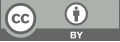
An Adaptive Cruise Control Algorithm Based on DDPG Algorithm Based on Deep Reinforcement Learning Under Variable Acceleration Conditions
- 1 College of Automotive Sciences, Tongji University, Shanghai, China
* Author to whom correspondence should be addressed.
Abstract
Adaptive cruise control (ACC) dynamically regulates a vehicle's speed to preserve a secure gap from the preceding vehicle, enhancing road safety. In this study, ACC is examined through the lens of deep reinforcement learning, with a focus on the Deep Deterministic Policy Gradient (DDPG) technique. The reward function takes into account the speed error, and two modes—speed control and distance control—are implemented. The proposed ACC strategy is trained and validated through simulations on the MATLAB/Simulink platform. The experimental results indicate that the reward function converges rapidly, confirming the suitability of the DDPG algorithm for automotive ACC research.
Keywords
Reinforcement Learning, DDPG, Adaptive Cruise, Intelligent Vehicle.
[1]. Yuchuan Fu, Changle Li, Fei Richard Yu. A decision-making strategy for vehicle autonomous braking in emergency via deep reinforcement learning[J]. IEEE Transactions on Vehicular Technology, 2020, 69(6):5876-5888.
[2]. Jindong Zhang, Haoting Zhong. Curve-based lane estimation model with lightweight attention mechanism[J]. Signal, Image and Video Processing, 2023, 17(5):2637-2643.
[3]. Tianbo Liu, Jindong Zhang. An adaptive traffic flow prediction model based on spatiotemporal graph neural network[J]. The Journal of Supercomputing, 2023, 79(14):1-25.
[4]. Jindong Zhang, Jian Dou. An adversarial pedestrian detection model based on virtual fisheye image training[J]. Signal, Image and Video Processing, 2024, 18(4):3527-3535.
[5]. Jingyi Jin, Jindong Zhang, Kunpeng Zhang, et al. 3D multi-object tracking with boosting data association and improved trajectory management mechanism[J]. Signal Processing, 2024, 218(2024):109367
[6]. Charles Desjardins, Brahim Chaib-draa. Cooperative adaptive cruise control: a reinforcement learning approach[J]. IEEE Transactions on intelligent transportation systems, 2011, 12(4):1248-1260.
[7]. Li, Meng, Cao Zehong, Li Zhibin. A reinforcement learning-Based vehicle platoon control strategy for reducing energy consumption in traffic oscillations[J]. IEEE Transactions on Neural Networks & Learning Systems, 2021, 32(12):5309-5322.
Cite this article
Lan,Y. (2024). An Adaptive Cruise Control Algorithm Based on DDPG Algorithm Based on Deep Reinforcement Learning Under Variable Acceleration Conditions. Applied and Computational Engineering,106,37-43.
Data availability
The datasets used and/or analyzed during the current study will be available from the authors upon reasonable request.
Disclaimer/Publisher's Note
The statements, opinions and data contained in all publications are solely those of the individual author(s) and contributor(s) and not of EWA Publishing and/or the editor(s). EWA Publishing and/or the editor(s) disclaim responsibility for any injury to people or property resulting from any ideas, methods, instructions or products referred to in the content.
About volume
Volume title: Proceedings of the 2nd International Conference on Machine Learning and Automation
© 2024 by the author(s). Licensee EWA Publishing, Oxford, UK. This article is an open access article distributed under the terms and
conditions of the Creative Commons Attribution (CC BY) license. Authors who
publish this series agree to the following terms:
1. Authors retain copyright and grant the series right of first publication with the work simultaneously licensed under a Creative Commons
Attribution License that allows others to share the work with an acknowledgment of the work's authorship and initial publication in this
series.
2. Authors are able to enter into separate, additional contractual arrangements for the non-exclusive distribution of the series's published
version of the work (e.g., post it to an institutional repository or publish it in a book), with an acknowledgment of its initial
publication in this series.
3. Authors are permitted and encouraged to post their work online (e.g., in institutional repositories or on their website) prior to and
during the submission process, as it can lead to productive exchanges, as well as earlier and greater citation of published work (See
Open access policy for details).