasets by simplifying parallel and distributed data processing. This study explores how the combination of these two technologies can provide more efficient and cost-effective ML solutions. Through a detailed analysis of serverless environments and the MapReduce framework, this paper shows how the combination can advance the fields of cloud computing and machine learning. The experimental part includes the implementation of Map-Reduce model on a serverless platform, exploring the impact of different parameter settings on performance and improving efficiency by optimizing the data processing flow. In addition, the paper analyzes the use of memory and CPU resources and derives the relationship between dataset size, memory consumption and processor configuration and execution time. Through these experiments and analyses, this paper provides an empirical basis and theoretical support for the optimization of cloud computing frameworks.
Keywords
MapReduce, Serverless Computing, Cloud Computing, Efficient and Cost-Effective
[1]. Vouloumzidis, K., et al. 2019. A Systematic Literature Review of Serverless Computing: The First 10 Years. Journal of Cloud Computing,7(3), 255–281.
[2]. Dean, J., & Ghemawat, S. 2008. MapReduce: simplified data processing on large clusters. Communications of the ACM, 51(1), 107–113.
[3]. Wang, L., et al. 2018. "A Survey on Serverless Computing: Architecture, Applications, and Security." IEEE Access, vol. 6, pp. 31535–31553.
[4]. Zaharia, M., Chowdhury, M., Franklin, M. J., Shenker, S., & Stoica, I. 2010. Spark: Cluster computing with working sets. In 2nd USENIX Workshop on Hot Topics in Cloud Computing (HotCloud 10).
[5]. Verma, A., Cho, B., Zea, N., Gupta, I., & Campbell, R. H. 2013. Breaking the MapReduce stage barrier. Cluster computing, 16, 191-206.
[6]. Gorgolewski, K., Burns, C. D., Madison, C., Clark, D., Halchenko, Y. O., Waskom, M. L., & Ghosh, S. S. 2011. Nipype: a flexible, lightweight and extensible neuroimaging data processing framework in python. Frontiers in neuroinformatics, 5, 13.
[7]. Wang, G., & Chan, C. Y. 2013. Multi-query optimization in mapreduce framework. Proceedings of the VLDB Endowment, 7(3), 145-156.
[8]. Tari, Z. 2014. Security and privacy in cloud computing. IEEE Cloud Computing, 1(01), 54-57.
[9]. QIAN Wenjun, SHEN Qingni, WU Pengfei, et al. 2022. Progress of privacy protection technology in big data computing environment. Journal of Computing, 45(4), 669-701.
[10]. Ibtisum, S., Bazgir, E., Rahman, S. A., & Hossain, S. S. 2023. A comparative analysis of big data processing paradigms: Mapreduce vs. apache spark. World Journal of Advanced Research and Reviews, 20(1), 1089-1098.
Cite this article
He,Y.;Qian,J.;Zhang,J.;Zhang,R. (2024). Word frequency statistics based on MapReduce on serverless platforms. Applied and Computational Engineering,68,347-358.
Data availability
The datasets used and/or analyzed during the current study will be available from the authors upon reasonable request.
Disclaimer/Publisher's Note
The statements, opinions and data contained in all publications are solely those of the individual author(s) and contributor(s) and not of EWA Publishing and/or the editor(s). EWA Publishing and/or the editor(s) disclaim responsibility for any injury to people or property resulting from any ideas, methods, instructions or products referred to in the content.
About volume
Volume title: Proceedings of the 6th International Conference on Computing and Data Science
© 2024 by the author(s). Licensee EWA Publishing, Oxford, UK. This article is an open access article distributed under the terms and
conditions of the Creative Commons Attribution (CC BY) license. Authors who
publish this series agree to the following terms:
1. Authors retain copyright and grant the series right of first publication with the work simultaneously licensed under a Creative Commons
Attribution License that allows others to share the work with an acknowledgment of the work's authorship and initial publication in this
series.
2. Authors are able to enter into separate, additional contractual arrangements for the non-exclusive distribution of the series's published
version of the work (e.g., post it to an institutional repository or publish it in a book), with an acknowledgment of its initial
publication in this series.
3. Authors are permitted and encouraged to post their work online (e.g., in institutional repositories or on their website) prior to and
during the submission process, as it can lead to productive exchanges, as well as earlier and greater citation of published work (See
Open access policy for details).