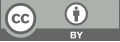
A Strategy for Advertisement Placement based on the Multi-Armed Tiger Problem
- 1 The Pennsylvania State University, 201 Old Main, University Park, PA 16802, USA
* Author to whom correspondence should be addressed.
Abstract
The purpose of this study is to investigate the effectiveness of using Multi armed bandit model, which contains ϵ -greedy, Upper Confidence Bound (UCB), and Thompson sampling algorithms, to optimize online advertisement placement. Through simulating different types of ad placements using different algorithms and comparing them, this paper intends to demonstrate the feasibility of the Multi-Armed Robber model for the ad placement problem. The results show that the multi-armed bandit model can improve the ad click rate compared with the traditional ad placement strategy. Thompson sampling algorithm outperforms the ϵ -greedy algorithm and UCB algorithm in this paper's experiments, which can better balance exploration and application and reduce regret. The algorithm provides a more efficient method of allocating ad resources. These findings provide new insights into the field of digital marketing and may have an impact on the development of actual ad placement strategies.
Keywords
Ad Placement, Multi armed bandit, Thompson Sampling, ϵ -greedy, Upper Confidence Bound
[1]. Geng,T,Lin,X.,&Nair,H.S.(2019).Online evaluation of audiences for targeted advertising via bandit experiments.
[2]. Auer, P.,Cesa-Bianchi, N., & Fischer, P. (2021). A Novel Application of Multi-Armed Bandits to Optimize Display Advertising.
[3]. Hu,H.,Charpentier,A.,Ghossoub,M.,& Schied,A.(2022).The multi-armed bandit problem under the mean-variance setting.
[4]. Auer,P,Cesa-Bianchi,N., & Fischer, P. (2021). Enhancing Online Advertisements Through Contextual Bandit Algorithms.
[5]. Cesa-Bianchi, N., Gentile, C., & Zappella, G. (2020). Adaptive Strategies in Real-Time Bidding Based on Multi-Armed Bandit.
[6]. Cesa-Bianchi, N., Gentile, C., & Zappella, G. (2022). Algorithmic Enhancements in Multi-Armed Bandits for Sophisticated Ad Targeting.
[7]. Jiang, C. (2021). Multi-armed bandits for personalized online advertising.
[8]. Zhou, J. (2024). Application and comparative analysis of adaptive strategies in multi-armed bandit algorithms. Applied and Computational Engineering, 64(1), 237-248.
[9]. Zhou, Z., Srikant, R., Veeravalli, V. V., Milenkovic, O., & Mehta, P. (2021). Impact of Thompson sampling in programmatic ad buying.University of Illinois Urbana-Champaign.
[10]. Jiang, C.,& Shabalina, O. (2022). Using advanced bandit algorithms to optimize ad placement on social media. University of Illinois Urbana-Champaign.
[11]. Kong, S. T. (2020).Multi-armed bandits and machine learning for effective ad campaigns. University of Illinois Urbana-Champaign.
[12]. Jiang,C.(2019).Real-time optimization of online advertisements using MAB models. University of Illinois Urbana-Champaign.
Cite this article
Zhao,Y. (2024). A Strategy for Advertisement Placement based on the Multi-Armed Tiger Problem. Applied and Computational Engineering,83,94-102.
Data availability
The datasets used and/or analyzed during the current study will be available from the authors upon reasonable request.
Disclaimer/Publisher's Note
The statements, opinions and data contained in all publications are solely those of the individual author(s) and contributor(s) and not of EWA Publishing and/or the editor(s). EWA Publishing and/or the editor(s) disclaim responsibility for any injury to people or property resulting from any ideas, methods, instructions or products referred to in the content.
About volume
Volume title: Proceedings of CONF-MLA 2024 Workshop: Semantic Communication Based Complexity Scalable Image Transmission System for Resource Constrained Devices
© 2024 by the author(s). Licensee EWA Publishing, Oxford, UK. This article is an open access article distributed under the terms and
conditions of the Creative Commons Attribution (CC BY) license. Authors who
publish this series agree to the following terms:
1. Authors retain copyright and grant the series right of first publication with the work simultaneously licensed under a Creative Commons
Attribution License that allows others to share the work with an acknowledgment of the work's authorship and initial publication in this
series.
2. Authors are able to enter into separate, additional contractual arrangements for the non-exclusive distribution of the series's published
version of the work (e.g., post it to an institutional repository or publish it in a book), with an acknowledgment of its initial
publication in this series.
3. Authors are permitted and encouraged to post their work online (e.g., in institutional repositories or on their website) prior to and
during the submission process, as it can lead to productive exchanges, as well as earlier and greater citation of published work (See
Open access policy for details).