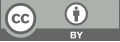
A Review on Human Pose Estimation Based on Deep Learning
- 1 School of Mathematics and Information Science, South China Agricultural University, Guangdong, China
* Author to whom correspondence should be addressed.
Abstract
With the rapid development of computer vision technology, deep learning-based human pose estimation has become a hot topic of research. This review outlines the progress made in this field in recent years, with a particular focus on the development of single-person and multi-person pose estimation. Single-person pose estimation primarily focuses on identifying and locating the joints of an individual, while multi-person pose estimation further extends to simultaneously recognizing the poses of multiple individuals. The article begins by introducing the basic concepts of pose estimation, then discusses in detail the application of deep learning models in single-person and multi-person pose estimation, as well as the advantages and disadvantages of the existing modules. In addition, the limitations of current models are analyzed at the end of the paper, and possible future optimization directions are explored. The aim of this article is to provide researchers with a comprehensive perspective to understand current technological trends and potential innovation points.
Keywords
Computer Vision, Single-Person Pose Estimation, Multi-Person Pose Estimation, Deep Learning.
[1]. Wang, K., et al. (2024). A Review of 2D Human Pose Estimation Based on Deep Learning. Journal of Zhengzhou University (Science Edition) 56.04: 11-20.
[2]. Zhang, G.P., et al. (2022). Research Progress of Deep Learning Methods in 2D Human Pose Estimation. Computer Science 49.12: 219-228.
[3]. Shi, X. R., (2023). Research on 3D Human Pose Estimation Based on Deep Learning. East China Normal University, MA thesis.
[4]. Yu, B. L., et al. (2020). A Multi-Target Human Skeleton Node Detection Algorithm Based on DeepPose and Faster RCNN. Journal of the Chinese Academy of Sciences 37.06: 828-834.
[5]. Wang, J.Y., et al. (2022). A Method for Identifying Divers' Operating Postures Based on Convolutional Pose Machine. Journal of Zhejiang University (Engineering Science) 56.0: 26-35+46.
[6]. Xiao, Y.B. (2023). Research on Human Pose Estimation, Behavior Analysis, and Application Based on Deep Learning. Beijing University of Posts and Telecommunications, PhD dissertation.
[7]. Jia, Y.G., et al. (2023). Standardized Evaluation of Running Action Based on Alphapose. Computer Era. 08: 117-120.
[8]. Liao, J.H., et al. (2024). Lightweight HRNet: A Ligtweight Network for Bottom-Up Human Pose Estimation. Engineering Letters 32. 3.
[9]. Artacho, B. and Savakis A. (2020). UniPose: Unified human pose estimation in single images and videos. Proceedings of the IEEE Computer Society Conference on Computer Vision and Pattern Recognition.
[10]. Chen, G.L., and Pang Y.S. (2024). An Improved Faster RCNN Algorithm for Micro-Operation Spatial Target Detection. Sensors and Microsystems 43.03: 144-147+151.
[11]. Li, J. (2021). Research on Bottom-Up Multi-Person Pose Estimation Methods. University of Science and Technology of China, PhD dissertation.
[12]. Sun, L. (2022). SPLP: A Certifiably Globally Optimal Solution to the Relative Pose Estimation Problem Using Points and Line Pairs." Journal of Interconnection Networks 22. Supp02.
[13]. Li, X.T. (2023). Research on Human Action Recognition Based on Deep Learning. Shanxi University, MA thesis.
[14]. Micheal D., et al. (2024). An Interpretable Modular Deep Learning Framework for Video-Based Fall Detection. Applied Sciences 14.11.
[15]. Eldar I., et al. (2016). DeeperCut: A Deeper, Stronger, and Faster Multi-Person Pose Estimation Model. CoRR abs/1605.03170.
Cite this article
Chen,W. (2024). A Review on Human Pose Estimation Based on Deep Learning. Applied and Computational Engineering,80,67-73.
Data availability
The datasets used and/or analyzed during the current study will be available from the authors upon reasonable request.
Disclaimer/Publisher's Note
The statements, opinions and data contained in all publications are solely those of the individual author(s) and contributor(s) and not of EWA Publishing and/or the editor(s). EWA Publishing and/or the editor(s) disclaim responsibility for any injury to people or property resulting from any ideas, methods, instructions or products referred to in the content.
About volume
Volume title: Proceedings of CONF-MLA Workshop: Mastering the Art of GANs: Unleashing Creativity with Generative Adversarial Networks
© 2024 by the author(s). Licensee EWA Publishing, Oxford, UK. This article is an open access article distributed under the terms and
conditions of the Creative Commons Attribution (CC BY) license. Authors who
publish this series agree to the following terms:
1. Authors retain copyright and grant the series right of first publication with the work simultaneously licensed under a Creative Commons
Attribution License that allows others to share the work with an acknowledgment of the work's authorship and initial publication in this
series.
2. Authors are able to enter into separate, additional contractual arrangements for the non-exclusive distribution of the series's published
version of the work (e.g., post it to an institutional repository or publish it in a book), with an acknowledgment of its initial
publication in this series.
3. Authors are permitted and encouraged to post their work online (e.g., in institutional repositories or on their website) prior to and
during the submission process, as it can lead to productive exchanges, as well as earlier and greater citation of published work (See
Open access policy for details).