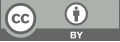
Weather prediction using CNN-LSTM for time series analysis: A case study on Delhi temperature data
- 1 Glasgow College, University of Electronic Science and Technology of China, Chengdu, China
- 2 Viterbi School of Engineering, University of Southern California, Los Angeles, California, USA
* Author to whom correspondence should be addressed.
Abstract
As global climate change intensifies, accurate weather forecasting is increasingly crucial for sectors such as agriculture, energy management, and environmental protection. Traditional methods, which rely on physical and statistical models, often struggle with complex, nonlinear, and time-varying data, underscoring the need for more advanced techniques. This study explores a hybrid CNN-LSTM model to enhance temperature forecasting accuracy for the Delhi region, using historical meteorological data from 1996 to 2017. We employed both direct and indirect methods, including comprehensive data preprocessing and exploratory analysis, to construct and train our model. The CNN component effectively extracts spatial features, while the LSTM captures temporal dependencies, leading to improved prediction accuracy. Experimental results indicate that the CNN-LSTM model significantly outperforms traditional forecasting methods in terms of both accuracy and stability, with a mean square error (MSE) of 3.26217 and a root mean square error (RMSE) of 1.80615. The hybrid model demonstrates its potential as a robust tool for temperature prediction, offering valuable insights for meteorological forecasting and related fields. Future research should focus on optimizing model architecture, exploring additional feature extraction techniques, and addressing challenges such as overfitting and computational complexity. This approach not only advances temperature forecasting but also provides a foundation for applying deep learning to other time series forecasting tasks.
Keywords
Time series forecasting, convolutional neural networks (CNN), long short-term memory networks (LSTM)
[1]. E. Kalnay et al., "The NCEP/NCAR 40-Year Reanalysis Project," Bull. Amer. Meteor. Soc., vol. 77, no. 3, pp. 437-471, 1996.
[2]. Y. LeCun, Y. Bengio, and G. Hinton, "Deep learning," Nature, vol. 521, pp. 436-444, 2015.
[3]. S. Hochreiter and J. Schmidhuber, "Long short-term memory," Neural Comput., vol. 9, no. 8, pp. 1735-1780, 1997.
[4]. Y. Qin et al., "A dual-stage attention-based recurrent neural network for time series prediction," in Proc. 26th Int. Joint Conf. Artif. Intell., 2017, pp. 2627-2633.
[5]. F. A. Gers, J. Schmidhuber, and F. Cummins, "Learning to forget: Continual prediction with LSTM," Neural Comput., vol. 12, no. 10, pp. 2451-2471, 2000.
[6]. M. Kiranyaz, T. Ince, and M. Gabbouj, "Real-time patient-specific ECG classification by 1-D convolutional neural networks," IEEE Trans. Biomed. Eng., vol. 63, no. 3, pp. 664-675, Mar. 2016.
[7]. X. Shi et al., "Convolutional LSTM network: A machine learning approach for precipitation nowcasting," in Proc. 29th Conf. Neural Inf. Process. Syst., 2015, pp. 802-810.
[8]. R. Chevallier et al., "Global real-time precipitation forecasting with a neural network approach," in Proc. IEEE Int. Conf. Big Data (Big Data), 2018, pp. 3849-3858.
[9]. A. Ahmed and J. A. S. Alalana, "Temperature prediction using LSTM neural network," in Proc. IEEE 9th International Conference on Electronics (ICEL), 2020, pp. 210-215.
[10]. H. Wang et al., "Temperature forecasting using deep learning methods: A comprehensive review," Energy Reports, vol. 6, pp. 232-245, 2020.
[11]. Y. Zhang et al., "Time series temperature forecasting with convolutional neural networks," in Proc. IEEE International Conference on Data Mining, 2019, pp. 734-739.
Cite this article
Li,B.;Qian,Y. (2024). Weather prediction using CNN-LSTM for time series analysis: A case study on Delhi temperature data. Applied and Computational Engineering,92,121-127.
Data availability
The datasets used and/or analyzed during the current study will be available from the authors upon reasonable request.
Disclaimer/Publisher's Note
The statements, opinions and data contained in all publications are solely those of the individual author(s) and contributor(s) and not of EWA Publishing and/or the editor(s). EWA Publishing and/or the editor(s) disclaim responsibility for any injury to people or property resulting from any ideas, methods, instructions or products referred to in the content.
About volume
Volume title: Proceedings of the 6th International Conference on Computing and Data Science
© 2024 by the author(s). Licensee EWA Publishing, Oxford, UK. This article is an open access article distributed under the terms and
conditions of the Creative Commons Attribution (CC BY) license. Authors who
publish this series agree to the following terms:
1. Authors retain copyright and grant the series right of first publication with the work simultaneously licensed under a Creative Commons
Attribution License that allows others to share the work with an acknowledgment of the work's authorship and initial publication in this
series.
2. Authors are able to enter into separate, additional contractual arrangements for the non-exclusive distribution of the series's published
version of the work (e.g., post it to an institutional repository or publish it in a book), with an acknowledgment of its initial
publication in this series.
3. Authors are permitted and encouraged to post their work online (e.g., in institutional repositories or on their website) prior to and
during the submission process, as it can lead to productive exchanges, as well as earlier and greater citation of published work (See
Open access policy for details).