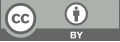
Research on the Principles and Applications of Generative Adversarial Networks in Image Style Transfer
- 1 College of Computer and Information Science, Southwest University School of Software, Southwest University, Chongqing 400715, China
* Author to whom correspondence should be addressed.
Abstract
With the rapid development of machine learning, the field of image style transfer has received widespread attention, and scientists have gradually realized that this is an important field in computer vision. Image style transfer refers to the conversion of an image from one visual style to another and preserving the content. The technology of changing image style has a wide range of applications in various fields from art to virtual reality. Although current learning models have achieved some results in the study of image style transfer, they still face challenges in improving resolution and complex style transfer. This paper mainly introduces the background and characteristics of existing learning models that can achieve image style transfer. It introduces the principles, characteristics and limitations of GANs. In the basis of introduction, this paper also analyses the differences and different characteristics of GANs and summarizes the advantages and disadvantages of GANs. At the same time, this paper predicts future research directions, continuing to optimize these models, reducing training costs, and exploring hybrid methods to further advance image style transfer technology. These developments not only strengthen the theoretical research of image style transfer and GANs, but also have broad application potential in fields such as art, design and medical imaging.
Keywords
image style transfer, GANs, deep learning model, computer vision.
[1]. Gatys L A, Ecker A S and Bethge M 2016 Image style transfer using convolutional neural networks the IEEE conf. on computer vision and pattern recognition pp 2414-23
[2]. Isola P, Zhu J Y, Zhou T and Efros A A 2017 Image-to-image translation with conditional adversarial networks the IEEE conf. on computer vision and pattern recognition pp 1125-34
[3]. Kingma D P and Welling M 2013 Auto-Encoding Variational Bayes CoRR, abs/1312.6114.
[4]. Yu Z and Zhang H 2021 Research and practice of image technology based on artificial intelligence (GAN) the 2021 1st Int. Conf. on Control and Intelligent Robotics pp 291-4
[5]. Liao Y and Huang Y 2022 Deep Learning‐Based Application of Image Style Transfer Mathematical Problems in Engineering 2022(1) 1693892.
[6]. [6] Xu X, Chang J and Ding S 2022 Image Style Transfering Based on StarGAN and Class Encoder Int. Journal of Software & Informatics, 12(2).
[7]. Richardson E, Alaluf Y, Patashnik O, Nitzan Y, Azar Y, Shapiro S and Cohen-Or, D. 2021 Encoding in style: a stylegan encoder for image-to-image translation IEEE/CVF conf. on computer vision and pattern recognition pp 2287-96
[8]. Zenoozi A D, Navi K and Majidi B 2022, February Argan: fast converging gan for animation style transfer 2022 Int. Conf. on Machine Vision and Image Processing (MVIP) pp 1-5
[9]. Chong M J and Forsyth D 2022, October Jojogan: One shot face stylization European Conf. on Computer Vision pp 128-5
[10]. Chai X, Zhou J, Zhou H and Lai J 2022, October PDD-GAN: Prior-based GAN network with decoupling ability for single image dehazing 30th ACM Int. Conf. on Multimedia pp 5952-60
Cite this article
Qu,Y. (2024). Research on the Principles and Applications of Generative Adversarial Networks in Image Style Transfer. Applied and Computational Engineering,82,29-34.
Data availability
The datasets used and/or analyzed during the current study will be available from the authors upon reasonable request.
Disclaimer/Publisher's Note
The statements, opinions and data contained in all publications are solely those of the individual author(s) and contributor(s) and not of EWA Publishing and/or the editor(s). EWA Publishing and/or the editor(s) disclaim responsibility for any injury to people or property resulting from any ideas, methods, instructions or products referred to in the content.
About volume
Volume title: Proceedings of the 2nd International Conference on Machine Learning and Automation
© 2024 by the author(s). Licensee EWA Publishing, Oxford, UK. This article is an open access article distributed under the terms and
conditions of the Creative Commons Attribution (CC BY) license. Authors who
publish this series agree to the following terms:
1. Authors retain copyright and grant the series right of first publication with the work simultaneously licensed under a Creative Commons
Attribution License that allows others to share the work with an acknowledgment of the work's authorship and initial publication in this
series.
2. Authors are able to enter into separate, additional contractual arrangements for the non-exclusive distribution of the series's published
version of the work (e.g., post it to an institutional repository or publish it in a book), with an acknowledgment of its initial
publication in this series.
3. Authors are permitted and encouraged to post their work online (e.g., in institutional repositories or on their website) prior to and
during the submission process, as it can lead to productive exchanges, as well as earlier and greater citation of published work (See
Open access policy for details).