1. Introduction
Sheet music recognition is vital in music education, digital archiving, and composition. It converts physical sheet music into digital formats, facilitating storage, editing, and sharing [1]. This technology also accelerates music learning, helping musicians and students to understand and perform musical pieces more efficiently, thereby advancing music research and development. That is why developing a tool to accomplish sheet music recognition is meaningful [2].
During the research, it is found that compared to traditional models, deep learning models have the following advantages [3,4]. (1) Fast Processing: Deep learning models can quickly process large volumes of sheet music images, making them ideal for real-time applications where speed is critical. (2) Adaptability to Complex Environments: Deep learning models excel in handling diverse and complex visual environments, including variations in fonts, note sizes, colors, and background interference in sheet music. (3) High Accuracy: These models provide precise recognition of musical symbols and rhythms, even in cases involving multiple notes and intricate patterns, ensuring accurate results. (4) Strong Robustness: Deep learning models maintain stable performance under various conditions, such as changes in resolution, lighting, and image quality, making them reliable across different scenarios. Before using You Only Look Once (YOLO) models, the most widely used model is Optical Music Recognition (OMR). Although OMR has been made significant progress, as it can convert music sheet into number format exactly, it still has some limitations. To be specific, the most advanced OMR system need multiple steps to generate the content of sheet music, which results in the complex of implementing production-ready models. Beyond that, it is limited to monophonic transcription, while ignores more complicated sheet music like counterpoint [5]. In contrast, the YOLO model is known for its ability to perform object detection in a single step. YOLO's primary advantage lies in its efficiency: it processes an entire image in one forward pass through the network, detecting multiple objects simultaneously. This "single-shot" detection significantly reduces the processing time and simplifies the model's architecture. In the context of OMR, if a YOLO-based model could be effectively trained to recognize musical symbols in one pass, it would eliminate the need for the sequential steps typically required by traditional OMR systems. This would result in a more streamlined, faster, and potentially more robust system for real-time applications.
To summarize, the approach of using individual musical notes as primary training data was implemented to enhance the YOLO model's ability in recognizing and selecting musical notes more efficiently, thereby improving the model's performance in sheet music recognition.
2. Method
2.1. Dataset and preprocessing
Since the OMR model does not require additional data training, the datasets and preprocessing are primarily intended for the YOLO model. In this research, five distinct types of musical notes were selected for the dataset: whole notes, half notes, quarter notes, eighth notes, and sixteenth notes. Each category was represented by 1000 carefully chosen images, ensuring a balanced representation across all classes. After image selection, an annotation process was carried out, where each image was labeled with five essential parameters: the note class, the x and y coordinates of the bounding box center, and the bounding box’s width and height. These annotations were formatted according to the YOLO model requirements, ensuring they were suitable for accurate training. The preprocessing steps were designed to standardize the data, thereby enhancing the model’s ability to generalize across different note types.
2.2. Model architecture
Two models are leveraged for the recognition of music notes.
2.2.1. The first model. OMR Utilizing OpenCV. Its workflow can be mainly divided into 5 parts. (1) Image preprocessing: First, the musical score image undergoes preprocessing steps, including grayscale conversion, binarization, noise removal, and skew correction. These steps aim to enhance the image quality, separating symbols from the background to simplify subsequent processing. (2) Staff Line Detection: Using techniques like Hough Trans to detect the position of staff notation, thereby ensures the basic structure of sheet music, which is the fundamental step to identify musical notes. (3) Symbol detection and segmentation: Detecting and segmenting the musical notes within sheet music through contour detection or template matching technology. Every note detected will be notated and extracted from the image. (4) Symbol classification: Using classification algorithm like template matching and Support Vector Machine (SVM) to classify the detected notes so that identify the specific musical notes. Building on this workflow, the OMR model exhibits several distinctive characteristics. 1. Based on traditional image processing techniques: Using image processing tools provided by OpenCV to analyze images, which depends on image features to perform symbol detection and classification. 2. High flexibility: Costuming and adjusting the process flow according to specific demand. 3. Modular implementation: Each step can be developed and optimized separately, which is easy to debug and improve performance. For its advantages, OMR model uses less resource-intensive attention model than the Transformer architecture, it decreases the demand of computing resource so that the model has the ability to work efficiently in general hardware. While it presents significant advantages in practicality, as it supports various programming language and is easy to understand. So, it can serve as a baseline for further development and improvements [6].
2.2.2. The second model. YOLO model is an object detection algorithm based on deep learning. Its major workflow is as the following 5 parts. (1) Image Input: The input image is divided into multiple grids (e.g., 13x13 or 19x19), with each grid responsible for detecting objects within it. (2) Feature Extraction: A Convolutional Neural Network (CNN) extracts features from the image, representing various patterns and information. (3) Bounding Box Prediction: For each grid, YOLO predicts multiple bounding boxes and their corresponding confidence scores. These predictions include the bounding box coordinates (center x, y, width, height) and the class probabilities. (4) Non-Maximum Suppression (NMS): YOLO generates a large number of candidate boxes. NMS is used to filter out overlapping and low-confidence boxes, leaving the best predictions. (5) Object Classification and Localization: The model outputs a set of bounding boxes with the highest confidence and their corresponding class labels, completing the object detection task. YOLO model is a new object detection method. Unlike traditional methods that treat detection tasks as classification problems, YOLO views them as regression problems. Predicting bounds and probability of category directly from complete image through single neural network, which makes YOLO is capable of processing images at extremely fast speeds [7]. Compared to traditional CNN models like Faster R-CNN, YOLO model is better at detecting large targets and making fewer errors in background detection. YOLO models have Less demand for resources, resulting in lower costs [8].
2.3. Evaluating indicator
When evaluating object detection models, precision and mean Average Precision (mAP) are commonly used metrics. Precision represents the ratio of correctly predicted objects to the total predictions, while mAP provides an overall performance measure by considering precision and recall across various confidence thresholds.
Model performance is primarily assessed by inference speed and parameter count. Inference speed indicates the efficiency of the model in real-time applications, often measured in frames per second (FPS). The parameter count refers to the total number of trainable parameters in the model, with fewer parameters typically leading to faster inference and lower computational requirements [9].
3. Result and Discussion
As OMR model majorly depends on OpenCV to perform image processing, it does not involve hyper-parameters such as learning-rate, loss-function. For YOLO models, four major parameters are considered, learning-rate, momentum, scale and epoch. Both YOLOv5 and YOLOv8 have the same parameters through train, as using the datasets ahead and with epochs=50, image-size=640, batch=16.
The accuracy metric is calculated either by dividing the number of recognized notes by the number of correct notes, or by dividing the number of correct notes by the number of recognized notes, depending on which is greater.
According to Table 1, it is obvious to see that YOLOv8 has the highest accuracy rate. While for 100 epochs training, YOLOv5’s performance is unsatisfactory. For OMR model, some details can be further explored.
Table 1. Result comparison of different models.
|
OMR |
YOLOv5 |
YOLOv8 |
accuracy |
0.39 |
0.17 |
0.92 |
parameter/templates |
15 |
7225885 |
3157200 |
time |
117.26s |
0.0441s |
0.8412s |
As demonstrated in Table 2, OMR model identify whole note and half note with 100% accuracy, while it does not perform well. Based on the output results, it could be explained clearly.
As Figure 1, OMR model only pays attention to the note head, while these parts for eight notes and quarter notes are the same. That is the reason why OMR model expresses low accuracy in identifying these two types of notes. While due to its pixel-level segmentation and the additional step of detecting a parallel staff to infer the pitch of the recognized symbols, the OMR model, even if it cannot distinguish between these two types of notes, can still differentiate them in the final output through a corresponding compensation mechanism [10].
Table 2. Accuracy on recognizing different notes.
|
whole |
half |
quarter |
eight |
OMR |
100% |
100% |
44% |
0% |
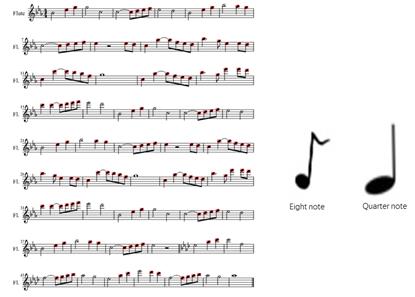
Figure 1. Representative recognized musical notes using OMR model (Figure Credits: Original).
Compared to the OMR model, the YOLO model clearly has more parameters (as the OMR model does not involve deep learning neural networks) and faster computation speed (since it completes the recognition of the entire image in a single pass)
Figure 2 shows the recognition results of the YOLOv8 model on the same image. From the figure, it can be observed that the model identifies all recognized notes at once, marking their categories and confidence scores above each note. Although it missed certain notes (such as whole notes), considering its high computational speed, this issue can be addressed in subsequent optimizations.
Based on existing research, it can be concluded that the YOLOv8 model demonstrates good performance in note detection for sheet music recognition. Although traditional OMR models may struggle to distinguish between quarter notes and eighth notes due to challenges in recognizing note heads, they can still achieve high accuracy at the output stage through their specialization in sheet music recognition. By characterizing elements such as notes, staves, and clefs, OMR models are able to accurately convert sheet music into audio output.
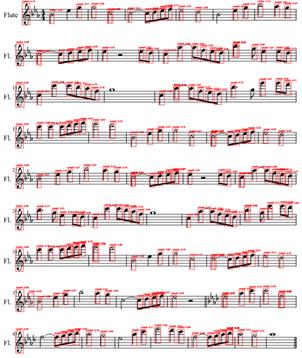
Figure 2. Visualization results of YOLOv8 model (Figure Credits: Original).
In addition, when considering computational speed and device performance, the YOLO model consumes less time during final detection. However, due to the YOLO model's generalization capabilities, it requires more extensive training to achieve accurate results in the specialized field of sheet music recognition.
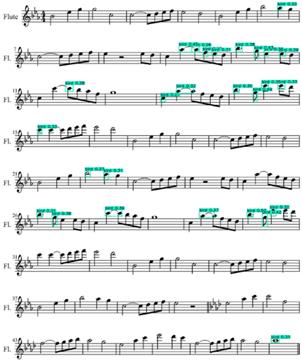
Figure 3. Visualization results of YOLOv5 model (Figure Credits: Original).
Figure 3 presents the results of directly using the YOLOv5 model to predict sheet music. As can be seen, without training on a note-specific dataset, the YOLOv5 model only makes predictions based on common detection outcomes.
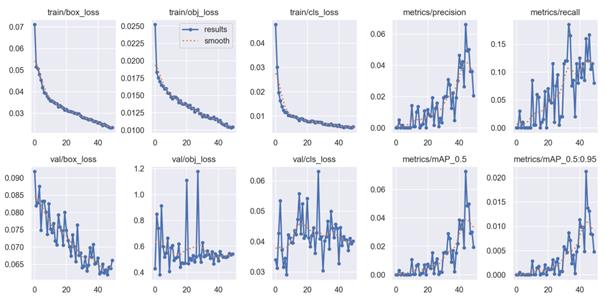
Figure 4. Training process of YOLOv5 model (Figure Credits: Original).
Figure 4 illustrates the training process of the YOLOv5 model. It can be observed that during the 50 epochs of training, the loss value decreases continuously while the metric values increase, although the accuracy remains relatively low. Overall, this indicates that more data and extended training are required to improve the model's performance.
To summarize, there are several limitations to this experiment, such as the insufficient size of the dataset and the constraints imposed by device performance, which prevented larger-scale training required to develop a YOLO model capable of perfectly recognizing all sheet music information. Additionally, the current training process did not yield a model with optimal accuracy, highlighting the need for more robust datasets and extended training iterations.
As YOLO models continue to evolve and further optimization efforts are made, particularly in the specialized field of sheet music recognition, it can be anticipated that significant advancements in both model accuracy and efficiency. Future research and development will likely lead to more refined YOLO models that can be effectively applied to practical scenarios in music transcription and analysis. Consequently, with further investment and exploration, YOLO models are expected to become a key tool in automated sheet music recognition systems.
4. Conclusion
This research explores the performance of three different object detection models in sheet music recognition. For YOLO models, it is necessary to select the dataset, train the model iteratively using the dataset, and finally obtain the results. For the OMR model, simply inputting the notes to be detected yields the output. In terms of experimental results, YOLOv8 strikes a balance between fast processing speed and high accuracy, while the OMR model provides comprehensive recognition of various components within the music score.
In conclusion, if computation time is not a priority and the goal is to obtain a complete and detailed output of sheet music information, the OMR model remains the most suitable option. On the other hand, if the focus is on obtaining results with extreme speed, YOLOv8 is the better choice, and its accuracy can be further improved through additional training.
Looking ahead, with advancements in model architectures and the expansion of annotated datasets, the potential for further refining YOLO models for specialized tasks such as music score recognition is promising. Moreover, hybrid approaches that combine the strengths of both YOLO and OMR models could be explored, potentially leading to more efficient and accurate solutions. These developments will contribute to the continued evolution of automated music recognition systems, enhancing their applicability in both academic and practical contexts.
References
[1]. Shatri, E., & Fazekas, G. (2020). Optical music recognition: State of the art and major challenges. arXiv preprint arXiv:2006.07885.
[2]. Calvo-Zaragoza, J., Jr, J. H., & Pacha, A. (2020). Understanding optical music recognition. ACM Computing Surveys, 53(4), 1-35.
[3]. LeCun, Y., Bengio, Y., & Hinton, G. (2015). Deep learning. nature, 521(7553), 436-444.
[4]. Guo, Y., Liu, Y., Oerlemans, A., Lao, S., Wu, S., & Lew, M. S. (2016). Deep learning for visual understanding: A review. Neurocomputing, 187, 27-48.
[5]. Ríos-Vila, A., Calvo-Zaragoza, J., Rizo, D., & Paquet, T. (2024). Sheet Music Transformer++: End-to-End Full-Page Optical Music Recognition for Pianoform Sheet Music. arXiv preprint arXiv:2405.12105.
[6]. Mayer, J., Straka, M., & Pecina, P. (2024). Practical End-to-End Optical Music Recognition for Pianoform Music. arXiv preprint arXiv:2403.13763.
[7]. Redmon, J., Divvala, S., Girshick, R., & Farhadi, A. (2016). You only look once: Unified, real-time object detection. In Proceedings of the IEEE conference on computer vision and pattern recognition, 779-788.
[8]. Vijayakumar, A., & Vairavasundaram, S. (2024). Yolo-based object detection models: A review and its applications. Multimedia Tools and Applications, 1-40.
[9]. Sapkota, R., Qureshi, R., Calero, M. F., Hussain, M., Badjugar, C., Nepal, U., ... & Karkee, M. (2024). Yolov10 to its genesis: A decadal and comprehensive review of the you only look once series. arXiv preprint arXiv:2406.19407.
[10]. Shatri, E., & Fazekas, G. (2024). Knowledge Discovery in Optical Music Recognition: Enhancing Information Retrieval with Instance Segmentation. arXiv preprint arXiv:2408.15002.
Cite this article
Zhu,J. (2024). Comparative Analysis of Object Detection Models for Sheet Music Recognition: A Focus on YOLO and OMR Technologies. Applied and Computational Engineering,94,33-39.
Data availability
The datasets used and/or analyzed during the current study will be available from the authors upon reasonable request.
Disclaimer/Publisher's Note
The statements, opinions and data contained in all publications are solely those of the individual author(s) and contributor(s) and not of EWA Publishing and/or the editor(s). EWA Publishing and/or the editor(s) disclaim responsibility for any injury to people or property resulting from any ideas, methods, instructions or products referred to in the content.
About volume
Volume title: Proceedings of CONF-MLA 2024 Workshop: Securing the Future: Empowering Cyber Defense with Machine Learning and Deep Learning
© 2024 by the author(s). Licensee EWA Publishing, Oxford, UK. This article is an open access article distributed under the terms and
conditions of the Creative Commons Attribution (CC BY) license. Authors who
publish this series agree to the following terms:
1. Authors retain copyright and grant the series right of first publication with the work simultaneously licensed under a Creative Commons
Attribution License that allows others to share the work with an acknowledgment of the work's authorship and initial publication in this
series.
2. Authors are able to enter into separate, additional contractual arrangements for the non-exclusive distribution of the series's published
version of the work (e.g., post it to an institutional repository or publish it in a book), with an acknowledgment of its initial
publication in this series.
3. Authors are permitted and encouraged to post their work online (e.g., in institutional repositories or on their website) prior to and
during the submission process, as it can lead to productive exchanges, as well as earlier and greater citation of published work (See
Open access policy for details).
References
[1]. Shatri, E., & Fazekas, G. (2020). Optical music recognition: State of the art and major challenges. arXiv preprint arXiv:2006.07885.
[2]. Calvo-Zaragoza, J., Jr, J. H., & Pacha, A. (2020). Understanding optical music recognition. ACM Computing Surveys, 53(4), 1-35.
[3]. LeCun, Y., Bengio, Y., & Hinton, G. (2015). Deep learning. nature, 521(7553), 436-444.
[4]. Guo, Y., Liu, Y., Oerlemans, A., Lao, S., Wu, S., & Lew, M. S. (2016). Deep learning for visual understanding: A review. Neurocomputing, 187, 27-48.
[5]. Ríos-Vila, A., Calvo-Zaragoza, J., Rizo, D., & Paquet, T. (2024). Sheet Music Transformer++: End-to-End Full-Page Optical Music Recognition for Pianoform Sheet Music. arXiv preprint arXiv:2405.12105.
[6]. Mayer, J., Straka, M., & Pecina, P. (2024). Practical End-to-End Optical Music Recognition for Pianoform Music. arXiv preprint arXiv:2403.13763.
[7]. Redmon, J., Divvala, S., Girshick, R., & Farhadi, A. (2016). You only look once: Unified, real-time object detection. In Proceedings of the IEEE conference on computer vision and pattern recognition, 779-788.
[8]. Vijayakumar, A., & Vairavasundaram, S. (2024). Yolo-based object detection models: A review and its applications. Multimedia Tools and Applications, 1-40.
[9]. Sapkota, R., Qureshi, R., Calero, M. F., Hussain, M., Badjugar, C., Nepal, U., ... & Karkee, M. (2024). Yolov10 to its genesis: A decadal and comprehensive review of the you only look once series. arXiv preprint arXiv:2406.19407.
[10]. Shatri, E., & Fazekas, G. (2024). Knowledge Discovery in Optical Music Recognition: Enhancing Information Retrieval with Instance Segmentation. arXiv preprint arXiv:2408.15002.