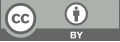
User Behavior and Satisfaction in AI-Generated Video Tools: Insights from Surveys and Online Comments
- 1 School of Future Science and Engineering, Soochow University, Suzhou, 215222, China
* Author to whom correspondence should be addressed.
Abstract
With the rapid advancement of AI, AI-generated video tools have become essential in digital content creation. However, there are significant differences in user acceptance and satisfaction with these tools. This study aims to explore the underlying reasons for these differences by analyzing user behavior, satisfaction levels, and preference characteristics through a questionnaire survey and online review data. Ridge regression analysis identified usage frequency and data security concerns as key factors influencing satisfaction, with familiarity and education level also playing significant roles. Based on these factors, K-means clustering categorized users into three groups: occasional users with high satisfaction, functionality-focused users with the highest satisfaction, and frequent users with lower satisfaction due to data security concerns. Sentiment analysis and LDA topic modeling of online reviews for five AI video tools-Animoto, Invideo, Lumen5, Pictory, and Synthesia-revealed differences in user evaluations. The findings indicate that Lumen5 excels in social media content creation, while Pictory has a weaker user experience with more negative feedback. The study concludes that developers should focus on increasing tool usage frequency and enhancing data security to improve user satisfaction. Moreover, targeted strategies based on different user segments can aid in more precise product optimization and market promotion. This research provides practical guidance for the further development of AI-generated video tools. Future studies could expand the sample size and data dimensions to further validate and deepen these conclusions.
Keywords
AI-generated video tools, ridge regression, K-means clustering, LDA topic modeling.
[1]. Leiker D, Gyllen A R, Eldesouky I and Cukurova M 2023 Generative AI for learning: Investigating the potential of synthetic learning videos. Working paper.
[2]. Isler M, Yesilbel B R, Santos V and Bacalhau L M 2023 Usage of Artificial Intelligence for Advertising Creation for Social Media Marketing: ChatGPT Combined with Pictory and DALL· E. In International Conference on Marketing and Smart Technologies, Springer Nature,73-85.
[3]. Kaur G, Kaur A and Khurana M 2024 A Survey of Computational Techniques for Automated Video Creation and their Evaluation. 2024 11th International Conference on Reliability, Infocom Technologies and Optimization (Trends and Future Directions) (ICRITO), 1-6.
[4]. Nuramila N, et al. 2024 Pembuatan Media Pembelajaran Berbasis Virtual Assistant Pictory & Fliki AI (Artificial Intelligence) Di SMP Negeri 6 Kota Gorontalo. Jurnal Pengabdian Bersama Masyarakat Indonesia, 2(1), 55-65.
[5]. Vayadande K, Bohri M, Chawala M, Kulkarni A M and Mursal A 2024 The Rise of AI-Generated News Videos: A Detailed Review. How Machine Learning is Innovating Today's World: A Concise Technical Guide, 423-451.
[6]. Zhang X 2023 How does AI-generated voice affect online video creation: evidence from TikTok. Doctoral dissertation, University of British Columbia.
[7]. Arashi M, Roozbeh M, Hamzah N A and Gasparini M 2021 Ridge regression and its applications in genetic studies. Plos one, 16(4), e0245376.
[8]. Chi D 2021 Research on the Application of K-Means Clustering Algorithm in Student Achievement. 2021 IEEE International Conference on Consumer Electronics and Computer Engineering (ICCECE), 435-438.
[9]. Barik K and Misra S 2024 Analysis of customer reviews with an improved VADER lexicon classifier. Journal of Big Data, 11(1), 10.
[10]. Rishu K V 2024 Comic exploration and Insights: Recent trends in LDA-Based recognition studies. Expert Systems with Applications, 255, 124732.
Cite this article
Hu,Y. (2024). User Behavior and Satisfaction in AI-Generated Video Tools: Insights from Surveys and Online Comments. Applied and Computational Engineering,94,136-145.
Data availability
The datasets used and/or analyzed during the current study will be available from the authors upon reasonable request.
Disclaimer/Publisher's Note
The statements, opinions and data contained in all publications are solely those of the individual author(s) and contributor(s) and not of EWA Publishing and/or the editor(s). EWA Publishing and/or the editor(s) disclaim responsibility for any injury to people or property resulting from any ideas, methods, instructions or products referred to in the content.
About volume
Volume title: Proceedings of CONF-MLA 2024 Workshop: Securing the Future: Empowering Cyber Defense with Machine Learning and Deep Learning
© 2024 by the author(s). Licensee EWA Publishing, Oxford, UK. This article is an open access article distributed under the terms and
conditions of the Creative Commons Attribution (CC BY) license. Authors who
publish this series agree to the following terms:
1. Authors retain copyright and grant the series right of first publication with the work simultaneously licensed under a Creative Commons
Attribution License that allows others to share the work with an acknowledgment of the work's authorship and initial publication in this
series.
2. Authors are able to enter into separate, additional contractual arrangements for the non-exclusive distribution of the series's published
version of the work (e.g., post it to an institutional repository or publish it in a book), with an acknowledgment of its initial
publication in this series.
3. Authors are permitted and encouraged to post their work online (e.g., in institutional repositories or on their website) prior to and
during the submission process, as it can lead to productive exchanges, as well as earlier and greater citation of published work (See
Open access policy for details).