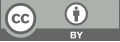
Diabetes Prediction Based on KNN, XGBoost, SVM and LR model
- 1 Department of Computer Science, Hong Kong Polytechnic university, Hong Kong, China
* Author to whom correspondence should be addressed.
Abstract
A. Diabetes mellitus is a chronic metabolic disease characterized by high blood sugar levels due to insulin production problems or insulin resistance. Early identification of diabetes is crucial for preventing associated complications and effectively managing the condition. This study explores the application of four machine learning models, i.e., K-Nearest Neighbor (KNN), XGBoost, Support Vector Machine (SVM) and Logistic Regression (LR) in diabetes prediction. the main goal is to assess and contrast these models' efficacy in identifying diabetes risk, thereby helping healthcare professionals make timely diagnostic and treatment decisions. The results show that the logistic regression model with an AUC value of 0.95 performs much better than the other models, demonstrating excellent sensitivity and specificity in diabetes identification. The XGBoost model also demonstrates considerable predictive accuracy with an AUC value of 0.84, highlighting its ability to effectively handle large-scale datasets. Although the SVM and KNN models had slightly lower AUC values of 0.79, they still provided reliable predictive capabilities. These results demonstrate how machine learning may be used to improve diabetes prediction.
Keywords
Diabetes prediction, KNN, XGBoost, SVM.
[1]. Kumar D A and Govindasamy R 2015 Performance and evaluation of classification data mining techniques in diabetes International Journal of Computer Science and Information Technologies vol 6(2) pp 1312-1319
[2]. Larabi-Marie-Sainte S, Aburahmah L, Almohaini R and Saba T 2019 Current techniques for diabetes prediction: review and case study Applied Sciences vol 9(21) p 4604
[3]. Ahola A J and Groop P H 2013 Barriers to self‐management of diabetes Diabetic medicine vol 30(4) pp 413-420
[4]. Sisodia D 2018 Prediction of diabetes using classification algorithms Procedia computer science vol 132 pp 1578-1585
[5]. Gupta S C and Goel N 2020 Performance enhancement of diabetes prediction by finding optimum K for KNN classifier with feature selection method Third International Conference on Smart Systems and Inventive Technology (ICSSIT) pp 980-986
[6]. Islam M M, Ferdousi R, Rahman S and Bushra H Y 2020 Likelihood prediction of diabetes at early stage using data mining techniques Computer vision and machine intelligence in medical image analysis pp 113-125
[7]. Yu W, Liu T, Valdez R, Gwinn M and Khoury M J 2010 Application of support vector machine modeling for prediction of common diseases: the case of diabetes and pre-diabetes BMC medical informatics and decision making vol 10 pp 1-7
[8]. Azrar A, Ali Y, Awais M and Zaheer K 2018 Data mining models comparison for diabetes prediction Int J Adv Comput Sci Appl vol 9(8) pp 320-323
[9]. Lehmann E L and Scheffé H 2011 Completeness similar regions and unbiased estimation-Part I Selected works of EL Lehmann pp 233-268
[10]. Cover T M 1965 Geometrical and statistical properties of systems of linear inequalities with applications in pattern recognition IEEE transactions on electronic computers vol 3 pp 326-334
[11]. Chen T and Guestrin C 2016 Xgboost: A scalable tree boosting system Proceedings of the 22nd acm sigkdd international conference on knowledge discovery and data mining pp 785-794
[12]. Dong X, Yu Z, Cao W, Shi Y and Ma Q 2020 A survey on ensemble learning Frontiers of Computer Science vol 14 pp 241-258
Cite this article
Yang,S. (2024). Diabetes Prediction Based on KNN, XGBoost, SVM and LR model. Applied and Computational Engineering,104,91-95.
Data availability
The datasets used and/or analyzed during the current study will be available from the authors upon reasonable request.
Disclaimer/Publisher's Note
The statements, opinions and data contained in all publications are solely those of the individual author(s) and contributor(s) and not of EWA Publishing and/or the editor(s). EWA Publishing and/or the editor(s) disclaim responsibility for any injury to people or property resulting from any ideas, methods, instructions or products referred to in the content.
About volume
Volume title: Proceedings of the 2nd International Conference on Machine Learning and Automation
© 2024 by the author(s). Licensee EWA Publishing, Oxford, UK. This article is an open access article distributed under the terms and
conditions of the Creative Commons Attribution (CC BY) license. Authors who
publish this series agree to the following terms:
1. Authors retain copyright and grant the series right of first publication with the work simultaneously licensed under a Creative Commons
Attribution License that allows others to share the work with an acknowledgment of the work's authorship and initial publication in this
series.
2. Authors are able to enter into separate, additional contractual arrangements for the non-exclusive distribution of the series's published
version of the work (e.g., post it to an institutional repository or publish it in a book), with an acknowledgment of its initial
publication in this series.
3. Authors are permitted and encouraged to post their work online (e.g., in institutional repositories or on their website) prior to and
during the submission process, as it can lead to productive exchanges, as well as earlier and greater citation of published work (See
Open access policy for details).