1. Introduction
The swift progress in deep learning has catalyzed a significant shift in how complex data generation tasks are approached, with diffusion models [1,2] emerging as a prominent class of generative models. Diffusion models, inspired by non-equilibrium thermodynamics [3], have gained traction due to their ability to iteratively transform simple noise distributions into complex, high-dimensional data representations. This method has been widely used across different fields, including image synthesis [4], text-to-image generation [5], and molecular design [6], demonstrating its versatility and robustness as well as medical image reconstruction [7]. Given the growing importance of these models, it is crucial to comprehensively review their development, applications, and potential challenges to guide future research and practical implementations.
Nowadays, the evolution of diffusion models has seen substantial improvement aimed at enhancing their efficiency and accuracy. Early methods, primarily based on Markovian processes, utilized Gaussian noise to iteratively perturb data samples. Although effective, these techniques were computationally expensive, hindering scalability. The introduction of innovations such as score matching and denoising diffusion probabilistic models (DDPMs) marked a notable leap forward by refining noise estimation, leading to better performance. Moreover, integrating neural network architectures with diffusion models has enabled the generation of increasingly complex and diverse data types.
Despite these advancements, several challenges persist. Model interpretability remains a critical issue, as the complex processes governing diffusion models are frequently opaque, making it challenging to understand how decisions are made. Training stability is another ongoing concern, as diffusion models can be prone to instability, especially when dealing with large-scale or complex datasets. The high computational demands associated with these models also pose significant challenges, limiting their practical application in resource-constrained environments.
Looking forward, the field is actively investigating solutions to these challenges. Researchers are exploring more efficient training algorithms, improved noise estimation techniques, and better integration with neural network frameworks to enhance model stability and interpretability. Additionally, there is a growing interest in developing hybrid models that combine the strengths of diffusion models with other generative approaches, potentially offering a more balanced trade-off between accuracy, efficiency, and computational resource requirements.
This review targets on offer an in-depth analysis of diffusion models, focusing on their theoretical foundations, technological advancements, as well as diverse applications. The review is structured to systematically explore diffusion models, starting with their origins from early stochastic processes and non-equilibrium thermodynamics to their current status as powerful generative models. The first section examines the historical development of diffusion models, tracing their evolution and highlighting the core techniques employed in their advancement. Key principles, such as the iterative process of noise addition and removal, are explored to understand how simple distributions are transformed into complex data representations. Innovations enhancing the efficiency and effectiveness of diffusion models are discussed, including advancements in score matching, DDPMs and the integration of neural network architectures. A comparative analysis of key technologies is presented, assessing their performance across various tasks and identifying strengths and limitations. The review also addresses ongoing challenges, such as interpretability, training stability, and computational cost, while offers directions for future research. The conclusion summarizes key findings and offers insights into future developments, aiming to guide researchers and practitioners in advancing the field of diffusion models.
2. Methodology
2.1. Dataset description and preprocessing
In the context of diffusion models, various datasets have been extensively used to benchmark and evaluate the performance of these models across different tasks. Some of the most commonly employed datasets include Canadian Institute for Advanced Research (CIFAR)-10, ImageNet and so on, each offering distinct characteristics that cater to specific application areas. For instance, the CIFAR-10 dataset [8] consists of 60,000 32x32 color images in 10 classes, with 6,000 images per class, commonly used for image classification and generation tasks. ImageNet [9], a large-scale visual dataset with over 14 million images categorized into thousands of classes, provides a standard benchmark for high-resolution image generation.
Preprocessing is a crucial step in preparing these datasets for use in diffusion models. For example, images are resized or cropped to a fixed resolution, such as 64*64 or 256*256 pixels. Normalization of pixel values, often to the range [-1, 1] or [0, 1], is also a standard procedure to ensure consistency in input data. Furthermore, various data augmentation methods, including random cropping, flipping, and color jittering, are utilized to enhance the variety and richness of the training data. thereby improving the model’s generalization capabilities. These preprocessing steps are essential for optimizing the datasets for diffusion model training, ensuring that the models can accurately capture the fundamental data distribution and perform well across various generative tasks.
2.2. Proposed approach
This research aims to explore diffusion models in image generation by comparing various methods. Author’s approach begins with a background introduction to the key technologies and their core ideas. Author then analyzes basic models, highlighting their strengths and limitations. The process also has an examination of advanced models including Latent Diffusion Models (LDMs), Denoising Diffusion Implicit Models (DDIMs) and control model followed by a comprehensive comparison of results. This will help the author to evaluate the performance, computational efficiency, and potential for future developments in the field. The overall structure ensures a thorough understanding of each model's impact on image generation, leading to informed future prospects (see in Figure 1).
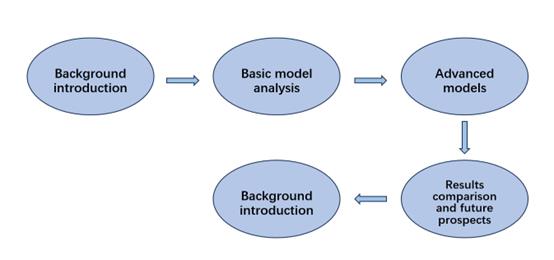
Figure 1. The pipeline of this study.
2.2.1. Introduction of basic techniques. DDPMs introduced by Ho in 2020, represent a significant advancement in generative modeling. These models operate by reversing a forward diffusion process, where data is systematically distorted through the addition of Gaussian noise over several time steps. The forward process is modeled as a Markov chain, with each step adding a small amount of noise, resulting in a progressively noisier and less recognizable version of the original data. The key innovation of DDPMs is their ability to reverse this process. They achieve this by gradually denoising the corrupted data in a step-by-step manner to regenerate the original clean data. The forward diffusion process begins with a data point sampled from the true data distribution. At each time step, added Gaussian noise, produces increasingly noisy data points. This sequence is governed by variance schedules that control the noise levels at each step.
The objective of DDPMs is to learn the reverse denoising process to reconstruct the original data from the noisiest version. This is accomplished by estimating and subtracting the noise added at every steps iteratively. The reverse process is typically modeled by a neural network, often a U-Net or similar architecture, which is trained to estimate the noise at each step using the current noisy data point. DDPMs are renowned for their ability to generate highly diverse and realistic data, particularly in image synthesis. Unlike Generative Adversarial Networks (GANs), DDPMs do not require adversarial training, leading to more stable training processes and fewer issues with mode collapse. This makes DDPMs a powerful and stable alternative for many generative tasks, as shown in the Figure 2.
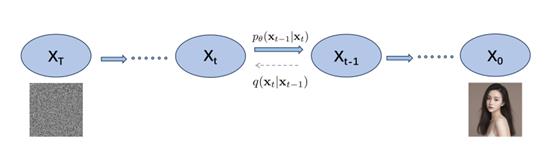
Figure 2. Implementation framework of diffusion model.
2.2.2. LDMs. LDMs [10] are a notable evolution in the diffusion model family, used to reduce the computational complexity while maintaining high-quality output. LDMs function within a reduced-dimensional latent space, rather than working directly within the high-dimensional data space. This method starts by transforming the data into a compressed latent representation. The diffusion process is later applied within this latent space before finally decoding it back to its original dimensionality.
The primary advantage of LDMs is their capability to generate detailed and high-resolution outputs with fewer computational resources. By performing the diffusion process in a compressed space, the model efficiently learns the essential features of the data, which allows for faster training and sampling. This makes LDMs especially effective for tasks such as high-resolution image synthesis and video generation [11].
LDMs provide a scalable and efficient approach to generative modeling, making them a mainstream choice in the field of diffusion models. They offer a balance between computational efficiency and output quality, positioning them as a preferred technique for large-scale generative tasks.
2.2.3. DDIMs. DDIMs [12] are an advanced iteration of diffusion models that optimize the sampling process, making it more efficient while maintaining high output quality. Introduced by Song et al., DDIMs adapt the reverse diffusion process to be deterministic rather than stochastic, which leads to faster and more predictable generation.
In traditional diffusion models like DDPMs, the reverse process involves random sampling, which requires numerous iterations to achieve a high-quality result. DDIMs change this by introducing a deterministic mapping that directly predicts the noise-free data from the noisy intermediate states. This deterministic approach is implemented by adjusting the noise schedule and the denoising step, allowing the model to infer the final image with fewer iterations. Essentially, the model can3ed map noisy data back to its clean form in a more direct and efficient manner.
The key advantage of DDIMs lies in their ability to generate samples in significantly fewer steps without losing image quality. This reduction in computational cost makes DDIMs highly practical for applications that demand rapid generation, such as real-time image synthesis or interactive Artificial Intelligence (AI) systems. Additionally, the deterministic nature of DDIMs ensures more consistent outputs across different runs, which is particularly beneficial in scenarios where reproducibility and precision are important.
2.2.4. Conditional diffusion models. Conditional Diffusion Models [11] represent an advanced approach within the diffusion model framework, particularly tailored for tasks like text-to-image generation. In this method, the generative process is guided by specific inputs, such as text descriptions, to produce outputs that are both high-quality and closely aligned with the given conditions.
The implementation of conditional control in diffusion models, involves integrating text-based conditioning signals at various stages of the diffusion process. This is primarily achieved through the use of cross-attention mechanisms, where the text features influence the denoising process by modulating the intermediate states of the model. The text features are extracted using a pre-trained language model and then embedded into the diffusion process, ensuring that each step of the denoising aligns with the semantic content of the input text.
This conditional setup allows the model to generate images that reflect the detailed nuances of the text, providing more flexibility and control over the generated outputs. The approach also enhances the diversity of the generated images, as the conditioning allows for varied interpretations of the same textual input, which is particularly useful in creative applications. By guiding the generation process with textual descriptions, the model is able to produce images that are not only visually coherent but also semantically relevant to the input conditions.
3. Result and Discussion
In the conducted study, an improved Cycle GAN model was employed with the aim of performing automatic colorization of black-and-white pictures. The model combines a GAN with the cycle consistency loss, enabling high-quality image-to-image translation between black-and-white and colour images. The training dataset consisted of over 1,000 images, which included various categories of black-and-white images paired with corresponding real colour image labels.
3.1. Result
The generated samples demonstrate the effectiveness of DDPMs across various datasets. Figure 3 showcases DDPMs' capability to generate high-quality facial images on the CelebFaces Attributes High Quality (CelebA-HQ) dataset at a resolution of 256 × 256. The faces appear realistic, maintaining diversity across different ethnicities and facial features. Figure 4 illustrates the model's performance on the LSUN Church and Bedroom datasets. The model successfully generates complex structures like churches and detailed indoor scenes, highlighting its ability to capture intricate details in diverse environments.
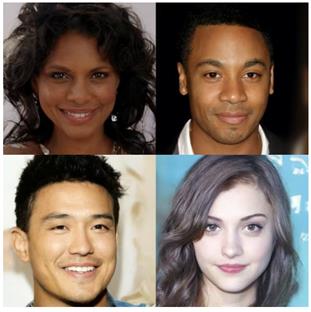
Figure 3. Generated samples of DDPMs on CelebA-HQ 256 × 256 [1].
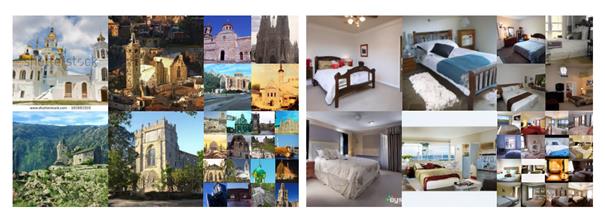
Figure 4. LSUN Church samples and LSUN bedroom samples of DDPMs [1].
Table 1 compares the performance of different diffusion models across various datasets. The table highlights that DDPM achieves relatively low Flame Ionization Detector (FID) scores on Large-Scale Scene Understanding (LSUN) datasets (7.89 for Church and 4.90 for Bedroom), indicating high-quality image generation, though it requires substantial computational resources. In contrast, LDM exhibits lower FID scores (e.g., 2.95 on LSUN Bedroom), showcasing its suitability for high-resolution image generation with reduced computational complexity. However, LDM does show slight distortions in some high-resolution images. DDIM offers faster sampling and consistent generation, yet it has higher FID scores on datasets like CIFAR10 (13.36), demonstrating a trade-off between speed and image quality.
These data suggests that while DDPM excels in quality, its resource demands make it less efficient. LDM strikes a balance between quality and efficiency, particularly in high-resolution tasks, while DDIM prioritizes speed at the expense of quality in more complex datasets.
Table 1. Comparison of different models’ performance on each dataset [1,10-12].
Model | Dataset | FID score | Pre | Recall | Feature description |
DDPM | LSUN Church | 7.89 | - | - | High-quality image generation but High computational resource consumption |
LSUN Bedroom | 4.90 | - | - | ||
LDM | LSUN Church | 4.02 | 0.64 | 0.52 | Lower computational complexity, suitable for high-resolution generation, but small distortions in high-resolution images |
LSUN Bedroom | 2.95 | 0.66 | 0.48 | ||
CelebA-HQ | 5.11 | 0.72 | 0.49 | ||
FFHQ | 4.98 | 0.73 | 0..50 | ||
DDIM | CIFAR10 | 13.36 | - | - | Faster sampling, more consistent generation, but Higher FID scores on some datasets |
CelebA-HQ | 3.51 | - | - |
3.2. Discussion
The analysis of DDPM, LDM, and DDIM underscores their distinct strengths and areas for improvement. DDPM is distinguished by its capability of generating high-quality, realistic images across diverse datasets, such as CelebA-HQ, but this comes at the cost of substantial computational resources. Its performance on datasets like LSUN Church and Bedroom highlights its proficiency in capturing intricate details, yet the high computational demand poses challenges for scalability and efficiency in practical applications.
By contrast, LDM offers a more balanced approach. It achieves lower FID scores, indicating superior image quality with less computational complexity, making it suitable for high-resolution image synthesis. However, occasional distortions in the generated images suggest that there is still room for refinement, particularly in handling high-resolution details. LDM’s versatility makes it a promising candidate for tasks that require a balance between quality and efficiency, such as content generation and high-resolution image editing.
DDIM stands out for its speed in sampling, offering a faster generation process compared to DDPM and LDM. This makes it advantageous in time-sensitive applications. However, the trade-off is evident in the higher FID scores observed on complex datasets like CIFAR10, where the emphasis on speed slightly diminishes image quality. Future work could explore optimizing DDIM to maintain fast sampling while enhancing image fidelity.
In terms of future research directions, optimizing the computational efficiency of DDPM without compromising image quality remains a key challenge. Hybrid models that integrate the strengths of DDPM, LDM, and DDIM could be a potential avenue, leveraging the high quality of DDPM, the efficiency of LDM, and the speed of DDIM. Additionally, exploring adaptive architectures that adjust computational complexity based on the task requirements could further enhance the applicability of these models in various domains, such as real-time video generation, large-scale content creation, and interactive AI systems.
Current challenges also include addressing the inherent trade-offs between speed, quality, and computational demands. Innovative solutions, such as incorporating more advanced network architectures, leveraging transfer learning, or combining these models with other generative approaches like GANs, could help mitigate these issues. Furthermore, improving the robustness of these models in handling diverse and complex datasets will be crucial for their broader application in fields ranging from entertainment and media to scientific simulations and medical imaging.
4. Conclusion
This study presents an innovative approach to improving image generation quality while optimizing computational efficiency by exploring DDPM, LDM, and DDIM in high-resolution tasks. The study systematically analysis and compares these models across various datasets, evaluating their performance in generating high-quality images while balancing speed and resource consumption. Key factors assessed include image quality, computational demands, and sampling efficiency. The experiments reveal that DDPM excels in generating high-quality images but demands substantial computational resources. In contrast, LDM strikes a balance with lower FID scores and moderate resource usage. DDIM, while offering faster sampling, shows slightly higher FID scores, especially on complex datasets. Future research will aim at enhancing the computational efficiency of these models. The goal is to develop hybrid models that integrate the strengths of DDPM, LDM, and DDIM, aiming to maintain high image quality while improving speed and reducing resource requirements. Further investigation will also assess the adaptability of these models for real-time applications and their robustness across a broader range of diverse and complex datasets.
References
[1]. Ho J Jain A Abbeel P 2020 Denoising diffusion probabilistic models Advances in neural information processing systems vol 33 pp 6840-6851
[2]. Jascha S D Weiss E Maheswaranathan N and Ganguli S 2015 Deep unsupervised learning using nonequilibrium thermodynamics In International Conference on Machine Learning pp 2256–2265
[3]. De Groot S R Mazur P 2013 Non-equilibrium thermodynamics Courier Corporation
[4]. Song Y Ermon S 2019 Generative modeling by estimating gradients of the data distribution Advances in neural information processing systems vol 32
[5]. Austin J Johnson D D Ho J et al. 2021 Structured denoising diffusion models in discrete state-spaces Advances in Neural Information Processing Systems vol 34 pp 17981-17993
[6]. Weiss T Mayo Yanes E Chakraborty S et al. 2023 Guided diffusion for inverse molecular design Nature Computational Science vol 3 no 10 pp 873-882
[7]. Cao C Cui Z X Wang Y et al. 2024 High-frequency space diffusion model for accelerated mri IEEE Transactions on Medical Imaging
[8]. Kingma D et al. 2021 Variational diffusion models Advances in neural information processing systems vol 34 pp 21696-21707
[9]. Dhariwal P and Alexander N 2021 Diffusion models beat gans on image synthesis Advances in neural information processing systems vol 34 pp 8780-8794
[10]. Zhang L Anyi R and Maneesh A 2023 Adding conditional control to text-to-image diffusion models Proceedings of the IEEE/CVF International Conference on Computer Vision pp 3836-3847
[11]. Rombach R et al. 2022 High-resolution image synthesis with latent diffusion models Proceedings of the IEEE/CVF conference on computer vision and pattern recognition pp 10684-10695
[12]. Song J Meng C Ermon S 2020 Denoising diffusion implicit models arxiv preprint 2010.02502
Cite this article
Jin,Z. (2024). Advancements in Diffusion Models for Image Generation: A Comparative Analysis of DDPM, LDM, and DDIM. Applied and Computational Engineering,104,96-103.
Data availability
The datasets used and/or analyzed during the current study will be available from the authors upon reasonable request.
Disclaimer/Publisher's Note
The statements, opinions and data contained in all publications are solely those of the individual author(s) and contributor(s) and not of EWA Publishing and/or the editor(s). EWA Publishing and/or the editor(s) disclaim responsibility for any injury to people or property resulting from any ideas, methods, instructions or products referred to in the content.
About volume
Volume title: Proceedings of the 2nd International Conference on Machine Learning and Automation
© 2024 by the author(s). Licensee EWA Publishing, Oxford, UK. This article is an open access article distributed under the terms and
conditions of the Creative Commons Attribution (CC BY) license. Authors who
publish this series agree to the following terms:
1. Authors retain copyright and grant the series right of first publication with the work simultaneously licensed under a Creative Commons
Attribution License that allows others to share the work with an acknowledgment of the work's authorship and initial publication in this
series.
2. Authors are able to enter into separate, additional contractual arrangements for the non-exclusive distribution of the series's published
version of the work (e.g., post it to an institutional repository or publish it in a book), with an acknowledgment of its initial
publication in this series.
3. Authors are permitted and encouraged to post their work online (e.g., in institutional repositories or on their website) prior to and
during the submission process, as it can lead to productive exchanges, as well as earlier and greater citation of published work (See
Open access policy for details).
References
[1]. Ho J Jain A Abbeel P 2020 Denoising diffusion probabilistic models Advances in neural information processing systems vol 33 pp 6840-6851
[2]. Jascha S D Weiss E Maheswaranathan N and Ganguli S 2015 Deep unsupervised learning using nonequilibrium thermodynamics In International Conference on Machine Learning pp 2256–2265
[3]. De Groot S R Mazur P 2013 Non-equilibrium thermodynamics Courier Corporation
[4]. Song Y Ermon S 2019 Generative modeling by estimating gradients of the data distribution Advances in neural information processing systems vol 32
[5]. Austin J Johnson D D Ho J et al. 2021 Structured denoising diffusion models in discrete state-spaces Advances in Neural Information Processing Systems vol 34 pp 17981-17993
[6]. Weiss T Mayo Yanes E Chakraborty S et al. 2023 Guided diffusion for inverse molecular design Nature Computational Science vol 3 no 10 pp 873-882
[7]. Cao C Cui Z X Wang Y et al. 2024 High-frequency space diffusion model for accelerated mri IEEE Transactions on Medical Imaging
[8]. Kingma D et al. 2021 Variational diffusion models Advances in neural information processing systems vol 34 pp 21696-21707
[9]. Dhariwal P and Alexander N 2021 Diffusion models beat gans on image synthesis Advances in neural information processing systems vol 34 pp 8780-8794
[10]. Zhang L Anyi R and Maneesh A 2023 Adding conditional control to text-to-image diffusion models Proceedings of the IEEE/CVF International Conference on Computer Vision pp 3836-3847
[11]. Rombach R et al. 2022 High-resolution image synthesis with latent diffusion models Proceedings of the IEEE/CVF conference on computer vision and pattern recognition pp 10684-10695
[12]. Song J Meng C Ermon S 2020 Denoising diffusion implicit models arxiv preprint 2010.02502