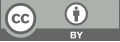
Application of artificial intelligence in construction
- 1 Beibu Gulf University, Guangxi, China
* Author to whom correspondence should be addressed.
Abstract
This paper mainly introduces the application and assistance of artificial intelligence in architecture and construction cost, as well as the future prospects and development trends. The study adopts a quantitative research design of survey research and data collection method of literature analysis. In addition, it can further explore the innovative applications of artificial intelligence in architectural design, such as spatial planning optimization based on big data analysis and simulation experience through virtual reality technology. At the same time, it can also deeply explore the role of artificial intelligence in the automated management of construction processes, material selection optimization, etc., and give detailed explanations with specific cases. In addition, when discussing the future prospects and development trends, it can consider the prediction of the impact of the continuous evolution of artificial intelligence technology on the construction industry, such as the wider application of machine learning algorithms in project management, the gradual maturity of intelligent sensor systems in building equipment monitoring. At the same time, it can also analyze the profound impact of artificial intelligence on the future development of the construction industry from economic, social and environmental angles, and put forward corresponding solutions.
Keywords
AI, RNN, CNN, Engineering Cost, Construction
[1]. Lu Guangyang, Liu Yang. Construction Engineering Construction Technology Innovation and Application [J]. Digital Design, 2018(1).
[2]. Chen Wanjin, He Xuesong. Big Data-Driven Social Work: Prospects and Challenges[J]. Social Sciences, 2017(1).
[3]. Deng Dongmei. Empirical Analysis and Research on the Structural Characteristics of Time-Sequential Networks, 0(2).
[4]. Li Siquan, Zhang Xuanxiong. Research on Facial Expression Recognition Based on Convolutional Neural Networks [J]. Software Guide, 2018(1).
[5]. Wang Lin. Design of Performance Improvement Plan Based on Employee Capability Enhancement [J]. Value Engineering, 2017(1).
[6]. Harvard Business Review. New Growth, New Leadership: The 2015 Davos Forum Magazine (Harvard Business Review), Vol. 4, 2015.
[7]. Qu Song. On Environmental Protection and Sustainable Development [J]. Environment and Development, 2012(1).
[8]. Wang Hao. Analysis and Protection Measures of Network Information Security[J]. Friend of Science: Chinese Edition, 2009(1).
[9]. Jiang Yong, Wang Junqi. On the Current Status and Development of Financial Intelligence [J]. Journal of Suzhou Education Institute, 2009(1).
[10]. Zhou Binbin, Gu Leisheng. Research on the Development Status of IoT Platforms in China[J]. Innovative Science and Technology, 2018(1).
[11]. Ding Yizhong. The Organizational Structure of Customer Credit Management in Shipping Enterprises [J]. Journal of Shanghai Maritime University, 2009(1).
[12]. Li Yuejing. Research on Real-time Motion Target Detection and Tracking System Based on Video, 0(2).
[13]. Apply information and intelligent technologies to enhance project management for smart engineering construction.
[14]. Ye Jian. Application of IoT Technology in the Architecture of Intelligent Transportation System [J]. Science and Technology Innovation and Application, 2018(1).
[15]. Chen Jianjun, Yu Zhixiang, Zhu Yun. Data Visualization Technology and Its Applications [J]. Infrared and Laser Engineering, 2001(1).
[16]. Xu Peiyun. A Research on the Method of Identifying Enterprise Risks Based on Financial Statement Analysis, 2008(2).
[17]. Ramesh Babu, A.,Singh, Y.P.,Sachdeva, R.K..Establishing a management information system,1997(1).
[18]. Luo Ruifang. Design and Implementation of a Web-based Warehouse Management System, 2009(2).
Cite this article
Lyu,Y. (2024). Application of artificial intelligence in construction. Applied and Computational Engineering,90,26-34.
Data availability
The datasets used and/or analyzed during the current study will be available from the authors upon reasonable request.
Disclaimer/Publisher's Note
The statements, opinions and data contained in all publications are solely those of the individual author(s) and contributor(s) and not of EWA Publishing and/or the editor(s). EWA Publishing and/or the editor(s) disclaim responsibility for any injury to people or property resulting from any ideas, methods, instructions or products referred to in the content.
About volume
Volume title: Proceedings of the 6th International Conference on Computing and Data Science
© 2024 by the author(s). Licensee EWA Publishing, Oxford, UK. This article is an open access article distributed under the terms and
conditions of the Creative Commons Attribution (CC BY) license. Authors who
publish this series agree to the following terms:
1. Authors retain copyright and grant the series right of first publication with the work simultaneously licensed under a Creative Commons
Attribution License that allows others to share the work with an acknowledgment of the work's authorship and initial publication in this
series.
2. Authors are able to enter into separate, additional contractual arrangements for the non-exclusive distribution of the series's published
version of the work (e.g., post it to an institutional repository or publish it in a book), with an acknowledgment of its initial
publication in this series.
3. Authors are permitted and encouraged to post their work online (e.g., in institutional repositories or on their website) prior to and
during the submission process, as it can lead to productive exchanges, as well as earlier and greater citation of published work (See
Open access policy for details).