1 Introduction
In the field of Natural Language Processing (NLP), meta-learning has demonstrated significant potential in few-shot learning scenarios. Compared to traditional learning models such as transfer learning and multi-task learning, meta-learning requires fewer training samples, allowing models to quickly adapt and perform well even in situations where only a small number of training examples are available [1]. A key feature of meta-learning is its ability to generalize the learning experience from previous tasks to new, similar types of tasks. These characteristics enable meta-learning to shine in fields where acquiring samples is costly, difficult, or where previous samples are scarce, such as medicine, low-resource languages, and astronomical observations. For instance, a pioneering optimization-based meta-learning algorithm, XG-REPTILE, has been applied to cross-lingual semantic parsing. It significantly reduces the need for samples in low-resource languages while demonstrating strong generalization capabilities across multiple datasets [2]. In literature [3], the authors introduced a meta-learning approach to address the problem of low-resource Automated Program Repair (APR) by designing the Meta-APR framework. By pre-training the model on a large number of high-resource bugs, the framework enables the model to quickly adapt when dealing with low-resource bugs. However, meta-learning also faces numerous challenges and difficulties, especially in complex application scenarios.
In literature [4], the authors propose and explore advanced deep meta-learning techniques. The paper introduces three categories of meta-learning methods and summarizes their respective advantages and disadvantages. The main challenges include limited generalization capabilities and adaptability when there are significant gaps between tasks, the risk of overfitting in few-shot scenarios, and high computational costs and resource requirements. For instance, the previously mentioned optimization-based meta-learning algorithm XG-REPTILE [2] addresses computational resource issues by employing first-order approximation methods like Reptile to ensure both performance and efficiency. However, the paper highlights that the algorithm still faces generalization and adaptability problems when aligning and generalizing across languages with large structural and form differences, where the task gap becomes too significant.
To further explore the potential advantages and challenges of meta-learning, this paper synthesizes recent cutting-edge research in applications such as sentiment analysis, complaint detection, and cross-task generalization. It systematically investigates how meta-learning optimizes parameter initialization to enhance a model's generalization capability on unseen or rarely encountered tasks. Additionally, this paper will conduct an in-depth analysis of the strengths and weaknesses of meta-learning in various complex applications, comparing its performance in natural language processing tasks like prompt tuning, multimodal learning, and few-shot classification. Finally, the paper will discuss how meta-learning demonstrates advantages over multi-task learning and analyze the challenges it faces in practical applications.
Structurally, this paper begins by citing a general meta-learning framework [5] in Section 2, which serves as the foundation for clarifying the basic definition of meta-learning and analyzing its key characteristics. This section also summarizes three main features of meta-learning, laying the groundwork for analyzing the potential advantages and challenges in subsequent research. In Section 3, based on the understanding of meta-learning established earlier, the focus shifts to exploring cutting-edge research on three topics: federated learning frameworks, cross-task generalization, and few-shot classification. Each topic is introduced with its research background and methodologies, followed by an analysis of experimental results, and finally, a comparison and summary of the findings. In Section 4, the paper synthesizes the advantages and challenges identified in the studies of these complex meta-learning applications and provides an analysis of the future development and prospects of meta-learning considering these factors.
2 Overview of Meta-learning Techniques
Today, in the field of machine learning, using deep neural networks to extract features from data for analysis has become a mainstream approach. However, the limitation of this method lies in the requirement for large amounts of input data to ensure the model's ability to solve specific tasks. This presents a significant challenge in scenarios where only few-shot or zero-shot learning is available. Additionally, traditional learning methods often struggle to generalize quickly from one domain to a similar new domain in certain specialized fields.
Meta-learning, as a potential solution, enables models to quickly adapt to new tasks with few samples while also generalizing across similar tasks. By allowing models to learn the process of learning itself, meta-learning enhances their ability to cope with and adapt to situations where data is scarce, expensive to obtain, or where environments change frequently.
2.1 Distinguishing Learning from Meta-Learning
In literature [5], the authors first propose that it is essential to understand and differentiate between learning and meta-learning in the context of machine intelligence problems. The learning process of a machine can be viewed as a function A(L):
\( A(L):K_{L}×D→M \) (1)
Where KL represents the configuration parameter space of the given learning machine L, and D denotes the space of data streams, which provide the learning material. M defines the space of target models.
Since learning itself is a process, the parameters of machine M during learning are determined by the algorithm L. Meta-learning, on the other hand, can be considered a more specific form of learning. In other words, its target model (output) is the configuration of the learning machine obtained through the meta-learning algorithm. This implies that, in theory, meta-learning is typically unrestricted, except by memory and time constraints.
2.2 General MLA Framework
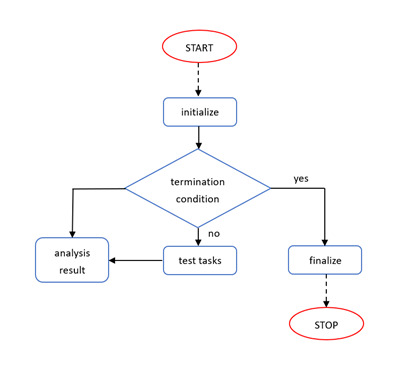
Figure 1. General meta-learning algorithm.
Figure 1 illustrates a general meta-learning algorithm (MLAs) based on observational learning, as proposed in the literature [5]. After initialization, the method enters a main loop and terminates under specific conditions. In each iteration, the algorithm defines several tasks and evaluates the performance of the corresponding learning configurations. By controlling the complexity of the test tasks, the loop adjusts the meta-learning process. This allows the dynamic selection and reordering of tasks based on feedback, helping the model adapt to various tasks, reduce redundant test tasks, and improve efficiency. However, the selection of the initial test tasks is crucial; an inappropriate initial setup may affect the search process, reducing learning efficiency.
After the testing phase, the system evaluates the results and converts them into meta-knowledge, which is used to adjust the algorithm's complexity and the machine's behavior, thereby improving efficiency and avoiding resource waste. Meta-knowledge can be accumulated over time, assisting the meta-learning algorithm in solving future tasks and enhancing its generalization ability to similar tasks. However, storing large amounts of meta-knowledge may lead to overfitting and increase storage requirements.
This approach not only conducts searches by progressively observing tasks (with the MLA autonomously determining the task group to initiate) but also maintains a dynamic and evolving search space, capable of covering various types of learning algorithms and dynamically generating learning machines. Through multiple iterations, it tests different algorithm configurations to find the optimal solution.
As a result, complex meta-learning algorithms exhibit greater openness to intermediate tasks and aim to find the optimal solution in the shortest possible time. When designing meta-learning algorithms, careful consideration should be given to the sequence of test tasks, incorporating previous experience to accumulate meta-knowledge.
2.3 Classification of Meta-Learning
In literature [4], the authors adopt the mainstream classification of meta-learning approaches, namely, metric-based, model-based, and optimization-based methods. Each approach has its own underlying principles, advantages, and limitations across different application scenarios.
Metric-based methods perform classification or regression by calculating the similarity between samples, with the fundamental assumption that similar tasks have similar representations in the feature space. Prototypical Networks, a classic algorithm, computes prototype vectors for each class (the mean of all samples within a class) and classifies new samples by comparing their distance to these prototypes. These methods are mainly applied in few-shot supervised learning tasks such as image and text classification. While they are conceptually simple and easy to implement, they perform poorly when task differences are large.
Model-based methods involve learning a model architecture that can quickly adapt to new tasks, often relying on recurrent neural networks (RNNs) to share information across tasks. These methods are suitable for reinforcement learning or unsupervised learning scenarios. However, when task variability is high, the generalization capability of the model may be compromised.
Optimization-based methods focus on learning a set of shared initial parameters, enabling the model to adapt quickly to new tasks with just a few gradient updates. The most representative algorithm in this category is MAML (Model-Agnostic Meta-Learning), which aims to train a set of initial parameters that generalize well across tasks, allowing good performance on new tasks after minimal updates. These methods are particularly effective in scenarios requiring rapid task adaptation, but the complex gradient optimization process leads to high computational costs, and the choice of initial parameters is crucial.
3 Meta-learning applications and challenges
This article will introduce and analyze several practical applications of meta-learning in more complex scenarios.
3.1 Meta-learning with Federated Learning
Federated Learning (FL), as a distributed learning method, allows multiple clients to store data locally and share model training, thereby ensuring data privacy. The study in literature [6] proposes a multimodal framework based on FL, which combines text and image data to address emotion-aware complaint recognition. This framework trains models locally and aggregates parameters to generate a global model, preventing data from leaving local devices and thus protecting privacy.
The study collected reviews from the Amazon India website, to build a multimodal complaint dataset with detailed annotations. The research tackled three tasks: complaint recognition, emotion recognition, and sentiment analysis, represented by cm, em, and sm, respectively. The core goal of this multi-task learning framework is to maximize the posterior probability of all tasks, as shown by the following equation, where θ represents the model parameters:
\( \prod_{m=0}^{M}P(s_{m}, e_{m}, c_{m} | x_{m} ; θ)\ \ \ (2) \)
The authors adopted an FL setup and proposed a Federated Meta-Learning framework based on Prototypical Networks (ProtoFed-MCI), which integrates metric-based learning. This enables each client to utilize prior knowledge from the support set to classify multimodal information such as titles, reviews, and images in the query set. The objective of each communication round is to optimize the global parameters transmitted to the clients. Additionally, multimodal feature extraction, modality encoders, and attention mechanisms were introduced to identify and extract key information from the dataset.
Table 1. Classification results of the baseline models and the proposed framework
Model |
MCD Dataset |
|||
Text |
Text + Image |
|||
F1 |
A |
F1 |
A |
|
Centralized Baselines |
||||
SOTA |
85.58 |
86.39 |
- |
- |
BSPMF |
85.43 |
86.17 |
- |
- |
Federated Learning Baseline |
||||
Fed-BMTL |
83.22 |
84.37 |
- |
- |
Fed-MCI |
- |
- |
84.33 |
86.46 |
Fed-ALBERT |
- |
83.51 |
84.85 |
|
Proposed approach |
||||
Proto-Fed MCI |
- |
- |
89.00* |
89.06* |
The authors conducted experiments using PyTorch and evaluated the effects of attention mechanisms, multimodal, and multi-task learning through ablation studies. Table 1 presents the classification results of the baseline models and the proposed framework, listing the performance in terms of macro F1-score (F1) and accuracy (A). ProtoFed-MCI outperforms all baseline models, especially when selecting 15 random clients over 25 communication rounds, demonstrating better generalization ability and proving the advantages of federated meta-learning in collaborative environments.
Table 2. Results of the centralized framework (MCI) and various ablation studies
Model |
MCD Dataset |
|||
Text |
Text+Image |
|||
F1 |
A |
F1 |
A |
|
Single-task Baselines |
||||
STLT |
86.78 |
86.96 |
- |
- |
STLT+I |
- |
- |
87.77 |
88.49 |
Multi-task Baselines |
||||
MTLT |
88.32 |
89.58 |
- |
- |
MTLT+I |
- |
- |
90.05 |
90.71 |
Multi-modal Baselines |
||||
MCICE |
- |
- |
88.71 |
89.95 |
MCICS |
- |
- |
87.43 |
88.65 |
MCIA |
- |
- |
89.38 |
90.21 |
MCIA |
- |
- |
89.20 |
90.35 |
Centralized Approach |
||||
MCI |
- |
- |
91.20* |
91.95* |
Table 2 presents the results of the centralized framework (MCI) and various ablation studies. The experiments confirm the rationale and effectiveness of the multi-task and multimodal design. Ablation studies show that integrating multiple tasks and modalities significantly improves model performance, especially after combining the tasks of complaint detection, emotion recognition, and sentiment analysis. The introduction of attention mechanisms further enhanced the performance of both ProtoFed-MCI and MCI. Compared to the state-of-the-art (SOTA) techniques, ProtoFed-MCI excels in handling data distribution across multiple clients.
This paper proposes a multi-task framework based on federated meta-learning, focusing on complaint detection in a multimodal environment. It incorporates dual attention mechanisms and optimizes tasks related to complaint, emotion, and sentiment recognition. Future research should focus on finer-grained annotations of complaint severity, sentence-level detection, and further exploration of security and resource utilization in federated meta-learning.
3.2 Cross-Task Generalization
Cross-task generalization has long been a critical challenge in model learning, as differences in distribution between training and testing data domains often lead to performance degradation. In [7], the authors propose a meta-learning model that combines optimization-based Meta-Prompt Tuning (MPT) techniques, aiming to quickly adapt to new domains with limited data and achieve better generalization performance in untrained domains.
The authors adopt an optimization-based meta-learning approach, with the goal of learning initial parameters 𝜙∗ that allow the model to quickly adapt to new tasks with limited data. The model learns from a series of meta-training tasks Tmeta, where each task’s dataset Di is divided into a support set S𝑖 and a query set Q𝑖. The learning objective is defined as, where L is the objective function, 𝜙 represents the parameters to be optimized, and 𝛼 is the inner learning rate.:
\( ϕ^{*}=argmin_{ϕ}\sum_{T_{i}ϵT_{meta}}L(ϕ-α∇_{ϕ}L(ϕ,S_{i}),Q_{i})\ \ \ (3) \)
The authors divide tasks into source tasks and target tasks, and evaluate cross-task generalization performance through a two-stage learning paradigm. The first stage involves upstream learning on source tasks to obtain meta-parameters, while the second stage focuses on downstream learning on target tasks, using the meta-parameters to initialize the model. The performance is evaluated using Average Relative Gain (ARG).
During the meta-training phase, the model trains prompt embeddings on the source tasks Tsrc , updating the prompt embedding parameters 𝜙 via gradient updates for use in subsequent meta-testing. In the meta-testing phase, the model uses the learned 𝜙∗ to initialize the unseen target tasks Ttgt, where it is trained and evaluated on these tasks.
In the experiments, the authors used the T5-Large model and compared the following methods:
Prompt Tuning (PT): A baseline method that directly tunes prompts.
MAML: A meta-learning approach that learns initial parameters during upstream learning.
FoMAML and Reptile: Two memory-efficient algorithms compared to MAML.
Multi-task Learning (MTL): Acquires initial parameters through multi-task learning.
Fine-tuning: Fine-tuning the entire model to test cross-task generalization effects.
In the analysis of the experimental results, MPT demonstrated outstanding performance in improving cross-task generalization, particularly in classification tasks, outperforming MTL. However, in some non-classification tasks, MTL performed slightly better due to its robustness to hyperparameter sensitivity and more suitable task-sharing structures. MPT showed robustness across different data scales, consistently outperforming MTL in both few-shot and full-data scenarios. The diversity of source tasks significantly impacted cross-task generalization in non-classification tasks, with increased source task variety further enhancing MPT’s performance. Additionally, MPT demonstrated greater robustness in large-scale models.
This study demonstrates that Meta-Prompt Tuning, by learning prompt embedding initializations, effectively improves cross-task generalization. However, MPT still faces challenges in optimizing hyperparameters, particularly in non-classification tasks where its performance lags behind multi-task learning. Furthermore, the study focuses more on performance improvements while providing insufficient exploration of how meta-learning accelerates model convergence. Lastly, MPT's reliance on computationally intensive second-order gradient optimization results in high memory resource demands when dealing with large-scale models and datasets.
3.3 Few-shot text classification
The PDAMeta meta-learning framework proposed in [8] combines the Progressive Data Augmentation (PDA) method and Dual-stream Contrastive Meta-learning, aiming to learn discriminative text representations from both original and augmented samples. This approach uses a dual-stream design, where two meta-learners are trained separately, applying constraints during the cross-task learning of meta-knowledge, thereby enhancing the model's generalization capability. The progressive augmentation further improves the model’s robustness when handling few-shot data.
PDAMeta leverages dual-stream contrastive meta-learning to simultaneously optimize the representations of both original and augmented samples, thereby acquiring richer meta-knowledge. A key advantage of this design is that the meta-learning framework can capture useful knowledge across multiple tasks, strengthening the model’s few-shot learning ability. By introducing progressive data augmentation, PDAMeta excels in handling unevenly distributed data. Additionally, the dual-stream contrastive learning strategy encourages the model to learn more distinctive representations during training on support and query sets, which in turn improves classification performance.
The experiments were conducted using four public few-shot learning (FSL) datasets, implemented in PyTorch. PDAMeta’s performance was compared with several baseline models, and only the most representative ones are shown here:
MAML: An optimization-based method that optimizes model parameters via gradient descent.
PN: A metric-based method that learns effective distance metrics.
HATT: An extension of Prototypical Networks using a hybrid attention mechanism.
ChatGPT: Applied directly for few-shot classification, generating augmented data through prompt design.
MetaPrompting(MP): An optimization-based meta-learning algorithm that searches for soft prompt initialization.
Table 3. Comparison of baseline performance across different datasets
Model |
HuffPost |
Amazon |
Reuters |
20News |
Average |
|||||
shot-5 |
shot-10 |
shot-5 |
shot-10 |
shot-5 |
shot-10 |
shot-5 |
shot-10 |
shot-5 |
shot-10 |
|
MAML |
49.3 |
50.8 |
47.1 |
49.5 |
62.9 |
64.1 |
43.7 |
46.0 |
50.8 |
52.6 |
PN |
41.3 |
42.9 |
52.1 |
54.4 |
66.9 |
68.7 |
45.3 |
46.9 |
51.4 |
53.2 |
HATT |
56.3 |
58.5 |
66.0 |
67.4 |
56.2 |
58.4 |
55.1 |
56.9 |
58.4 |
60.3 |
ChatGPT |
71.5 |
72.5 |
81.5 |
83.4 |
94.3 |
97.1 |
77.3 |
81.1 |
81.2 |
83.5 |
MP |
76.3 |
78.3 |
85.5 |
87.3 |
97.2 |
97.7 |
76.6 |
78.2 |
83.9 |
85.4 |
PDAMeta |
70.3 |
72.8 |
87.2 |
89.4 |
98.5 |
98.9 |
83.1 |
84.5 |
84.8 |
86.4 |
As shown in Table 3. Compared to baseline models, PDAMeta demonstrates outstanding performance, particularly in multi-task learning and multimodal data environments. Although the framework relies on large-scale language models for data augmentation, which could pose limitations in resource-constrained scenarios, its optimization strategy significantly enhances data diversity and classification accuracy.
This paper analyzes the effectiveness of meta-learning frameworks in few-shot learning, highlighting how progressive data augmentation and contrastive learning can improve the capture of meta-knowledge. Future research should address issues related to the quality and diversity of augmented data, computational costs, the selection of negative samples in contrastive learning, and cross-domain generalization. Solving these challenges will further advance the application of meta-learning.
4 Conclusion
This paper has thoroughly explored the applications and challenges of meta-learning in the field of natural language processing, highlighting its potential and limitations through specific research cases. By comparing various meta-learning methods and conducting experiments, the study reveals the unique advantages of meta-learning in prompt tuning, federated learning, and cross-task learning, particularly in scenarios where data is scarce and tasks are highly diverse. Meta-learning demonstrates significant effectiveness, yet it still faces numerous challenges in practical applications.
One of the main challenges is that the ability of meta-learning to share knowledge between old and new tasks heavily relies on the similarity between tasks. When there is a large discrepancy between tasks, the generalization capability of meta-learning may be limited. Additionally, certain meta-learning algorithms, such as MAML and FoMAML, are highly sensitive to hyperparameter settings, particularly when adjusting for optimal settings across different algorithms and tasks. This sensitivity can reduce the robustness and portability of these algorithms. Optimization-based meta-learning methods, in particular, require substantial computational resources and memory, which limits their applicability in resource-constrained environments. Task selection also plays a crucial role in meta-learning; since meta-learning involves learning how to learn, inappropriate source task selection can prevent effective cross-task knowledge transfer and training. These issues suggest that while the future of meta-learning holds great promise, further research and optimization are necessary to overcome the current technical bottlenecks.
Future research should focus on developing more efficient meta-learning algorithms with lower memory demands to meet the needs of resource-constrained environments. Moreover, exploring how meta-learning can accelerate model convergence and how it can facilitate more effective cross-task knowledge transfer across a broader range of tasks and datasets are promising directions for further investigation. Overall, meta-learning, as an emerging machine learning method, still requires ongoing refinement and improvement to fully realize its potential in more complex real-world scenarios.
References
[1]. Vettoruzzo, A., Bouguelia, M.-R., Vanschoren, J., Rognvaldsson, T., & Santosh, K. (2024). Advances and challenges in meta-learning: A technical review. IEEE Transactions on Pattern Analysis and Machine Intelligence, 46(7), 4763–4779. https://doi.org/10.1109 /TPAMI.2024.3357847
[2]. Sherborne, T., & Lapata, M. (2023). Meta-learning a cross-lingual manifold for semantic parsing. Transactions of the Association for Computational Linguistics, 11, 49-67. https://doi.org/10.1162/tacl_a_00533
[3]. Wang, W., Wang, Y., Hoi, S. C. H., & Joty, S. (2023). Towards low-resource automatic program repair with meta-learning and pretrained language models. Proceedings of the 2023 Conference on Empirical Methods in Natural Language Processing, 6954–6968. https://doi.org/10.18653/v1/2023.emnlp-main.598
[4]. Huisman, M., van Rijn, J. N., & Plaat, A. (2021). A survey of deep meta-learning. Artificial Intelligence Review, 54(5), 4483–4541. https://doi.org/10.1007/s10462-021-10004-4
[5]. Jankowski, N., Duch, W., & Grabczewski, K. (2011). Meta-learning in computational intelligence (1st ed. 2011). Springer. https://doi.org/10.1007/978-3-642-20980-2
[6]. Singh, A., Chandrasekar, S., Saha, S., & Sen, T. (2023). Federated meta-learning for emotion and sentiment aware multi-modal complaint identification. Proceedings of the EMNLP 2023.
[7]. Qin, C., Joty, S., Li, Q., & Zhao, R. (2024). Learning to initialize: Can meta-learning improve cross-task generalization in prompt tuning? Proceedings of the Conference on Empirical Methods in Natural Language Processing (EMNLP), 11802–11809.
[8]. Li, X., Song, K., Lin, T., Kang, Y., Zhao, F., Sun, C., & Liu, X. (2024). PDAMeta: Meta-learning framework with progressive data augmentation for few-shot text classification. Proceedings of the LREC-COLING 2024, 12668–12678.
Cite this article
Luo,S. (2024). Applications and Challenges of Meta-Learning in Natural Language Processing. Applied and Computational Engineering,109,1-8.
Data availability
The datasets used and/or analyzed during the current study will be available from the authors upon reasonable request.
Disclaimer/Publisher's Note
The statements, opinions and data contained in all publications are solely those of the individual author(s) and contributor(s) and not of EWA Publishing and/or the editor(s). EWA Publishing and/or the editor(s) disclaim responsibility for any injury to people or property resulting from any ideas, methods, instructions or products referred to in the content.
About volume
Volume title: Proceedings of the 2nd International Conference on Machine Learning and Automation
© 2024 by the author(s). Licensee EWA Publishing, Oxford, UK. This article is an open access article distributed under the terms and
conditions of the Creative Commons Attribution (CC BY) license. Authors who
publish this series agree to the following terms:
1. Authors retain copyright and grant the series right of first publication with the work simultaneously licensed under a Creative Commons
Attribution License that allows others to share the work with an acknowledgment of the work's authorship and initial publication in this
series.
2. Authors are able to enter into separate, additional contractual arrangements for the non-exclusive distribution of the series's published
version of the work (e.g., post it to an institutional repository or publish it in a book), with an acknowledgment of its initial
publication in this series.
3. Authors are permitted and encouraged to post their work online (e.g., in institutional repositories or on their website) prior to and
during the submission process, as it can lead to productive exchanges, as well as earlier and greater citation of published work (See
Open access policy for details).
References
[1]. Vettoruzzo, A., Bouguelia, M.-R., Vanschoren, J., Rognvaldsson, T., & Santosh, K. (2024). Advances and challenges in meta-learning: A technical review. IEEE Transactions on Pattern Analysis and Machine Intelligence, 46(7), 4763–4779. https://doi.org/10.1109 /TPAMI.2024.3357847
[2]. Sherborne, T., & Lapata, M. (2023). Meta-learning a cross-lingual manifold for semantic parsing. Transactions of the Association for Computational Linguistics, 11, 49-67. https://doi.org/10.1162/tacl_a_00533
[3]. Wang, W., Wang, Y., Hoi, S. C. H., & Joty, S. (2023). Towards low-resource automatic program repair with meta-learning and pretrained language models. Proceedings of the 2023 Conference on Empirical Methods in Natural Language Processing, 6954–6968. https://doi.org/10.18653/v1/2023.emnlp-main.598
[4]. Huisman, M., van Rijn, J. N., & Plaat, A. (2021). A survey of deep meta-learning. Artificial Intelligence Review, 54(5), 4483–4541. https://doi.org/10.1007/s10462-021-10004-4
[5]. Jankowski, N., Duch, W., & Grabczewski, K. (2011). Meta-learning in computational intelligence (1st ed. 2011). Springer. https://doi.org/10.1007/978-3-642-20980-2
[6]. Singh, A., Chandrasekar, S., Saha, S., & Sen, T. (2023). Federated meta-learning for emotion and sentiment aware multi-modal complaint identification. Proceedings of the EMNLP 2023.
[7]. Qin, C., Joty, S., Li, Q., & Zhao, R. (2024). Learning to initialize: Can meta-learning improve cross-task generalization in prompt tuning? Proceedings of the Conference on Empirical Methods in Natural Language Processing (EMNLP), 11802–11809.
[8]. Li, X., Song, K., Lin, T., Kang, Y., Zhao, F., Sun, C., & Liu, X. (2024). PDAMeta: Meta-learning framework with progressive data augmentation for few-shot text classification. Proceedings of the LREC-COLING 2024, 12668–12678.