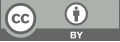
A Review and Outlook of the Latest Results on Document-level Information Extraction
- 1 School of Computer Information and Engineering, School of Henan University of Economics and Law, Zhengzhou, China
- 2 College of Computer Science and Technology, Hainan University, Haikou, China
- 3 School of Computer Information and Engineering, School of Henan University of Economics and Law, Zhengzhou, China
* Author to whom correspondence should be addressed.
Abstract
Relationship extraction is a key step in information extraction and aims to identify entities from text data and identify semantic links between them, which is the foundation of knowledge graph construction. Improving relationship extraction efficiency can effectively enhance the quality of information extraction, which has an impact on the construction of a knowledge graph and subsequent downstream tasks. The paper improves the efficiency and quality of relationship extraction by optimizing the model, choosing the way of relationship extraction and proposing new evaluation parameter criteria. The paper summarizes the current state-of-the-art research results, and briefly describes SIEF, an evaluation mechanism that focuses on important sentences, Coreference Aid, which captures the internal structure of entities as well as the external fine-grained information, and FILR, a framework that extracts global document information and performs multi-granular logical reasoning. Aggregate semi-automated data augmentation by integrating large model languages, remote supervision and construction of domain graphs integrating domain information of entities, cross-article entity-centered relationship extraction capable of capturing connections between different articles, and the introduction of mean-accuracy averaging capable of evaluating the comprehensibility of the model are presented. This paper presents a vision of new and better optimization algorithms by describing the latest approaches to relationship extraction optimization and finding that they all have their own limitations.
Keywords
Document-Level Relationship Extraction, Framework Optimization, Natural Language Processing, Model Evaluation, Cross-document Relation Extraction.
[1]. Xu, W., Chen, K. H., Mou, L. L., Zhao, T. J. (2022). Document-Level Relation Extraction with Sentences Importance Estimation and FocusingProceedings of the 2022 Conference of the North American Chapter of the Association for Computational Linguistics: Human Language Technologies, pages 2920 - 2929
[2]. Lu, C., Zhang, R., Sun, K., Kim, J., Zhang, C., Mao, Y. (2023). Anaphor Assisted Document-Level Relation Extraction. Proceedings of the 2023 Conference on Empirical Methods in Natural Language Processing, pages 15453-15464
[3]. Li, L., Lian, R., Lu, H., Tang, J. (2023). Document-level Biomedical Relation Extraction Based on Multi-Dimensional Fusion Information and Multi-Granularity Logical Reasoning. Proceedings of the 29th International Conference on Computational Linguistics, pages 2098-2107
[4]. Li, J., Jia, Z., Zheng, Z. (2024). Semi-automatic Data Enhancement for Document-Level Relation Extraction with Distant Supervision from Large Language Models. Technical Report. National Key Laboratory of General Artificial Intelligence, BIGAI. https://github.com/bigai-nlco/DocGNR
[5]. Wang, F., Li, F., Fei, H., Li, J., Wu, S., Su, F., Shi, W., Ji, D., & Cai, B. (2022). Entity-centered Cross-document Relation Extraction. Title of Periodical, volume number (issue number).
[6]. Wei, Z., Su, J., Wang, Y., Tian, Y., & Chang, Y. (2020). A Novel Cascade Binary Tagging Framework for Relational Triple Extraction. Technical Report. School of Artificial Intelligence, Jilin University; Key Laboratory of Symbolic Computation and Knowledge Engineering of Ministry of Education; International Center of Future Science, Jilin University; Shenzhen Zhuiyi Technology Co.; University of North Carolina at Chapel Hill.
[7]. Sundararajan, M., Taly, A., & Yan, Q. (2017). Axiomatic Attribution for Deep Networks. Proceedings of the 34th International Conference on Machine Learning, Volume 70 of Proceedings of Machine Learning Research, pages 3319-3328. PMLR.
[8]. Mudrakarta, P. K., Taly, A., Sundararajan, M., & Dhamdhere, K. (2018). Did the Model Understand the Question? In Proceedings of the 56th Annual Meeting of the Association for Computational Linguistics (Volume 1: Long Papers), pages 1896-1906, Melbourne, Australia. Association for Computational Linguistics.
[9]. Liu, F., & Avci, B. (2019). Incorporating Priors with Feature Attribution on Text Classification. In Proceedings of the 57th Annual Meeting of the Association for Computational Linguistics, pages 6274-6283, Florence, Italy. Association for Computational Linguistics
[10]. Bastings, J., & Filippova, K. (2020). The Elephant in the Interpretability Room: Why Use Attention as Explanation When We Have Saliency Methods? In Proceedings of the Third BlackboxNLP Workshop on Analyzing and Interpreting Neural Networks for NLP, pages 149-155, Online. Association for Computational Linguistics.
[11]. Hao, Y., Dong, L., Wei, F., & Xu, K. (2021). Self Attention Attribution: Interpreting Information Interactions Inside Transformer. In Proceedings of the AAAI Conference on Artificial Intelligence, Volume 35, pages 12963-12971.
[12]. Nigam, K., McCallum, A., Kachites, C., Thrun, S., & Mitchell, T. (2000). Text Classification from Labeled and Unlabeled Documents Using EM. Technical Report. School of Computer Science, Carnegie Mellon University, Pittsburgh, PA 15213, USA.
Cite this article
Li,H.;Wei,L.;Wang,Z. (2024). A Review and Outlook of the Latest Results on Document-level Information Extraction. Applied and Computational Engineering,96,120-129.
Data availability
The datasets used and/or analyzed during the current study will be available from the authors upon reasonable request.
Disclaimer/Publisher's Note
The statements, opinions and data contained in all publications are solely those of the individual author(s) and contributor(s) and not of EWA Publishing and/or the editor(s). EWA Publishing and/or the editor(s) disclaim responsibility for any injury to people or property resulting from any ideas, methods, instructions or products referred to in the content.
About volume
Volume title: Proceedings of the 2nd International Conference on Machine Learning and Automation
© 2024 by the author(s). Licensee EWA Publishing, Oxford, UK. This article is an open access article distributed under the terms and
conditions of the Creative Commons Attribution (CC BY) license. Authors who
publish this series agree to the following terms:
1. Authors retain copyright and grant the series right of first publication with the work simultaneously licensed under a Creative Commons
Attribution License that allows others to share the work with an acknowledgment of the work's authorship and initial publication in this
series.
2. Authors are able to enter into separate, additional contractual arrangements for the non-exclusive distribution of the series's published
version of the work (e.g., post it to an institutional repository or publish it in a book), with an acknowledgment of its initial
publication in this series.
3. Authors are permitted and encouraged to post their work online (e.g., in institutional repositories or on their website) prior to and
during the submission process, as it can lead to productive exchanges, as well as earlier and greater citation of published work (See
Open access policy for details).