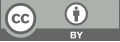
Enhancing stability and explainability in reinforcement learning with machine learning
- 1 Central South University
* Author to whom correspondence should be addressed.
Abstract
In the field of reinforcement learning, training agents using machine learning algorithms to learn and perform tasks in complex environments has become a prevalent approach. However, reinforcement learning faces challenges such as training instability and decision opacity, which limit its feasibility in real-world applications. To solve the problems of stability and transparency in reinforcement learning, this project will use advanced algorithms like Proximal Policy Optimization (PPO), Q-DAGGER, and Gradient Boosting Decision Trees to set up reinforcement learning agents in the OpenAI Gymnasium environment. Specifically, the study selected the Atari game Breakout as the testbed, enhancing training efficiency and game performance by refining reward structures and decision-making processes, and integrating interpretable models to provide explanations for agent decisions. This study has successfully developed robust reinforcement learning agents that excel in complex environments. By employing advanced algorithms like PPO, Q-DAGGER, and Gradient Boosting Decision Trees, the study has addressed issues of training instability, and improved game performance through optimized reward structures and decision processes. Additionally, by integrating interpretable models, the study has provided insights into the learned strategies of the agents, thereby enhancing decision transparency. These findings provide crucial support for the broader application of reinforcement learning in real-world scenarios and offer valuable insights for tackling other complex tasks.
Keywords
Reinforcement learning, Explainable artificial intelligence, Proximal policy gradient, GBDT.
[1]. Reinforcement Learning Stability: Mnih, V., Kavukcuoglu, K., Silver, D., et al. (2015). Human-level control through deep reinforcement learning. Nature, 518(7540), 529-533.
[2]. Machine Learning for Decision Making: Ribeiro, M.T., Singh, S., & Guestrin, C. (2016). "Why should I trust you?": Explaining the predictions of any classifier. In Proceedings of the 22nd ACM SIGKDD international conference on knowledge discovery and data mining (pp. 1135-1144).
[3]. Proximal Policy Optimization (PPO): Schulman, J., Wolski, F., Dhariwal, P., Radford, A., & Klimov, O. (2017). Proximal policy optimization algorithms. arXiv preprint arXiv:1707.06347.
[4]. Q-DAGGER: Ross, S., & Bagnell, D. (2010). Efficient reductions for imitation learning. In Proceedings of the thirteenth international conference on artificial intelligence and statistics (pp. 661-668).
[5]. OpenAI Gymnasium: Brockman, G., Cheung, V., Pettersson, L., Schneider, J., Schulman, J., Tang, J., & Zaremba, W. (2016). OpenAI gym. arXiv preprint arXiv:1606.01540.
[6]. Reinforcement Learning Stability: Mnih, V., Kavukcuoglu, K., Silver, D., et al. (2015). Human-level control through deep reinforcement learning. Nature, 518(7540), 529-533.
[7]. Interpretable Machine Learning Models: Molnar, C. (2020). Interpretable machine learning. Lulu.com.
[8]. Machine Learning for Decision Making: Ribeiro, M.T., Singh, S., & Guestrin, C. (2016). "Why should I trust you?": Explaining the predictions of any classifier. In Proceedings of the 22nd ACM SIGKDD international conference on knowledge discovery and data mining (pp. 1135-1144).
[9]. Computational Demands in AI Research: Jordan, M.I., & Mitchell, T.M. (2015). Machine learning: Trends, perspectives, and prospects. Science, 349(6245), 255-260.
[10]. Visual Methods for Model Interpretability: Zeiler, M.D., & Fergus, R. (2014). Visualizing and understanding convolutional networks. In European conference on computer vision (pp. 818-833).
Cite this article
Chen,Y. (2024). Enhancing stability and explainability in reinforcement learning with machine learning. Applied and Computational Engineering,101,25-34.
Data availability
The datasets used and/or analyzed during the current study will be available from the authors upon reasonable request.
Disclaimer/Publisher's Note
The statements, opinions and data contained in all publications are solely those of the individual author(s) and contributor(s) and not of EWA Publishing and/or the editor(s). EWA Publishing and/or the editor(s) disclaim responsibility for any injury to people or property resulting from any ideas, methods, instructions or products referred to in the content.
About volume
Volume title: Proceedings of the 2nd International Conference on Machine Learning and Automation
© 2024 by the author(s). Licensee EWA Publishing, Oxford, UK. This article is an open access article distributed under the terms and
conditions of the Creative Commons Attribution (CC BY) license. Authors who
publish this series agree to the following terms:
1. Authors retain copyright and grant the series right of first publication with the work simultaneously licensed under a Creative Commons
Attribution License that allows others to share the work with an acknowledgment of the work's authorship and initial publication in this
series.
2. Authors are able to enter into separate, additional contractual arrangements for the non-exclusive distribution of the series's published
version of the work (e.g., post it to an institutional repository or publish it in a book), with an acknowledgment of its initial
publication in this series.
3. Authors are permitted and encouraged to post their work online (e.g., in institutional repositories or on their website) prior to and
during the submission process, as it can lead to productive exchanges, as well as earlier and greater citation of published work (See
Open access policy for details).