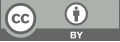
Review on Multisensor SLAM Datasets for Advanced Perception and Mapping Technologies
- 1 Tianjin University, Tianjin, China, 300130
* Author to whom correspondence should be addressed.
Abstract
SLAM (Simultaneous Localization and Mapping) is a technique in robotics and computer vision used to build a map of an unknown environment while simultaneously tracking the location of a robot or vehicle within that environment. The primary goal is to enable autonomous systems to navigate and understand their surroundings without prior knowledge of the environment. It has evolved significantly with the integration of diverse sensor modalities which initially used either a single LIDAR (light detection and ranging, or laser imaging, detection, and ranging) or visual sensor to perform the dual tasks of mapping an environment and localizing the device within it. These systems had limitations in accuracy and robustness due to their reliance on a single type of data input. Over time, the field has advanced to incorporate multiple sensor modalities, including LIDAR, visual cameras, Inertial Measurement Units (IMUs), ultrasonic sensors, and GPS. This multi-sensor fusion approach has dramatically enhanced the precision and reliability of SLAM systems. This paper reviews the state-of-the-art datasets that combine data from infrared cameras, depth cameras, LiDAR, and 4D millimeter-wave radar, focusing on their contributions to advancing SLAM technologies. The study analyzes the advantages and limitations of each sensor type, the challenges associated with data fusion, and the impact on perception and mapping accuracy. This review aims to provide a comprehensive understanding of how these multisensor datasets enhance SLAM systems and highlight areas for future research.
Keywords
SLAM, LIDAR, multisensor.
[1]. Xu, X., Zhang, L., Yang, J., Cao, C., Wang, W., Ran, Y., ... & Luo, M. (2022). A review of multi-sensor fusion slam systems based on 3D LIDAR. Remote Sensing, 14(12), 2835.
[2]. Duan, C., Junginger, S., Huang, J., Jin, K., & Thurow, K. (2019). Deep learning for visual SLAM in transportation robotics: A review. Transportation Safety and Environment, 1(3), 177-184.
[3]. Xie, J., Nashashibi, F., Parent, M. N., & Garcia-Favrot, O. (2010, October). A real-time robust SLAM for large-scale outdoor environments. In 17th ITS world congress (ITSwc'2010) (p. S_EU00913).
[4]. Wang, H., Gao, C., Gao, T., Hu, J., Xu, Z., Han, J., ... & Wu, Y. (2024, June). SLAM in Low-Light Environments Based on Infrared-Visible Light Fusion. In 2024 IEEE 18th International Conference on Control & Automation (ICCA) (pp. 868-873). IEEE.
[5]. Xu, X., Zhang, L., Yang, J., Cao, C., Wang, W., Ran, Y., ... & Luo, M. (2022). A review of multi-sensor fusion slam systems based on 3D LIDAR. Remote Sensing, 14(12), 2835.
[6]. Taylor, T. S. (2019). Introduction to laser science and engineering. CRC Press.
[7]. Shan, J., & Toth, C. K. (Eds.). (2018). Topographic laser ranging and scanning: principles and processing. CRC press.
[8]. Geiger, A., Lenz, P., Stiller, C., & Urtasun, R. (2015). The kitti vision benchmark suite. URL http://www. cvlibs. net/datasets/kitti, 2(5), 1-13.
[9]. Li, X., Zhang, H., & Chen, W. (2023). 4d radar-based pose graph slam with ego-velocity pre-integration factor. IEEE Robotics and Automation Letters.
[10]. Chong, C. Y. (2012). Tracking and data fusion: A handbook of algorithms (bar-shalom, y. et al; 2011)[bookshelf]. IEEE Control Systems Magazine, 32(5), 114-116.
[11]. Wang, X. (2017). Monte Carlo Methods for Statistical Signal Processing. In Mathematical Foundations for Signal Processing, Communications, and Networking (pp. 411-441). CRC Press.
[12]. Fayyad, J., Jaradat, M. A., Gruyer, D., & Najjaran, H. (2020). Deep learning sensor fusion for autonomous vehicle perception and localization: A review. Sensors, 20(15), 4220.
[13]. Zhang, Z. (2000). A flexible new technique for camera calibration. IEEE Transactions on pattern analysis and machine intelligence, 22(11), 1330-1334.
[14]. Jacobson, A., Chen, Z., & Milford, M. (2015). Autonomous Multisensor Calibration and Closed‐loop Fusion for SLAM. Journal of Field Robotics, 32(1), 85-122.
[15]. Owens, J. D., Houston, M., Luebke, D., Green, S., Stone, J. E., & Phillips, J. C. (2008). GPU computing. Proceedings of the IEEE, 96(5), 879-899.
[16]. Sualeh, M., & Kim, G. W. (2020). Visual-LiDAR based 3D object detection and tracking for embedded systems. IEEE Access, 8, 156285-156298.
[17]. You, Y., Wei, P., Cai, J., Huang, W., Kang, R., & Liu, H. (2022). MISD‐SLAM: multimodal semantic SLAM for dynamic environments. Wireless Communications and Mobile Computing, 2022(1), 7600669.
[18]. Wan, G., Yang, X., Cai, R., Li, H., Zhou, Y., Wang, H., & Song, S. (2018, May). Robust and precise vehicle localization based on multi-sensor fusion in diverse city scenes. In 2018 IEEE international conference on robotics and automation (ICRA) (pp. 4670-4677). IEEE.
[19]. Li, Q., Queralta, J. P., Gia, T. N., Zou, Z., & Westerlund, T. (2020). Multi-sensor fusion for navigation and mapping in autonomous vehicles: Accurate localization in urban environments. Unmanned Systems, 8(03), 229-237.
Cite this article
Song,B. (2024). Review on Multisensor SLAM Datasets for Advanced Perception and Mapping Technologies. Applied and Computational Engineering,97,170-174.
Data availability
The datasets used and/or analyzed during the current study will be available from the authors upon reasonable request.
Disclaimer/Publisher's Note
The statements, opinions and data contained in all publications are solely those of the individual author(s) and contributor(s) and not of EWA Publishing and/or the editor(s). EWA Publishing and/or the editor(s) disclaim responsibility for any injury to people or property resulting from any ideas, methods, instructions or products referred to in the content.
About volume
Volume title: Proceedings of the 2nd International Conference on Machine Learning and Automation
© 2024 by the author(s). Licensee EWA Publishing, Oxford, UK. This article is an open access article distributed under the terms and
conditions of the Creative Commons Attribution (CC BY) license. Authors who
publish this series agree to the following terms:
1. Authors retain copyright and grant the series right of first publication with the work simultaneously licensed under a Creative Commons
Attribution License that allows others to share the work with an acknowledgment of the work's authorship and initial publication in this
series.
2. Authors are able to enter into separate, additional contractual arrangements for the non-exclusive distribution of the series's published
version of the work (e.g., post it to an institutional repository or publish it in a book), with an acknowledgment of its initial
publication in this series.
3. Authors are permitted and encouraged to post their work online (e.g., in institutional repositories or on their website) prior to and
during the submission process, as it can lead to productive exchanges, as well as earlier and greater citation of published work (See
Open access policy for details).