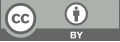
Application of Stable Diffusion and LoRA Models in AI Drawing
- 1 City University of Macau
* Author to whom correspondence should be addressed.
Abstract
This paper explores the application of Stable Diffusion model and LoRA (Low-Rank Adaptation) model in AI-generated artwork. The authors introduce the foundational principles of Stable Diffusion model and LoRA, as well as their application in high-quality image generation. Using three popular datasets — ImageNet, COCO, and CelebA — we apply various image quality assessment metrics, including PSNR (Peak Signal-to-Noise Ratio), IS (Inception Score), and FID (Fréchet Inception Distance), and further validate their potential in artistic creation through subjective evaluations. By comparing the performance of these two models across different datasets, we examine their strengths, weaknesses, areas for improvement in image generation tasks, along with user experience considerations. The experimental results show that the Stable Diffusion model excels in terms of image quality and diversity, while the LoRA model offers significant benefits in computational efficiency and resource usage. Through comprehensive experimental evaluations, this paper provides a scientific basis for model selection in AI art creation and offers insights for the future development of hybrid models.
Keywords
AI Plotting, Stable Diffusion, Diffusion Model, Lora.
[1]. Goodfellow, I., Pouget-Abadie, J., Mirza, M., Xu, B., Warde-Farley, D., Ozair, S., ... & Bengio, Y. (2014). Generative adversarial nets. Advances in neural information processing systems, 27.
[2]. Ho, J., Jain, A., & Abbeel, P. (2020). Denoising diffusion probabilistic models. arXiv preprint arXiv:2006.11239.
[3]. Hu, E. J., Shen, Y., Wallis, P., Allen-Zhu, Z., Li, Y., Wang, L., & Chen, W. (2021). LoRA: Low-Rank Adaptation of Large Language Models. arXiv preprint arXiv:2106.09685.
[4]. Deng, J., Dong, W., Socher, R., Li, L. J., Li, K., & Fei-Fei, L. (2009). Imagenet: A large-scale hierarchical image database. In 2009 IEEE conference on computer vision and pattern recognition (pp. 248-255). Ieee.
[5]. Lin, T. Y., Maire, M., Belongie, S., Hays, J., Perona, P., Ramanan, D., ... & Zitnick, C. L. (2014). Microsoft coco: Common objects in context. In European conference on computer vision (pp. 740-755). Springer, Cham.
[6]. Liu, Z., Luo, P., Wang, X., & Tang, X. (2015). Deep learning face attributes in the wild. In Proceedings of the IEEE international conference on computer vision (pp. 3730-3738).
[7]. Heusel, M., Ramsauer, H., Unterthiner, T., Nessler, B., & Hochreiter, S. (2017). Gans trained by a two time-scale update rule converge to a local nash equilibrium. Advances in neural information processing systems, 30.
[8]. Salimans, T., Goodfellow, I., Zaremba, W., Cheung, V., Radford, A., & Chen, X. (2016). Improved techniques for training gans. Advances in neural information processing systems, 29.
[9]. Wang, Z., Bovik, A. C., Sheikh, H. R., & Simoncelli, E. P. (2004). Image quality assessment: from error visibility to structural similarity. IEEE transactions on image processing, 13(4), 600-612.
[10]. Goodfellow, I., Pouget-Abadie, J., Mirza, M., Xu, B., Warde-Farley, D., Ozair, S., Courville, A., & Bengio, Y. (2014). Generative Adversarial Networks. arXiv preprint arXiv:1406.2661.
Cite this article
Gong,S. (2024). Application of Stable Diffusion and LoRA Models in AI Drawing. Applied and Computational Engineering,97,12-17.
Data availability
The datasets used and/or analyzed during the current study will be available from the authors upon reasonable request.
Disclaimer/Publisher's Note
The statements, opinions and data contained in all publications are solely those of the individual author(s) and contributor(s) and not of EWA Publishing and/or the editor(s). EWA Publishing and/or the editor(s) disclaim responsibility for any injury to people or property resulting from any ideas, methods, instructions or products referred to in the content.
About volume
Volume title: Proceedings of the 2nd International Conference on Machine Learning and Automation
© 2024 by the author(s). Licensee EWA Publishing, Oxford, UK. This article is an open access article distributed under the terms and
conditions of the Creative Commons Attribution (CC BY) license. Authors who
publish this series agree to the following terms:
1. Authors retain copyright and grant the series right of first publication with the work simultaneously licensed under a Creative Commons
Attribution License that allows others to share the work with an acknowledgment of the work's authorship and initial publication in this
series.
2. Authors are able to enter into separate, additional contractual arrangements for the non-exclusive distribution of the series's published
version of the work (e.g., post it to an institutional repository or publish it in a book), with an acknowledgment of its initial
publication in this series.
3. Authors are permitted and encouraged to post their work online (e.g., in institutional repositories or on their website) prior to and
during the submission process, as it can lead to productive exchanges, as well as earlier and greater citation of published work (See
Open access policy for details).