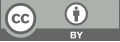
An Empirical Study of WGAN and WGAN-GP for Enhanced Image Generation
- 1 College of Liberal Arts & Sciences, School University of Illinois Urbana Champaign, Champaign–Urbana, Illinois, 61820, United States
* Author to whom correspondence should be addressed.
Abstract
This paper aims to advance the Wasserstein Generative Adversarial Networks (WGANs) and their enhancements, particularly focusing on the gradient penalty. Generative Adversarial Networks (GANs), introduced by Goodfellow et al. in 2014, have revolutionized the domain of image generation. To address the limitations of GANs, the WGAN was proposed. However, WGANs rely on weight clipping, which introduces its own set of issues such as slow convergence and potential gradient vanishing. The inefficiency and instability of WGANs have troubled its users. To solve these problems, WGAN with Gradient Penalty (WGAN-GP) was developed to address these challenges. It provides more stable gradients and reduces the risk of mode collapse by using a gradient penalty to enforce the necessary constraints. In this paper, the author implemented both WGAN and WGAN with Gradient Penalty (WGAN-GP) and evaluated them using the CIFAR-10 and MNIST datasets. The results show that WGAN-GP's outputs are more stable and efficient in the early rounds, confirming the effectiveness of the gradient penalty in training image datasets.
Keywords
Generative adversarial networks, Wasserstein GAN, Image generation
[1]. Goodfellow, I., Pouget-Abadie, J., Mirza, M., Xu, B., Warde-Farley, D., et, al. (2014). Generative adversarial nets. Advances in neural information processing systems, 27, 2672-2680.
[2]. Creswell, A., White, T., Dumoulin, V., Arulkumaran, K., Sengupta, B., & Bharath, A. A. (2018). Generative adversarial networks: An overview. IEEE signal processing magazine, 35(1), 53-65.
[3]. Gui, J., Sun, Z., Wen, Y., Tao, D., & Ye, J. (2021). A review on generative adversarial networks: Algorithms, theory, and applications. IEEE transactions on knowledge and data engineering, 35(4), 3313-3332.
[4]. Wang, K., Gou, C., Duan, Y., Lin, Y., Zheng, X., & Wang, F. Y. (2017). Generative adversarial networks: introduction and outlook. IEEE/CAA Journal of Automatica Sinica, 4(4), 588-598.
[5]. Arjovsky, M., Chintala, S., & Bottou, L. (2017). Wasserstein GAN. arXiv preprint arXiv:1701.07875.
[6]. Gulrajani, I., Ahmed, F., Arjovsky, M., Dumoulin, V., & Courville, A. (2017). Improved training of Wasserstein GANs. arXiv preprint arXiv:1704.00028.
[7]. Saxena, D., & Cao, J. (2021). Generative adversarial networks (GANs) challenges, solutions, and future directions. ACM Computing Surveys, 54(3), 1-42.
[8]. Pan, Z., Yu, W., Yi, X., Khan, A., Yuan, F., & Zheng, Y. (2019). Recent progress on generative adversarial networks (GANs): A survey. IEEE access, 7, 36322-36333.
[9]. The CIFAR-10 dataset. URL: https://www.cs.toronto.edu/~kriz/cifar.html. Last Accessed: 2024/08/26
[10]. Deng, L. (2012). The mnist database of handwritten digit images for machine learning research. IEEE signal processing magazine, 29(6), 141-142.
Cite this article
Lu,L. (2024). An Empirical Study of WGAN and WGAN-GP for Enhanced Image Generation. Applied and Computational Engineering,83,103-109.
Data availability
The datasets used and/or analyzed during the current study will be available from the authors upon reasonable request.
Disclaimer/Publisher's Note
The statements, opinions and data contained in all publications are solely those of the individual author(s) and contributor(s) and not of EWA Publishing and/or the editor(s). EWA Publishing and/or the editor(s) disclaim responsibility for any injury to people or property resulting from any ideas, methods, instructions or products referred to in the content.
About volume
Volume title: Proceedings of CONF-MLA 2024 Workshop: Semantic Communication Based Complexity Scalable Image Transmission System for Resource Constrained Devices
© 2024 by the author(s). Licensee EWA Publishing, Oxford, UK. This article is an open access article distributed under the terms and
conditions of the Creative Commons Attribution (CC BY) license. Authors who
publish this series agree to the following terms:
1. Authors retain copyright and grant the series right of first publication with the work simultaneously licensed under a Creative Commons
Attribution License that allows others to share the work with an acknowledgment of the work's authorship and initial publication in this
series.
2. Authors are able to enter into separate, additional contractual arrangements for the non-exclusive distribution of the series's published
version of the work (e.g., post it to an institutional repository or publish it in a book), with an acknowledgment of its initial
publication in this series.
3. Authors are permitted and encouraged to post their work online (e.g., in institutional repositories or on their website) prior to and
during the submission process, as it can lead to productive exchanges, as well as earlier and greater citation of published work (See
Open access policy for details).