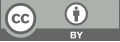
Comparative Analysis of Value Iteration and Policy Iteration in Robotic Decision-Making
- 1 University College London, 25 Gordon Street, London WC1H 0AY, UK
* Author to whom correspondence should be addressed.
Abstract
This study focuses on Markov Decision Processes (MDPs) as a framework for decision-making in robotics. MDPs are crucial for enabling autonomous systems to operate in dynamic environments. MDPs enable the development of optimal strategies that balance immediate and future rewards, making them essential for intelligent robotic behavior. The purpose of this study is to evaluate the performance differences of value iteration and strategy iteration algorithms in different robot tasks. The study highlights the strengths and weaknesses of each algorithm. This comparative analysis uniquely addresses a gap in existing research by evaluating both Value Iteration and Policy Iteration side by side, offering critical insights into their respective performances across diverse robotic tasks. Results indicate that Policy Iteration converges faster and adapts better in complex environments, making it ideal for real-time applications, while Value Iteration is more efficient in smaller state spaces.These findings provide critical insights for selecting algorithms tailored to specific robotic applications, highlighting their role in advancing intelligent robotic systems.
Keywords
Markov Decision Process, Value Iteration, Policy Iteration
[1]. Puterman M L. Markov decision processes: discrete stochastic dynamic programming[M]. John Wiley & Sons, 2014.
[2]. Kaelbling L P, Lozano-Pérez T. Integrated task and motion planning in belief space[J]. The International Journal of Robotics Research, 2013, 32(9-10): 1194-1227.
[3]. Sutton R S, Barto A G. Reinforcement learning: An introduction[M]. MIT press, 2018.
[4]. Lee J, Sutton R S. Policy iterations for reinforcement learning problems in continuous time and space—Fundamental theory and methods[J]. Automatica, 2021, 126: 109421.
[5]. Dong B, Jiang H, Cui Y, et al. Value Iteration-Based Adaptive Fuzzy Backstepping Optimal Control of Modular Robot Manipulators via Integral Reinforcement Learning[J]. International Journal of Fuzzy Systems, 2024: 1-17.
[6]. Geist M, Scherrer B, Pietquin O. A theory of regularized markov decision processes[C]//International Conference on Machine Learning. PMLR, 2019: 2160-2169.
[7]. Bertsekas D. Reinforcement learning and optimal control[M]. Athena Scientific, 2019.
[8]. Lauri M, Hsu D, Pajarinen J. Partially observable markov decision processes in robotics: A survey[J]. IEEE Transactions on Robotics, 2022, 39(1): 21-40.
[9]. Bertsekas D. Abstract dynamic programming[M]. Athena Scientific, 2022.
[10]. Silver D, Huang A, Maddison C J, et al. Mastering the game of Go with deep neural networks and tree search[J]. nature, 2016, 529(7587): 484-489.
[11]. Mnih V, Kavukcuoglu K, Silver D, et al. Human-level control through deep reinforcement learning[J]. nature, 2015, 518(7540): 529-533.
[12]. Ye F, Zhang S, Wang P, et al. A survey of deep reinforcement learning algorithms for motion planning and control of autonomous vehicles[C]//2021 IEEE Intelligent Vehicles Symposium (IV). IEEE, 2021: 1073-1080.
Cite this article
Qiu,Y. (2024). Comparative Analysis of Value Iteration and Policy Iteration in Robotic Decision-Making. Applied and Computational Engineering,83,140-147.
Data availability
The datasets used and/or analyzed during the current study will be available from the authors upon reasonable request.
Disclaimer/Publisher's Note
The statements, opinions and data contained in all publications are solely those of the individual author(s) and contributor(s) and not of EWA Publishing and/or the editor(s). EWA Publishing and/or the editor(s) disclaim responsibility for any injury to people or property resulting from any ideas, methods, instructions or products referred to in the content.
About volume
Volume title: Proceedings of CONF-MLA 2024 Workshop: Semantic Communication Based Complexity Scalable Image Transmission System for Resource Constrained Devices
© 2024 by the author(s). Licensee EWA Publishing, Oxford, UK. This article is an open access article distributed under the terms and
conditions of the Creative Commons Attribution (CC BY) license. Authors who
publish this series agree to the following terms:
1. Authors retain copyright and grant the series right of first publication with the work simultaneously licensed under a Creative Commons
Attribution License that allows others to share the work with an acknowledgment of the work's authorship and initial publication in this
series.
2. Authors are able to enter into separate, additional contractual arrangements for the non-exclusive distribution of the series's published
version of the work (e.g., post it to an institutional repository or publish it in a book), with an acknowledgment of its initial
publication in this series.
3. Authors are permitted and encouraged to post their work online (e.g., in institutional repositories or on their website) prior to and
during the submission process, as it can lead to productive exchanges, as well as earlier and greater citation of published work (See
Open access policy for details).