1. Introduction
The advent of artificial intelligence (AI) and machine learning (ML) has transformed numerous industries by enabling more accurate predictions, optimized decision-making, and personalized user experiences. Among various AI techniques, stochastic processes and reinforcement learning (RL) have emerged as powerful tools for strategic planning and decision support. Stochastic processes incorporate randomness into mathematical models, allowing for the prediction and analysis of systems that evolve over time. This capability is particularly valuable in environments characterized by uncertainty and variability, such as financial markets, supply chains, and healthcare. Reinforcement learning focuses on how agents can learn to make optimal decisions through interactions with their environment, maximizing cumulative rewards. By combining these two approaches, organizations can develop adaptive decision-making frameworks that are resilient to changes and capable of optimizing outcomes in real time. The integration of stochastic processes and RL offers a compelling solution to the complexities of modern strategic decision-making. In financial services, stochastic models can forecast market trends while RL algorithms optimize trading strategies to maximize returns. In logistics, these technologies can predict traffic patterns and dynamically adjust routing and scheduling, reducing operational costs and improving service delivery. Healthcare applications include patient outcome prediction and treatment plan optimization, enhancing personalized care and improving patient outcomes [1]. Retailers leverage these AI techniques for demand forecasting and inventory management, leading to increased sales and optimized stock levels. In manufacturing, predictive maintenance systems use stochastic models and RL to anticipate equipment failures and schedule timely repairs, minimizing downtime and maintenance costs. Despite their potential, the adoption of stochastic processes and RL faces challenges such as computational complexity, data quality, and ethical considerations, which must be addressed to fully realize the benefits of these technologies.
2. Theoretical Foundations
2.1. Stochastic Processes in Decision Support
Stochastic processes are mathematical models that incorporate randomness and are used to predict and analyze systems that evolve over time. In the context of decision support, these processes enable the modeling of uncertainties and variabilities inherent in strategic planning. For instance, in supply chain management, stochastic models can forecast demand fluctuations, helping businesses to optimize inventory levels and minimize costs. By incorporating random variables and probabilistic distributions, organizations can develop more resilient strategies that account for potential risks and uncertainties, ultimately leading to better-informed decisions. These models are also essential in financial risk management, where they help in assessing the volatility of asset prices and the likelihood of various economic scenarios. The use of stochastic processes in these areas ensures that decisions are based on a comprehensive analysis of possible outcomes, reducing the impact of unforeseen events. Figure 1 illustrates the impact of stochastic processes in decision support for supply chain management and financial risk management [2].
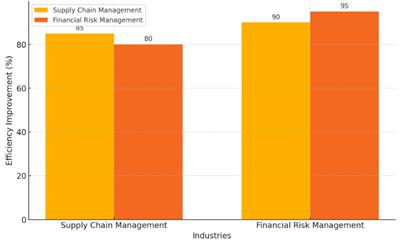
Figure 1. Impact Of Stochastic Processes In Decision Support
2.2. Reinforcement Learning Mechanisms
Reinforcement learning (RL) is a dynamic area of AI that focuses on how agents ought to take actions in an environment to maximize cumulative rewards. In RL, agents learn to make decisions by interacting with their environment, receiving feedback in the form of rewards or penalties. This learning paradigm is particularly effective in scenarios where the optimal strategy is not immediately obvious and must be discovered through exploration. For example, in automated trading systems, RL algorithms can learn to adjust trading strategies based on market conditions, thereby maximizing profits. The ability of RL to adapt and optimize decision-making processes in real-time makes it a powerful tool for strategic planning. Furthermore, RL has applications in robotics, where it enables machines to learn complex tasks through trial and error, improving their performance over time. The flexibility and adaptability of RL make it suitable for a wide range of applications, from game playing to industrial automation [3]. One fundamental equation in RL is the Bellman equation, which is central to many RL algorithms:
\( Q^{π}(s,a)=E_{π}[r_{t}+γ∙Q^{π}(s_{t+1}, a_{t+1})s_{t}=s,a_{t}=a]\ \ \ (1) \)
Where:
𝑄𝜋(𝑠,𝑎) is the action-value function, representing the expected return (sum of rewards) after taking action 𝑎a in state 𝑠s and following policy 𝜋. 𝑟𝑡 is the reward received after taking action 𝑎a in state 𝑠s. 𝛾 is the discount factor, which determines the importance of future rewards ( 0≤γ≤1 ). 𝑠𝑡+1 and 𝑎𝑡+1 are the state and action at the next time step. The Bellman equation can be used to iteratively improve the policy 𝜋 by updating the action-value function 𝑄. This iterative process is a key mechanism in RL algorithms like Q-learning and Deep Q-Networks (DQN).
2.3. Synergy Between Stochastic Processes and RL
The integration of stochastic processes and reinforcement learning creates a powerful synergy for decision support systems. Stochastic processes provide a structured way to model uncertainties and predict outcomes, while RL algorithms utilize these models to learn and optimize strategies over time. This combination allows for the development of adaptive decision-making frameworks that can respond to changing conditions and uncertainties. For instance, in personalized advertisement recommendation, stochastic models can predict user behavior patterns, and RL can adjust ad placements based on these predictions to enhance user engagement and conversion rates [4]. This synergy is also evident in healthcare, where predictive models can anticipate patient outcomes, and RL can optimize treatment plans based on these predictions. By combining these approaches, organizations can achieve a higher level of precision and efficiency in their decision-making processes, leading to improved outcomes and greater operational effectiveness.
3. Practical Implementations
3.1. Strategic Decision Support Systems
Table 1. Strategic Decision Support Systems (SDSS) applications
Industry |
Application |
Stochastic Model |
Reinforcement Learning |
Benefits |
Financial Services |
Market trend forecasting and trading strategy development |
ARIMA, GARCH |
Q-learning, Deep Q Networks |
Maximized returns, reduced financial risk |
Logistics |
Routing and scheduling optimization based on traffic predictions |
Poisson Process, Markov Chains |
Policy Gradient Methods, Actor-Critic Models |
Reduced operational costs, improved service delivery |
Healthcare |
Patient outcome prediction and treatment plan optimization |
Bayesian Networks, Hidden Markov Models |
Deep Q Networks, Actor-Critic Methods |
Personalized treatment plans, improved patient outcomes |
Retail |
Demand forecasting and inventory management |
Exponential Smoothing, Monte Carlo Simulation |
Multi-Armed Bandit, Deep Q Networks |
Optimized inventory levels, increased sales |
Manufacturing |
Predictive maintenance and repair scheduling |
Weibull Distribution, Gamma Process |
Deep Q Networks, Policy Gradient Methods |
Reduced downtime, lower maintenance costs |
Strategic decision support systems (SDSS) leverage AI technologies to assist organizations in making informed decisions. By integrating stochastic processes and RL, these systems can analyze vast amounts of data, identify trends, and optimize decision-making strategies. For example, in financial services, SDSS can use stochastic models to forecast market trends and RL to develop trading strategies that maximize returns. The combination of these technologies enables businesses to navigate complex environments with greater confidence, making more accurate and strategic decisions. In logistics, SDSS can optimize routing and scheduling by predicting traffic patterns and adjusting plans in real-time. This results in reduced operational costs and improved service delivery. The versatility of SDSS in various industries underscores its importance as a tool for enhancing organizational performance and competitiveness [5]. Table 1 includes various industries, their respective applications, the stochastic models and reinforcement learning methods used, and the benefits gained from implementing these systems.
3.2. Personalized Advertisement Recommendations
Personalized advertisement recommendation systems aim to deliver tailored ad content to individual users based on their preferences and behavior. By utilizing stochastic processes to model user interactions and RL to optimize ad placements, these systems can significantly enhance ad relevance and user engagement. For instance, e-commerce platforms can use stochastic models to predict user purchase patterns and RL algorithms to recommend products that align with these patterns. This personalized approach not only improves user experience but also increases the likelihood of conversion, making advertising efforts more effective [6]. Additionally, in streaming services, personalized recommendations can enhance viewer satisfaction by suggesting content that aligns with their viewing history and preferences. This leads to increased user retention and engagement, driving higher revenue for the service providers. The ability to deliver highly relevant content is a key advantage of personalized recommendation systems, making them an essential component of modern marketing strategies.
3.3. Case Studies in Industry
The practical application of stochastic processes and reinforcement learning (RL) has led to significant advancements across various industries. In the financial sector, a leading investment firm implemented an SDSS that combined ARIMA models for market trend forecasting with Deep Q Networks (DQN) for trading strategy optimization. This integration reduced financial risks and enhanced trading performance. In logistics, a global company adopted an SDSS using Poisson processes for traffic prediction and Policy Gradient Methods for dynamic routing and scheduling, resulting in lower operational costs and improved service delivery. In healthcare, a hospital network utilized Bayesian Networks and Actor-Critic Methods to predict patient outcomes and optimize treatment plans, leading to better patient care. A prominent retail chain used Exponential Smoothing models and Multi-Armed Bandit algorithms for demand forecasting and inventory management, increasing sales and reducing stockouts. In manufacturing, a predictive maintenance system employing Weibull Distribution models and Deep Q Networks minimized downtime and maintenance costs by accurately predicting equipment failures and scheduling timely repairs [7]. These case studies demonstrate the versatility and effectiveness of integrating stochastic processes and RL in various industries, driving innovation, efficiency, and improved decision-making.
4. Challenges and Limitations
4.1. Computational Complexity
One of the primary challenges associated with implementing stochastic processes and RL is the computational complexity involved. Both technologies require significant computational resources to process large datasets and perform complex calculations. This complexity can be a barrier to adoption, particularly for smaller organizations with limited resources. Additionally, the need for specialized expertise to develop and maintain these systems further complicates their implementation. Addressing these challenges requires advancements in computational efficiency and the development of user-friendly tools that can simplify the adoption process. High-performance computing infrastructure and cloud-based solutions can mitigate some of these challenges, providing scalable resources for organizations to leverage these advanced AI techniques [8]. Continuous research and development in this area are essential to making these technologies more accessible and practical for widespread use. Table 2 provides a concise overview of the challenges and solutions related to computational complexity in the context of stochastic processes and RL, highlighting the benefits of addressing these challenges.
Table 2. Computational Complexity Challenges And Solutions
Challenge |
Impact |
Solution |
Benefits |
High Computational Requirements |
Increased operational costs |
High-performance computing infrastructure |
Reduced costs, faster processing |
Large Dataset Processing |
Long processing times |
Efficient data processing algorithms |
Timely data analysis, enhanced performance |
Complex Calculations |
High error rates without precise calculations |
Advanced mathematical techniques |
Accurate results, improved decision making |
Need for Specialized Expertise |
Difficulty in system development and maintenance |
User-friendly development tools |
Simplified development, easier maintenance |
Resource Limitations for Smaller Organizations |
Barrier to adoption |
Cloud-based scalable resources |
Access to advanced AI techniques, increased adoption |
4.2. Data Quality and Availability
The effectiveness of stochastic processes and RL heavily depends on the quality and availability of data. Inaccurate or incomplete data can lead to suboptimal models and flawed decision-making processes. Ensuring data integrity and access to relevant datasets is crucial for the successful implementation of these technologies. Furthermore, the dynamic nature of real-world environments means that data must be continuously updated to reflect current conditions. Organizations must establish robust data management practices and invest in data acquisition and maintenance to overcome these challenges [9]. Data governance frameworks and advanced data cleaning techniques can enhance data quality, ensuring that AI models are built on reliable and accurate information. Collaboration with data providers and the development of industry-specific data standards can also improve data availability, supporting the effective use of AI technologies.
4.3. Ethical and Privacy Concerns
The use of AI technologies in decision support and personalized advertising raises ethical and privacy concerns. The collection and analysis of user data for personalized recommendations can infringe on privacy rights, and biased algorithms can lead to unfair or discriminatory outcomes. Ensuring transparency, fairness, and accountability in AI systems is essential to addressing these concerns. Organizations must implement ethical guidelines and comply with regulatory standards to protect user privacy and promote the responsible use of AI technologies. Ethical AI practices involve designing algorithms that are explainable and auditable, allowing stakeholders to understand and trust the decision-making processes [10]. Regular audits and impact assessments can help identify and mitigate potential biases, ensuring that AI systems operate fairly and ethically. By prioritizing ethical considerations, organizations can build trust with users and create AI systems that benefit society as a whole.
5. Conclusion
The integration of stochastic processes and reinforcement learning in strategic decision support systems and personalized advertisement recommendations has the potential to revolutionize traditional business practices. By modeling uncertainties and optimizing decision-making processes in real time, these technologies enhance organizational performance, reduce costs, and improve service delivery. Case studies across various industries demonstrate the practical benefits and versatility of these AI techniques, showcasing their ability to drive innovation and efficiency. However, challenges such as computational complexity, data quality, and ethical considerations must be addressed to ensure successful implementation. Enhancing computational efficiency, improving data management practices, and developing robust ethical AI guidelines are essential steps towards making these technologies more accessible and practical for widespread use. Looking to the future, advancements in quantum computing, more sophisticated algorithms, and enhanced data processing capabilities promise to further amplify the impact of stochastic processes and RL. Additionally, interdisciplinary collaboration will be crucial in overcoming current limitations and exploring new applications. As research and development in this field continue to evolve, the potential for these technologies to achieve even greater precision and efficiency in decision-making frameworks is vast. Embracing these advancements will enable organizations to stay competitive and innovative in an increasingly complex and dynamic environment.
References
[1]. Wang, Fuzhang, et al. “Artificial intelligence and stochastic optimization algorithms for the chaotic datasets.” FRACTALS (fractals) 31.06 (2023): 1-14.
[2]. Albergo, Michael S., Nicholas M. Boffi, and Eric Vanden-Eijnden. “Stochastic interpolants: A unifying framework for flows and diffusions.” arXiv preprint arXiv:2303.08797 (2023).
[3]. Faulwasser, Timm, et al. “Behavioral theory for stochastic systems? A data-driven journey from Willems to Wiener and back again.” Annual Reviews in Control (2023).
[4]. Dutordoir, Vincent, et al. “Neural diffusion processes.” International Conference on Machine Learning. PMLR, 2023.
[5]. Rosati, Riccardo, et al. “From knowledge-based to big data analytic model: a novel IoT and machine learning based decision support system for predictive maintenance in Industry 4.0.” Journal of Intelligent Manufacturing 34.1 (2023): 107-121.
[6]. Higgins, Oliver, et al. “Artificial intelligence (AI) and machine learning (ML) based decision support systems in mental health: An integrative review.” International Journal of Mental Health Nursing 32.4 (2023): 966-978.
[7]. Ali, Md Ramjan, Shah Md Ashiquzzaman Nipu, and Sharfuddin Ahmed Khan. “A decision support system for classifying supplier selection criteria using machine learning and random forest approach.” Decision Analytics Journal 7 (2023): 100238.
[8]. Valluri, Durga Deepak. “Exploring cognitive reflection for decision-making in robots: Insights and implications.” International Journal of Science and Research Archive 11.2 (2024): 518-530.
[9]. Rolf, Benjamin, et al. “A review on reinforcement learning algorithms and applications in supply chain management.” International Journal of Production Research 61.20 (2023): 7151-7179.
[10]. Hasan, MD Rokibul. “Addressing Seasonality and Trend Detection in Predictive Sales Forecasting: A Machine Learning Perspective.” Journal of Business and Management Studies 6.2 (2024): 100-109.
Cite this article
Ma,Q. (2024). Applications of stochastic processes and reinforcement learning in strategic decision support and personalized ad recommendation: An AIGC study. Applied and Computational Engineering,87,66-71.
Data availability
The datasets used and/or analyzed during the current study will be available from the authors upon reasonable request.
Disclaimer/Publisher's Note
The statements, opinions and data contained in all publications are solely those of the individual author(s) and contributor(s) and not of EWA Publishing and/or the editor(s). EWA Publishing and/or the editor(s) disclaim responsibility for any injury to people or property resulting from any ideas, methods, instructions or products referred to in the content.
About volume
Volume title: Proceedings of the 6th International Conference on Computing and Data Science
© 2024 by the author(s). Licensee EWA Publishing, Oxford, UK. This article is an open access article distributed under the terms and
conditions of the Creative Commons Attribution (CC BY) license. Authors who
publish this series agree to the following terms:
1. Authors retain copyright and grant the series right of first publication with the work simultaneously licensed under a Creative Commons
Attribution License that allows others to share the work with an acknowledgment of the work's authorship and initial publication in this
series.
2. Authors are able to enter into separate, additional contractual arrangements for the non-exclusive distribution of the series's published
version of the work (e.g., post it to an institutional repository or publish it in a book), with an acknowledgment of its initial
publication in this series.
3. Authors are permitted and encouraged to post their work online (e.g., in institutional repositories or on their website) prior to and
during the submission process, as it can lead to productive exchanges, as well as earlier and greater citation of published work (See
Open access policy for details).
References
[1]. Wang, Fuzhang, et al. “Artificial intelligence and stochastic optimization algorithms for the chaotic datasets.” FRACTALS (fractals) 31.06 (2023): 1-14.
[2]. Albergo, Michael S., Nicholas M. Boffi, and Eric Vanden-Eijnden. “Stochastic interpolants: A unifying framework for flows and diffusions.” arXiv preprint arXiv:2303.08797 (2023).
[3]. Faulwasser, Timm, et al. “Behavioral theory for stochastic systems? A data-driven journey from Willems to Wiener and back again.” Annual Reviews in Control (2023).
[4]. Dutordoir, Vincent, et al. “Neural diffusion processes.” International Conference on Machine Learning. PMLR, 2023.
[5]. Rosati, Riccardo, et al. “From knowledge-based to big data analytic model: a novel IoT and machine learning based decision support system for predictive maintenance in Industry 4.0.” Journal of Intelligent Manufacturing 34.1 (2023): 107-121.
[6]. Higgins, Oliver, et al. “Artificial intelligence (AI) and machine learning (ML) based decision support systems in mental health: An integrative review.” International Journal of Mental Health Nursing 32.4 (2023): 966-978.
[7]. Ali, Md Ramjan, Shah Md Ashiquzzaman Nipu, and Sharfuddin Ahmed Khan. “A decision support system for classifying supplier selection criteria using machine learning and random forest approach.” Decision Analytics Journal 7 (2023): 100238.
[8]. Valluri, Durga Deepak. “Exploring cognitive reflection for decision-making in robots: Insights and implications.” International Journal of Science and Research Archive 11.2 (2024): 518-530.
[9]. Rolf, Benjamin, et al. “A review on reinforcement learning algorithms and applications in supply chain management.” International Journal of Production Research 61.20 (2023): 7151-7179.
[10]. Hasan, MD Rokibul. “Addressing Seasonality and Trend Detection in Predictive Sales Forecasting: A Machine Learning Perspective.” Journal of Business and Management Studies 6.2 (2024): 100-109.