1. Introduction
Back in 2018, Ming-Hui Huang [1] discussed the impact of AI's tech ethics in the service industry in an article examining the substitutability of AI in terms of machines, foreseeing that in the future in the service industry, some simple tasks will be taken over by AI, resulting in the loss of personnel, which is seen as a transitional phase of augmentation, and then, when it has the ability to take over all the work tasks it will then completely replace human labour.
By 2022, Martin Reisenbichler [2] shows in his research that the emergence of AI has enabled the realisation of natural language generation to support content marketing, a study of the ethics of AI from the field of writing, pointing out that although machine-generated content is designed to perform well in search engines, the role of human editors is still vital.
Next in 2024, targeting the aspect of literary creation, Holden Thorp [3] tried in his experiment to ask generative AI to rewrite the first scene of the classic American play Death of a Salesman, but with Princess Elsa from the animated film Frozen as the main character instead of Willy Loman, which resulted in an amusing dialogue in which Elsa returns home after a hard day of selling, and her son, Harpy, says to her, "Come on, Mum, you're Elsa from Frozen. You have the power of ice and snow and you are the queen. You're unstoppable." Mashups like this are certainly interesting, but they have serious implications for generative AI programs like ChatGPT in science and academia. And not just in terms of literary creation. In the same year, also in terms of literary creation, Chris Stokel-Walker [4] noted that as generative AIs like ChatGPT become popular in 2023, major ethical discussions about their role in academic authorship have emerged. Prominent ethical organisations, including the ICMJE and COPE, as well as leading publishers, have developed ethical clauses that make it clear that these models don't meet authorship standards due to accountability issues.
Next, in 2024, Shiavax J Rao [5] argues in his article that AI, and in particular high-level language models like ChatGPT, have the potential to revolutionise all aspects of healthcare, medical education and research, reviewing the benefits of ChatGPT in personalising patient care, particularly in geriatric care, medication management, weight loss and nutrition, and sports activity instruction, with further insights into its potential for enhancing medical research through the analysis of large datasets and the development of new methods. In the field of medical education, to make ChatGPT an effective resource for medical students and professionals as an information retrieval tool and for personalised learning.ChatGPT has many promising applications that may trigger a paradigm shift in healthcare practice, education and research.The use of ChatGPT may be beneficial in the areas of clinical decision-making, geriatric care, medication management, weight loss and nutrition, physical fitness scientific research, and medical education, among other areas. However, it is worth noting that issues around ethics, data privacy, transparency, inaccuracy and inadequacy remain. The real-world impact of ChatGPT and generative AI must be objectively assessed using a risk-based approach before it can be widely used in medicine. It is not difficult to see that AI ethical issues, gradually from the ethical issues of man and machine, to the field of literary creation and then to the medical and other fields with greater relevance to people, generative AI technology ethical issues are destined to become an unavoidable problem for people in the future.
2. Research design
2.1. Analysis of questionnaire results
2.1.1. Questionnaire data collation
The data for this study came from a questionnaire that categorised the main reasons affecting the ethics of science and technology into five categories in the form of scales: "access to discriminatory search results", "inaccurate information answered", "misuse of information", "false content and malicious dissemination", and "impact on a person's ability to make autonomous decisions", with five specific measurable indicators in each category. misuse of information", "false content and malicious dissemination", and "impact on people's ability to make autonomous decisions", which are five specific and measurable indicators, and the sub-questions in each category are measured by a score from 1 to 10, and the average value will be calculated. degree, and derive the average value, classifying 1~4 as mild (a), 5~7 as moderate (b), and 8~10 as severe (c), and at the same time, respectively, using A=obtaining discriminatory search results, B=inaccurate information in the answers, C=information misuse, D=false content and malicious dissemination, and E=influence on people's autonomous decision-making ability, and, the questionnaire's "Perceived importance of AI" column, with scores of 1 to 5 as unimportant and scores of 6 to 10 as important, to facilitate the next decision tree C4.5 algorithm.
2.1.2. Questionnaire design and distribution
A total of 270 questionnaires were distributed in this research study, with response time of more than 200 seconds as the detection criterion, and excluding the text papers that did not meet the criteria, leaving a total of 221 valid questionnaires. The questionnaires were divided into three levels, namely young (10-28 years old), middle-aged (29-47 years old), and old (48-66 years old), and 30% of the respondents from each age group were selected as the final database, of which 89 were selected from the young, 110 from the middle-aged, and 22 from the old, and the questionnaires were taken as non-scaled questions to collect information and data analysis was done using SPSS.
2.2. Data analysis
2.2.1. Summary of basic information
Table 1. Analysis of Respondents by Industry
Industry Name |
frequency |
Percentage (%) |
Internet technology industry |
32 |
14.5 |
financial industry |
56 |
25.3 |
Consultancy services industry |
41 |
18.6 |
Education Industry |
46 |
20.8 |
Government and public interest organisations |
23 |
10.4 |
student at school |
17 |
7.7 |
the rest |
6 |
2.7 |
(grand) total |
221 |
100 |
In terms of industry distribution, 221 questionnaires were sent and 221 were valid, with the financial industry having the most practitioners with 56; the education industry followed with 46; and the third was the counselling services industry with 41, as shown in Table 2.
2.2.2. Next, let's look at the percentage of people who have used generative AI in each industry:
Table 2. Status of use of generative AI by industry
|
Internet technology industry |
financial industry |
Consultancy services industry |
|||
|
frequency |
per cent |
frequency |
per cent |
frequency |
per cent |
used up |
20 |
10 |
30 |
13.6 |
20 |
9 |
unused |
10 |
4.5 |
26 |
11.8 |
21 |
9.5 |
|
Education Industry |
Government and public interest organisations |
student population |
|||
|
frequency |
per cent |
frequency |
per cent |
frequency |
per cent |
used up |
19 |
8.6 |
11 |
5 |
7 |
3.2 |
unused |
27 |
12.2 |
12 |
5.4 |
9 |
4.1 |
Through the above table, we can see that although the number of employees in the financial industry is large, the number of people who have used generative AI and those who have not used it each accounts for about half, but the percentage of those who have used generative AI in the Internet industry reaches 10%, which is 5.5% higher than that of those who haven't used it, and the difference between the percentage of those who have used it and the percentage of those who haven't is -0.5%, -3.6%, and -0.4% respectively, indicating that the most widely exposed to and using generative AI is the group in the Internet technology industry. The percentage difference between those who have used it and those who haven't is -0.5%, -3.6%, and -0.4% respectively, indicating that the most widely exposed to and used generative AI is the group in the Internet technology industry.
Table 3. Statistics on the number of people who expect to use generative AI again
|
frequency |
Effective percentage |
lesser |
43 |
38.7 |
usual |
40 |
36 |
non-recurrent |
26 |
23.4 |
I can't get away from it. |
2 |
1.8 |
(grand) total |
111 |
100 |
The table shows that generative AI is not used very often among the people surveyed, and the number of people who use it generally versus less often amounts to 83, or 74.7%.
How many of those using generative AI have heard of the concept of tech ethics, as shown in Table 4.
Table 4. Perceptions of technology ethics among those who have used generative AI
|
frequency |
Effective percentage |
be |
55 |
50 |
clogged |
55 |
50 |
(grand)total |
110 |
100 |
As can be seen from the table above, there is exactly a 50/50 split between those who have heard of tech ethics and those who have not, but since those who have used generative AI accounted for 49.4% of the total survey respondents, it suggests that tech ethics is still a relatively new concept in the general public's perception.
2.3. Data modelling
We take the decision tree C4.5 model to measure the importance people attach to the ethical issue content of different generative AIs, so we have to calculate by INFO information, e information entropy, Gain information gain, Gain Rate information gain rate, and Gini coefficient [12].
First of all, we should calculate the binary classification result: whether it is important to organise the science and technology ethics of generative AI into "important" and "unimportant", which results in the number of important people as H, and the number of unimportant people as J. Then the total amount of information will be as in Expression 1.
\( INFO_{总}=I[H,J]=\frac{H}{H+J}\log_{2}{(\frac{H}{H+J})} - \frac{J}{H+J}\log_{2}{(\frac{J}{H+J})}\ \ \ (1) \)
Next, we want to calculate the information entropy. Each dataset is converted into a rank 1\2\3 (0~4 is classified as "1", 5~8 is classified as "2", and 9~11 is classified as "3") based on the scores Assuming that the set E ∈ ({A},{B},{C},{D},{E}), \( \ P_i \) is a probability distribution, the information entropy ENT (E) is as in Equation 2.
\( ENT(E)=\sum_{I=1}^{3} P_{i}\log_{2}{(P_{i})}\ \ \ (2) \)
After finding the information entropy \( \ ENT(E) \) , we calculate its information gain as in Equation 3.
\( Gain(E)=INFO_{总} - ENT(E)\ \ \ (3) \)
Next, the value of the split information is derived by setting the number of important and unimportant ratings corresponding to each classification to \( \ L_1 \) = number of important and \( \ L_2 \) = number of unimportant ratings, as in Equation 4.
\( SplitINFO_{E}= - \frac{L1}{L1+L2}\log_{2}{(\frac{L1}{L1+L2})} - \frac{L2}{L1+L2}\log_{2}{(\frac{L2}{L1+L2})}\ \ \ (4) \)
Using the information on it, the information gain ratio is calculated as in Equation 5.
\( GainRate(E)=\frac{Gain(E)}{SplitINFO_{E}}\ \ \ (5) \)
Meanwhile, the Gini coefficient is calculated as in Equation 6.
\( Ginicoefficient=1 - \sum_{}^{} (pi^{∧}2)\ \ \ (6) \)
2.4. Operating Mechanisms
In the above manner, repeat the calculation, select MAX as the node, to divide the root node, leaf nodes and the end point, until a branch is all "important" or "unimportant" so as to gradually draw a decision tree.
2.4.1. Data entry
Firstly, the data is divided into test set and training set in the ratio of 1:9. The parameters are designed as follows:
In the second step, in the training set, the root node is selected and the calculated values are given in Table 5 below.The set E has the largest gain information rate, so it is selected as the root node.
Next continue to repeat this arithmetic rule, you can plot the decision tree, as in Figure 1.
Table 5. Selection of root nodes
|
\( \mathrm{ENT}(E) \) |
\( \mathrm{Gain}(E) \) |
\( \mathrm{SplitINF}\mathrm{O}_E \) |
\( GainRate \) |
Weight value |
A |
0.26 |
0.037 |
1.416 |
0.026 |
0.272 |
B |
0.134 |
0.163 |
1.393 |
0.117 |
0.139 |
C |
0.125 |
0.172 |
1.436 |
0.120 |
0.162 |
D |
0.132 |
0.165 |
1.450 |
0.113 |
0.151 |
E |
0.14 |
0.157 |
1.163 |
0.135 |
0.276 |
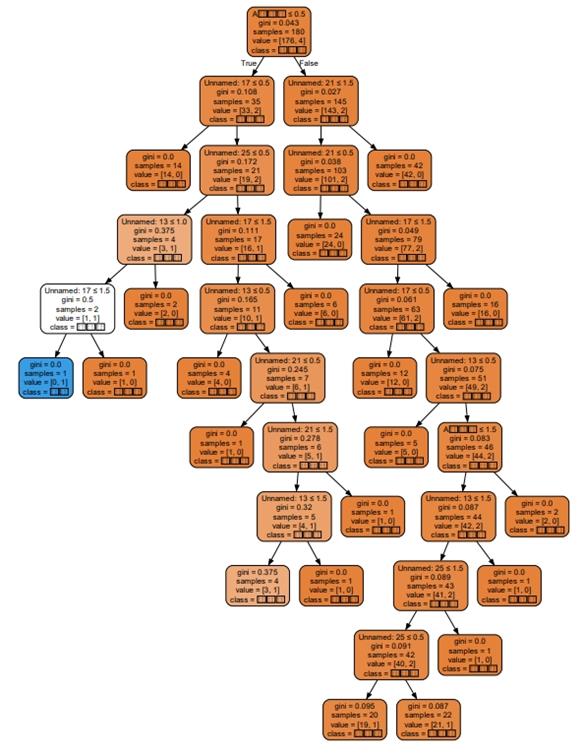
Figure 1. Decision Tree Model of Influencing Factors for Generative AI Tech Ethics
2.4.2. Prune (branches etc)
When using decision tree algorithms for classification or regression problems, pruning techniques are often employed to avoid overfitting. ccp_alpha is a pruning parameter that controls the strength of the pruning.
For each leaf node, we can compute an effective value with respect to ccp_alpha. Specifically, the effective value of each node is its reduced impurity (e.g., Gini impurity) minus a scaling factor, ccp_alpha, which is multiplied by the number of offspring of that node. Thus, if a node's effective value is less than zero, then that node will be pruned. the larger the ccp_alpha, the stronger the pruning and the simpler the final decision tree; conversely, the smaller the ccp_alpha, the weaker the pruning and the more complex the final decision tree.
If a node has a negative valid value, the node will be pruned out. Note that this is just an example and in practice different datasets and models may require different ccp_alpha values.
When using decision tree algorithms for classification or regression problems, we can control the complexity and generalisation ability of the model by adjusting the ccp_alpha parameter. Typically, we use techniques such as cross-validation to select the best ccp_alpha value to achieve the best model performance and generalisation ability.
2.4.3. Model testing
Table 6. Training set model evaluation results
term (in a mathematical formula) |
accuracy |
recall rate |
f1-score |
sample size |
usual |
0.61 |
0.59 |
0.60 |
37 |
significant |
0.90 |
0.90 |
0.90 |
143 |
accuracy |
|
|
0.84 |
180 |
average value |
0.75 |
0.75 |
0.75 |
180 |
Average (combined) |
0.84 |
0.84 |
0.84 |
180 |
Table 7. Test set model evaluation results
term (in a mathematical formula) |
accuracy |
recall rate |
f1-score |
sample size |
usual |
0.50 |
0.33 |
0.40 |
3 |
significant |
0.89 |
0.94 |
0.92 |
18 |
accuracy |
|
|
0.86 |
21 |
average value |
0.70 |
0.64 |
0.66 |
21 |
Average (combined) |
0.84 |
0.86 |
0.84 |
21 |
Table 8. Integrated model assessment
name (of a thing) | parameter name | parameter value |
Model parameter setting | Data preprocessing | Norm |
Training set ratio | 0.9 | |
Nodal split criteria | Gini | |
Node division method | Best | |
Minimum number of samples for node splitting | 2 | |
Leaf node minimum sample tree | 3 | |
Maximum tree depth | 10 | |
Modelling to assess effectiveness | accuracy | 85.714 per cent |
Precision rate (combined) | 83.835 per cent | |
Recall rate (combined) | 85.714 per cent | |
f1-score | 0.845 |
The above tables show the performance of the model on the training set and test set respectively.
First: accuracy rate, the proportion of samples with correct prediction results to the total samples, the accuracy rate training set is 0.84, the test set is 0.86, which belongs to the higher accuracy rate; Second: precision rate, the prediction results are positive in the results of the training set and the test set is greater than 0.5, the precision is better; Third: recall rate, the proportion of positive samples with positive predictions, except for the test set which is "general" is 0.33, the others are all greater than 0.5, indicating that the model has a high recall; Fourth: f1-score, is a comprehensive evaluation index that integrates the precision rate and the recall rate it is the reconciled average of the precision rate and the recall rate; Fifth: the higher the precision rate and the recall rate are, the better, but the two tend to contradict each other, so the f1-score is commonly used to integrate the precision rate and recall rate. Commonly used f1-score to comprehensively evaluate the effect of the classifier, which takes the value of the range of 0 to 1, the closer to 1 the better the effect, so the improvement of the model is better.
So in synthesis, it can be seen that: the final model obtained an accuracy of 85.71% on the test set, a precision (combined) of 83.83%, a recall (combined) of 85.71%, and an f1-score (combined) of 0.84. The model results are acceptable.
3. Conclusion
According to the model, among the people who think generative AI is important, item E "impact on human autonomous decision-making ability" is the most important impact of generative AI in technology ethics, and those who are concerned about item E are also more concerned about item A "obtaining discriminatory search results", followed by item C "misuse of information", while item B "inaccurate answer information" and item D "false content and malicious dissemination" are considered by people to be the most important impacts of generative AI. and "obtaining discriminatory search results" in item A, followed by "misuse of information" in item C. People are less concerned about "inaccurate information" in item B and "false content and malicious dissemination" in item D. Therefore, in practice, people will be more concerned about the "misuse of information" in item B and "inaccurate information" in item D. Therefore, in practice, if we want people to pay attention to the ethics of generative AI, we should focus on the impact of generative AI on human autonomy and the avoidance of discriminatory answers when formulating the ethical norms of generative AI, rather than just guaranteeing that the output of generative AI is accurate or not in order to govern the industry. At the same time, it is also necessary to strengthen people's attention to the misuse of information and privacy protection, such as: the establishment of science and technology ethics education courses in colleges and universities, included in the mandatory curriculum, and at the same time, the establishment of WeChat public number, or in the short video number of the continuous release of a series of science and technology ethics education courses and other ways to subconsciously influence the importance of science and technology ethics in the minds of the people.
References
[1]. Huang, M.-H., & Rust, R. T. (2018). Artificial Intelligence in Service. Journal of Service Research, 21(2), 155-172.
[2]. Aaker JL (1997) Dimensions of brand personality. J. Marketing Res. 34(3):347–356.
[3]. Nazarovets, S., & Teixeira da Silva, J. A. (2024). ChatGPT as an “author”: Bibliometric analysis to assess the validity of authorship. Accountability in Research, 1–11.
[4]. Stokel-Walker, C., & van Noorden, R. (2023). What ChatGPT and generative AI mean for science. Nature, 614, 214-216.
[5]. Rao, S.J., Isath, A., Krishnan, P. et al.(2024) ChatGPT: A Conceptual Review of Applications and Utility in the Field of Medicine. J Med Syst 48-59.
Cite this article
Xu,S. (2024). Decision tree C4.5 algorithm for generative AI technology ethics--Based on the results of the questionnaire. Applied and Computational Engineering,87,33-40.
Data availability
The datasets used and/or analyzed during the current study will be available from the authors upon reasonable request.
Disclaimer/Publisher's Note
The statements, opinions and data contained in all publications are solely those of the individual author(s) and contributor(s) and not of EWA Publishing and/or the editor(s). EWA Publishing and/or the editor(s) disclaim responsibility for any injury to people or property resulting from any ideas, methods, instructions or products referred to in the content.
About volume
Volume title: Proceedings of the 6th International Conference on Computing and Data Science
© 2024 by the author(s). Licensee EWA Publishing, Oxford, UK. This article is an open access article distributed under the terms and
conditions of the Creative Commons Attribution (CC BY) license. Authors who
publish this series agree to the following terms:
1. Authors retain copyright and grant the series right of first publication with the work simultaneously licensed under a Creative Commons
Attribution License that allows others to share the work with an acknowledgment of the work's authorship and initial publication in this
series.
2. Authors are able to enter into separate, additional contractual arrangements for the non-exclusive distribution of the series's published
version of the work (e.g., post it to an institutional repository or publish it in a book), with an acknowledgment of its initial
publication in this series.
3. Authors are permitted and encouraged to post their work online (e.g., in institutional repositories or on their website) prior to and
during the submission process, as it can lead to productive exchanges, as well as earlier and greater citation of published work (See
Open access policy for details).
References
[1]. Huang, M.-H., & Rust, R. T. (2018). Artificial Intelligence in Service. Journal of Service Research, 21(2), 155-172.
[2]. Aaker JL (1997) Dimensions of brand personality. J. Marketing Res. 34(3):347–356.
[3]. Nazarovets, S., & Teixeira da Silva, J. A. (2024). ChatGPT as an “author”: Bibliometric analysis to assess the validity of authorship. Accountability in Research, 1–11.
[4]. Stokel-Walker, C., & van Noorden, R. (2023). What ChatGPT and generative AI mean for science. Nature, 614, 214-216.
[5]. Rao, S.J., Isath, A., Krishnan, P. et al.(2024) ChatGPT: A Conceptual Review of Applications and Utility in the Field of Medicine. J Med Syst 48-59.