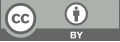
Analysis and comparison of sensor accuracy of autonomous vehicles
- 1 Changsha WES Academy, Changsha, China
* Author to whom correspondence should be addressed.
Abstract
Autonomous driving is gradually becoming one of the major directions in the development of automotive technology nowadays. Environmental detection technology is indispensable for existing intelligent vehicles, especially when such cars are used in daily life, where many complex road environments cannot be helped by environmental detection technology. Environmental detection technology cannot be divorced from hardware and software support. This study will discuss the different sensors used in autonomous driving environment detection technology, to gain a deeper understanding of the characteristics, functions and applications of these sensors, and to discuss the advantages and limitations of each sensor. Then a comparison of the three widely used sensors in the world is conducted, in terms of the detection range and angle, the accuracy of road detection, and the stability of the detection. The three sensors are a camera, millimeter wave radar and laser radar. A more suitable and stable one from these three sensors will be chosen for in-depth consideration. On this basis, new ideas for the future development of existing sensors are provided, while the direction of improvement of existing sensors is summarized based on the results of existing analyses.
Keywords
Environmental perception, driverless cars, sensors
[1]. Hoss, M., Scholtes, M., & Eckstein, L. (2022). A Review of Testing Object-Based Environment Perception for Safe Automated Driving. Automot. Innov, 5, 223–250. https://doi.org/10.1007/s42154-021-00172-y
[2]. Yuan, Q., Peng, Y., Xu, X. D., & Wang, X. H. (2021). Key points of investigation and analysis on traffic accidents involving intelligent vehicles. Transportation Safety and Environment, 3(4), tdab020. https://doi.org/10.1093/tse/tdab020
[3]. Song, Z., & Deng, H. (2023). Research on Sensor Optimization Technology of Driverless Vehicle. Frontiers in Computing and Intelligent Systems, 4(2), 131-137. https://doi.org/10.54097/fcis.v4i2.10370
[4]. Wang, P.(2021). Research on Comparison of LiDAR and Camera in Autonomous Driving. Journal of Physics: Conference Series, 2093 012032. https://doi.org/10.1088/1742-6596/2093/1/012032
[5]. Ye, L., Duan, T., & Zhu, J. (2020). Neural network-based semantic segmentation model forrobot perception of driverless vision. IET Cyber-Syst. Robot, 2(4), 190–196. https://doi.org/10.1049/iet-csr.2020.0040
[6]. Ignatious, H.A., El- Sayed, H., & Khan, M. (2022). An overview of sensors in Autonomous Vehicles. Procedia Computer Science, 198, 736-741.https://doi.org/10.1016/j.procs.2021.12.315
[7]. De, J. Y., Velasco-Hernandez, G., Barry, J., & Walsh, J. (2021). Sensor and Sensor Fusion Technology in Autonomous Vehicles: A Review. Sensors 2021, 21(6), 2140-2177.https://doi.org/10.3390/s21062140
[8]. Zhou, Y., Lu, L., Zhao, H., López-Benítez, M., Yu, L., & Yue, Y. (2022). Towards Deep Radar Perception for Autonomous Driving: Datasets, Methods, and Challenges. Sensors 2022, 22(11), 4208-4253. https://doi.org/10.3390/s22114208
[9]. Pei, S. et al. (2020). Scalability in Perception for Autonomous Driving: Waymo Open Dataset. 2020 IEEE/CVF Conference on Computer Vision and Pattern Recognition (CVPR), Seattle, WA, USA,2020, 2443-2451. https://doi.org/10.1109/CVPR42600.2020.00252
[10]. Bathla, G., Bhadane, K., Singh, R. K., Kumar, R., Aluvalu, R., Krishnamurthi, R., Kumar, A., Thakur, R. N., & Basheer, S. (2022). Autonomous Vehicles and Intelligent Automation: Applications, Challenges, and Opportunities. Mobile Information Systems, 7632892, 1-36. https://doi.org/10.1155/2022/7632892
Cite this article
Chen,H. (2024). Analysis and comparison of sensor accuracy of autonomous vehicles. Applied and Computational Engineering,93,1-6.
Data availability
The datasets used and/or analyzed during the current study will be available from the authors upon reasonable request.
Disclaimer/Publisher's Note
The statements, opinions and data contained in all publications are solely those of the individual author(s) and contributor(s) and not of EWA Publishing and/or the editor(s). EWA Publishing and/or the editor(s) disclaim responsibility for any injury to people or property resulting from any ideas, methods, instructions or products referred to in the content.
About volume
Volume title: Proceedings of the 2nd International Conference on Machine Learning and Automation
© 2024 by the author(s). Licensee EWA Publishing, Oxford, UK. This article is an open access article distributed under the terms and
conditions of the Creative Commons Attribution (CC BY) license. Authors who
publish this series agree to the following terms:
1. Authors retain copyright and grant the series right of first publication with the work simultaneously licensed under a Creative Commons
Attribution License that allows others to share the work with an acknowledgment of the work's authorship and initial publication in this
series.
2. Authors are able to enter into separate, additional contractual arrangements for the non-exclusive distribution of the series's published
version of the work (e.g., post it to an institutional repository or publish it in a book), with an acknowledgment of its initial
publication in this series.
3. Authors are permitted and encouraged to post their work online (e.g., in institutional repositories or on their website) prior to and
during the submission process, as it can lead to productive exchanges, as well as earlier and greater citation of published work (See
Open access policy for details).