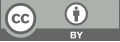
Quantum Ant Colony Algorithm for Solving the Traveling Salesman Problem: A Theoretical and Practical Analysis
- 1 School of Computer and Control Engineering, Yantai University, YanTai, China
* Author to whom correspondence should be addressed.
Abstract
The quantum ant colony algorithm (QACO) is explored as a solution to the traveling salesman problem (TSP), targeting inefficiencies such as slow convergence and local optima entrapment found in traditional ant colony optimization (ACO) methods. By integrating quantum computing elements, specifically quantum rotation gates and qubits, into the ACO framework, QACO demonstrates enhanced efficiency and faster convergence. This paper delves into the foundational principles of quantum computing and their application to refine ACO, effectively solving the TSP. An in-depth analysis is provided, showcasing QACO's advantages over traditional ACO through empirical examples. These examples illustrate QACO's potential to improve path planning for applications within the rapidly growing e-commerce and logistics sectors. The integration of quantum mechanisms facilitates a more dynamic pheromone update process, which significantly reduces the likelihood of premature convergence and enhances solution quality in complex optimization scenarios. This advancement indicates a promising direction for future research in algorithmic optimization in both theoretical and practical applications.
Keywords
TSP, Quantum computing, ACO
[1]. Wu, L., Huang, X., Cui, J., Liu, C., & Xiao, W. (2023). Modified adaptive ant colony optimization algorithm and its application for solving path planning of mobile robot. Expert Systems with Applications, 215, 119410.
[2]. Li, Y., Zhao, J., & Zhang, Y. (2009). An improved quantum ant colony algorithm for solving TSP. Computer Engineering and Design, 16, 3843-3845+3874.
[3]. de Andoin, M. G., & Echanobe, J. (2022). Implementable hybrid quantum ant colony optimization algorithm. Quantum Machine Intelligence.
[4]. Chen, Z., Zheng, X., Zhou, S., Liu, C., & Chen, H. (2019). Quantum-inspired ant colony optimization algorithm for a two-stage permutation flow shop with batch processing machines. International Journal of Production Research, 58(19), 5945–5963.
[5]. Skinderowicz, R. (2022). Improving Ant Colony Optimization efficiency for solving large TSP instances. Applied Soft Computing (prepublish), 108653.
[6]. Shi, K., Huang, L., Jiang, D., Sun, Y., Tong, X., Xie, Y., & Fang, Z. (2022). Path planning optimization of intelligent vehicle based on improved genetic and ant colony hybrid algorithm. Frontiers in Bioengineering and Biotechnology, 10, 905983.
[7]. Zhu, X., Zhang, Y., Zhao, Z., & Zuo, J. (2019, July). Radio frequency sensing based environmental monitoring technology. In Fourth International Workshop on Pattern Recognition (Vol. 11198, pp. 187-191). SPIE.
[8]. Zhang, Y., Xu, H., Zhu, X. et al. Detection and Quantization Technique of Optical Distributed Acoustic Coupling Based on φ-OTDR. J. Shanghai Jiaotong Univ. (Sci.) 25, 208–213 (2020).
[9]. Zhang, Y., Zhao, H., Zhu, X., Zhao, Z., & Zuo, J. (2019, October). Strain Measurement Quantization Technology based on DAS System. In 2019 IEEE 3rd Advanced Information Management, Communicates, Electronic and Automation Control Conference (IMCEC) (pp. 214-218). IEEE.
[10]. Toaza, B., & Esztergár-Kiss, D. (2023). A review of metaheuristic algorithms for solving TSP-based scheduling optimization problems. Applied Soft Computing, 110908.
[11]. Trust, T., Nyamugure, P., Kumar, S., & Munapo, E. (2023). A labelling method for the Travelling Salesman Problem. Applied Sciences, 11.
[12]. Bi, Z., Yu, X., Wang, B., Huang, W., Zhang, D., & Dong, Z. (2024). Fast fault location technology for distribution network based on quantum ant colony algorithm. Journal of Shanghai Jiaotong University, 58(5), 693.
[13]. Mei, P., Ding, G., Jin, Q., Zhang, F., & Chen, Y. C. (2021). Reconstruction and optimization of complex network community structure under deep learning and quantum ant colony optimization algorithm. Intelligent Automation & Soft Computing, 27(1).
[14]. Zhu, X., Zhao, Z., Wei, X., Wang, X., & Zuo, J. (2021, February). Action recognition method based on wavelet transform and neural network in wireless network. In Proceedings of the 2021 5th International Conference on Digital Signal Processing (pp. 60-65).
[15]. Wang, R., Zhu, J., Wang, S., Wang, T., Huang, J., & Zhu, X. (2024). Multi-modal emotion recognition using tensor decomposition fusion and self-supervised multi-tasking. International Journal of Multimedia Information Retrieval, 13(4), 39.
[16]. Mohsin, S. A., Younes, A., & Darwish, S. M. (2021). Dynamic cost ant colony algorithm to optimize query for distributed database based on quantum-inspired approach. Symmetry, 13(1), 70.
[17]. Chen, Z., Zheng, X., Zhou, S., Liu, C., & Chen, H. (2019). Quantum-inspired ant colony optimisation algorithm for a two-stage permutation flow shop with batch processing machines. International Journal of Production Research, 58(19), 5945–5963.
[18]. Li, F., Liu, M., & Xu, G. (2019). A quantum ant colony multi-objective routing algorithm in WSN and its application in a manufacturing environment. Sensors, 19(15), 3334.
[19]. An, J., Liu, X., He, M., & Song, H. (2022). Overview of quantum group intelligence optimization algorithms. Computer Engineering and Applications, 07, 31-42.
Cite this article
Li,Y. (2024). Quantum Ant Colony Algorithm for Solving the Traveling Salesman Problem: A Theoretical and Practical Analysis. Applied and Computational Engineering,110,175-181.
Data availability
The datasets used and/or analyzed during the current study will be available from the authors upon reasonable request.
Disclaimer/Publisher's Note
The statements, opinions and data contained in all publications are solely those of the individual author(s) and contributor(s) and not of EWA Publishing and/or the editor(s). EWA Publishing and/or the editor(s) disclaim responsibility for any injury to people or property resulting from any ideas, methods, instructions or products referred to in the content.
About volume
Volume title: Proceedings of CONF-MLA 2024 Workshop: Securing the Future: Empowering Cyber Defense with Machine Learning and Deep Learning
© 2024 by the author(s). Licensee EWA Publishing, Oxford, UK. This article is an open access article distributed under the terms and
conditions of the Creative Commons Attribution (CC BY) license. Authors who
publish this series agree to the following terms:
1. Authors retain copyright and grant the series right of first publication with the work simultaneously licensed under a Creative Commons
Attribution License that allows others to share the work with an acknowledgment of the work's authorship and initial publication in this
series.
2. Authors are able to enter into separate, additional contractual arrangements for the non-exclusive distribution of the series's published
version of the work (e.g., post it to an institutional repository or publish it in a book), with an acknowledgment of its initial
publication in this series.
3. Authors are permitted and encouraged to post their work online (e.g., in institutional repositories or on their website) prior to and
during the submission process, as it can lead to productive exchanges, as well as earlier and greater citation of published work (See
Open access policy for details).