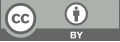
Integrating Deep Learning with Generative Design and Topology Optimization for Efficient Additive Manufacturing
- 1 Meiji University, Tokyo, Japan
* Author to whom correspondence should be addressed.
Abstract
Additive manufacturing (AM) through generative design and topology optimisation creates complex, lightweight structures with exceptional material efficiency and structural integrity. When coupled with deep learning functionality, generative design and topology optimisation can explore broader design spaces and optimise more efficiently, creating novel AM structures that utilise material more efficiently and have better strength and performance than their counterparts created through conventional AM methods. The study tackles how deep learning models such as convolutional neural networks (CNNs) can be integrated into generative design and topology optimisation and how these integration help optimise material usage, production time and performance. Case studies from the aerospace, automotive, and healthcare industries exemplify how these synergies resulted in more resilient, cost-effective designs that would not have been possible through conventional AM approaches. The study focuses on material usage efficiency, reduction in production time and performance improvement to showcase how deep learning integrations enhance the process from design conceptualisation, through iterations, to final production.
Keywords
Generative Design, Topology Optimization, Deep Learning, Additive Manufacturing, Material Efficiency.
[1]. Bucher, Martin Juan José, et al. "Performance-based generative design for parametric modeling of engineering structures using deep conditional generative models." Automation in Construction 156 (2023): 105128.
[2]. Ni, Bo, David L. Kaplan, and Markus J. Buehler. "Generative design of de novo proteins based on secondary-structure constraints using an attention-based diffusion model." Chem 9.7 (2023): 1828-1849.
[3]. Zhu, Bao, et al. "Generative design of texture for sliding surface based on machine learning." Tribology International 179 (2023): 108139.
[4]. Hankins, Sarah N., et al. "Generative design of large-scale fluid flow structures via steady-state diffusion-based dehomogenization." Scientific Reports 13.1 (2023): 14344.
[5]. Nourian, Pirouz, Shervin Azadi, and Robin Oval. "Generative design in architecture: From mathematical optimization to grammatical customization." Computational Design and Digital Manufacturing. Cham: Springer International Publishing, 2023. 1-43.
[6]. Rosen, David W., and Christina Youngmi Choi. "Research issues in the generative design of cyber-physical-human systems." Journal of Computing and Information Science in Engineering 23.6 (2023): 060810.
[7]. Starodubcev, Nikita O., et al. "Generative design of physical objects using modular framework." Engineering Applications of Artificial Intelligence 119 (2023): 105715.
[8]. Momeni Rad, Faeze, Christoph Sydora, and Karim El-Basyouny. "Leveraging generative design and point cloud data to improve conformance to passing lane layout." Sensors 24.2 (2024): 318.
[9]. Klooker, Marie, and Katharina Hölzle. "A generative design of collaborative innovation space." R&D Management 54.2 (2024): 323-346.
[10]. Timperley, Louis, et al. "Towards improving the design space exploration process using generative design with mbse." 2023 IEEE Aerospace Conference. IEEE, 2023.
Cite this article
Huang,L. (2024). Integrating Deep Learning with Generative Design and Topology Optimization for Efficient Additive Manufacturing. Applied and Computational Engineering,116,55-60.
Data availability
The datasets used and/or analyzed during the current study will be available from the authors upon reasonable request.
Disclaimer/Publisher's Note
The statements, opinions and data contained in all publications are solely those of the individual author(s) and contributor(s) and not of EWA Publishing and/or the editor(s). EWA Publishing and/or the editor(s) disclaim responsibility for any injury to people or property resulting from any ideas, methods, instructions or products referred to in the content.
About volume
Volume title: Proceedings of the 5th International Conference on Signal Processing and Machine Learning
© 2024 by the author(s). Licensee EWA Publishing, Oxford, UK. This article is an open access article distributed under the terms and
conditions of the Creative Commons Attribution (CC BY) license. Authors who
publish this series agree to the following terms:
1. Authors retain copyright and grant the series right of first publication with the work simultaneously licensed under a Creative Commons
Attribution License that allows others to share the work with an acknowledgment of the work's authorship and initial publication in this
series.
2. Authors are able to enter into separate, additional contractual arrangements for the non-exclusive distribution of the series's published
version of the work (e.g., post it to an institutional repository or publish it in a book), with an acknowledgment of its initial
publication in this series.
3. Authors are permitted and encouraged to post their work online (e.g., in institutional repositories or on their website) prior to and
during the submission process, as it can lead to productive exchanges, as well as earlier and greater citation of published work (See
Open access policy for details).