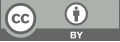
Research on Traffic Flow Prediction Methods Based on Deep Learning
- 1 International College, Wenzhou Business College, Wenzhou, China
- 2 Oulu College, Nanjing Institute of Technology, Nanjing, China
* Author to whom correspondence should be addressed.
Abstract
In recent years, traffic flow prediction technology has been transformed from statistics based parametric methods and machine learning driven non-parametric methods to big data driven deep learning methods. This paper summarizes and summarizes the existing methods and improvement measures of long and short term traffic flow prediction based on deep learning. The time range of traffic flow forecast based on the model is divided into long-term and short term. The short-term traffic flow forecasting methods are subdivided into time series model, non-parametric forecasting model and probability forecasting model, and the advantages and disadvantages of each method and the feasibility of the specific methods are summarized. As for the long-term model, it is mainly based on the application of GCN model to other models, and then the specific methods of its hybrid model are outlined, systematically describing the value of deep learning in traffic flow prediction. Finally, the future research direction and development trend in this field are predicted and prospected.
Keywords
Traffic flow prediction, deep learning, long and short term traffic flow.
[1]. Cui, J. X., Yaojia, & Zhao, B. Y. (2024). A review of short-term traffic flow forecasting methods based on deep learning. Acta Photonica Sinica, 24(2), 5064. doi: 10.19818/j.cnki.16711637.2024.02.003
[2]. Zhu, S. L., Cheng, L., & Chu, Z. M. (2013). Bayesian network model for traffic flow estimation using prior link flows. Journal of Southeast University (English Edition), 29(3), 322327.
[3]. Liu, Z., Du, W., Yan, D. M., et al. (2017). Short term traffic flow forecast based on K nearest neighbor algorithm and support vector regression. Journal of Highway and Transportation Research and Development, 34(5), 122128.
[4]. Tan, M. C., Feng, L. B., & Xu, J. M. (2007). Traffic flow prediction based on hybrid ARIMA and ANN model. China Journal of Highway and Transport, 20(4), 118121. doi: 10.3321/j.issn:10017372.2007.04.021
[5]. Yin, L. S., Tang, S. Q., Li, S., & He, Y. G. (2019). Traffic flow prediction based on hybrid model of autoregressive integrated moving average and genetic particle swarm optimization wavelet neural network. Journal of Electronics & Information Technology, 41(9), 22732279. doi: 10.11999/JEIT181073
[6]. Guo, H. F., Fang, L. J., & Yu, L. (2013). short-term traffic flow prediction based on fuzzy kalman filter method. Journal of Zhejiang University of Technology, 41(2), 4. DOI: 10.3969/j.issn.10064303.2013.02.022
[7]. Lin, C. (2015). Based on K nearest neighbor nonparametric regression of short-term traffic flow prediction algorithm research. University of Electronic Science and Technology. The DOI: 10.7666/d.D.664651
[8]. Lyu, Y. S., Duany, Y. J., Kang, W. W., et al. (2015). Traffic flow prediction with big data: a deep learning approach. IEEE Transactions on Intelligent Transportation Systems, 16(2), 865873.
[9]. Ge, L., Li, S. Y., Wang, Y. Q., et al. (2020). Global spatialtemporal graph convolutional network for urban traffic speed prediction. Applied Sciences, 10(4), 1509. doi: 10.3390/app10041509
[10]. Lin, H. X., Jia, W. J., Sun, Y. P., et al. (2019). Spatialtemporal selfattention network for flow prediction. arXiv preprint arXiv:1912.07663.
[11]. Guo, S. N., Lin, Y. F., Li, S. J., et al. (2019). Deep spatialtemporal 3D convolutional neural networks for traffic data forecasting. IEEE Transactions on Intelligent Transportation Systems, 20(10), 39133926.
[12]. Sun, X. H., & Liu, T. Q. (2008). short-term traffic flow forecasting based on a hybrid neural network model and SARIMA model. Journal of Transportation Systems Engineering and Information Technology, 8(5), 3237.
[13]. Yu, B., Yin, H., & Zhu, Z. (2017). spatio-temporal graph convolutional networks: A deep learning framework for traffic forecasting. arXiv preprint arXiv:1709.04875.
[14]. Liu, Y., & Gu, Y. (2022). Prediction of spatio-temporal characteristics of highway traffic flow based on CNNBiLSTM. Transportation Science and Technology and Economy, 24(1), 918.
[15]. Wan, S. X., Lan, Y. Y., Guo, J. F., et al. (2017). Localized bidirectional long short-term memory for text classification. Journal of Chinese Information Science, 31(3), 7.
[16]. Huang, W., Song, G., Hong, H., & Xie, K. (2018). Deep Architecture for Traffic Flow Prediction: Combining Convolutional Neural Networks and Bidirectional Long Short-Term Memory Networks. IEEE Transactions on Intelligent Transportation Systems, 19(5), 1447-1457. https://doi.org/10.1109/TITS.2017.2766864
Cite this article
Chen,J.;Song,J. (2024). Research on Traffic Flow Prediction Methods Based on Deep Learning. Applied and Computational Engineering,111,72-80.
Data availability
The datasets used and/or analyzed during the current study will be available from the authors upon reasonable request.
Disclaimer/Publisher's Note
The statements, opinions and data contained in all publications are solely those of the individual author(s) and contributor(s) and not of EWA Publishing and/or the editor(s). EWA Publishing and/or the editor(s) disclaim responsibility for any injury to people or property resulting from any ideas, methods, instructions or products referred to in the content.
About volume
Volume title: Proceedings of CONF-MLA 2024 Workshop: Mastering the Art of GANs: Unleashing Creativity with Generative Adversarial Networks
© 2024 by the author(s). Licensee EWA Publishing, Oxford, UK. This article is an open access article distributed under the terms and
conditions of the Creative Commons Attribution (CC BY) license. Authors who
publish this series agree to the following terms:
1. Authors retain copyright and grant the series right of first publication with the work simultaneously licensed under a Creative Commons
Attribution License that allows others to share the work with an acknowledgment of the work's authorship and initial publication in this
series.
2. Authors are able to enter into separate, additional contractual arrangements for the non-exclusive distribution of the series's published
version of the work (e.g., post it to an institutional repository or publish it in a book), with an acknowledgment of its initial
publication in this series.
3. Authors are permitted and encouraged to post their work online (e.g., in institutional repositories or on their website) prior to and
during the submission process, as it can lead to productive exchanges, as well as earlier and greater citation of published work (See
Open access policy for details).