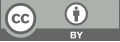
Cognitive machine learning techniques for predictive maintenance in industrial systems: A data-driven analysis
- 1 The University of Sydney, Sydney, Australia
- 2 The University of Adelaide, South Australia, Australia
- 3 The University of New South Wales, Sydney, Australia
* Author to whom correspondence should be addressed.
Abstract
This paper delves into the intricate relationship between machine learning (ML) and data analysis, spotlighting the recent advancements, prevailing challenges, and emerging opportunities that underscore their integration. By conducting an extensive review of scholarly literature and real-world case studies, this article uncovers the synergistic potential of ML and data analysis, emphasizing their combined influence across diverse industries and domains. The exploration is framed around pivotal themes including algorithmic innovations, which are at the heart of ML's ability to transform vast and complex datasets into actionable insights. Moreover, the discussion extends to predictive modeling techniques, a cornerstone of data analysis that leverages historical data to forecast future trends, behaviors, and outcomes. Practical applications are scrutinized to demonstrate how the confluence of ML and data analysis is pioneering solutions in fields as varied as healthcare, where predictive analytics can save lives, to finance, where it is used to navigate market uncertainties. This paper also addresses the barriers to effective integration, such as data privacy concerns and the need for robust data governance frameworks. Through this comprehensive examination, the article sheds light on the rapidly evolving landscape of ML-driven data analysis, offering insights into how these technological advancements are reshaping research methodologies, industry practices, and societal norms.
Keywords
Machine Learning, Data Analysis, Integration, Advancements.
[1]. Chong, Edwin KP, Wu-Sheng Lu, and Stanislaw H. Zak. An Introduction to Optimization: With Applications to Machine Learning. John Wiley & Sons, 2023.
[2]. Amini, Mahyar, and Ali Rahmani. "Agricultural databases evaluation with machine learning procedure." Australian Journal of Engineering and Applied Science 8.2023 (2023): 39-50.
[3]. Copeland, Robert A. Enzymes: a practical introduction to structure, mechanism, and data analysis. John Wiley & Sons, 2023.
[4]. Park, Minseok, and Nitya Prasad Singh. "Predicting supply chain risks through big data analytics: role of risk alert tool in mitigating business disruption." Benchmarking: An International Journal 30.5 (2023): 1457-1484.
[5]. Ness, Stephanie, Nicki James Shepherd, and Teo Rong Xuan. "Synergy Between AI and Robotics: A Comprehensive Integration." Asian Journal of Research in Computer Science 16.4 (2023): 80-94.
[6]. Rangineni, Sandeep, Divya Marupaka, and Arvind Kumar Bhardwaj. "An examination of machine learning in the process of data integration." International Journal of Computer Trends and Technology 71.6 (2023): 79-85.
[7]. Satam, Heena, et al. "Next-generation sequencing technology: Current trends and advancements." Biology 12.7 (2023): 997.
[8]. Iman, Mohammadreza, Hamid Reza Arabnia, and Khaled Rasheed. "A review of deep transfer learning and recent advancements." Technologies 11.2 (2023): 40.
[9]. Aldoseri, Abdulaziz, Khalifa N. Al-Khalifa, and Abdel Magid Hamouda. "Re-thinking data strategy and integration for artificial intelligence: concepts, opportunities, and challenges." Applied Sciences 13.12 (2023): 7082.
[10]. Ghazanfar, Shila, Carolina Guibentif, and John C. Marioni. "Stabilized mosaic single-cell data integration using unshared features." Nature biotechnology 42.2 (2024): 284-292.
Cite this article
Chai,Y.;Jin,L.;Zhang,W. (2024). Cognitive machine learning techniques for predictive maintenance in industrial systems: A data-driven analysis. Applied and Computational Engineering,87,47-53.
Data availability
The datasets used and/or analyzed during the current study will be available from the authors upon reasonable request.
Disclaimer/Publisher's Note
The statements, opinions and data contained in all publications are solely those of the individual author(s) and contributor(s) and not of EWA Publishing and/or the editor(s). EWA Publishing and/or the editor(s) disclaim responsibility for any injury to people or property resulting from any ideas, methods, instructions or products referred to in the content.
About volume
Volume title: Proceedings of the 6th International Conference on Computing and Data Science
© 2024 by the author(s). Licensee EWA Publishing, Oxford, UK. This article is an open access article distributed under the terms and
conditions of the Creative Commons Attribution (CC BY) license. Authors who
publish this series agree to the following terms:
1. Authors retain copyright and grant the series right of first publication with the work simultaneously licensed under a Creative Commons
Attribution License that allows others to share the work with an acknowledgment of the work's authorship and initial publication in this
series.
2. Authors are able to enter into separate, additional contractual arrangements for the non-exclusive distribution of the series's published
version of the work (e.g., post it to an institutional repository or publish it in a book), with an acknowledgment of its initial
publication in this series.
3. Authors are permitted and encouraged to post their work online (e.g., in institutional repositories or on their website) prior to and
during the submission process, as it can lead to productive exchanges, as well as earlier and greater citation of published work (See
Open access policy for details).