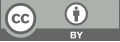
Research on Image Feature Extraction Based on Convolutional Neural Network
- 1 College of Engineering, Shantou University, Shantou, China
* Author to whom correspondence should be addressed.
Abstract
This article explores the impact of various hyperparameters on the performance of image feature extraction using convolutional neural networks (CNNs), with a focus on learning rate, dropout rate, batch size, and the number of epochs. Using the CIFAR-10 dataset, extensive experiments were conducted to optimize these parameters, aiming to achieve high accuracy while avoiding overfitting. The findings underscore the importance of carefully selecting these hyperparameters to balance training efficiency and model performance. Through a rigorous analysis of the effects of these hyperparameters on model performance under various configurations, including training accuracy, test accuracy, training loss, and test loss, our experimental results indicate that the model achieves optimal performance with a learning rate of 0.0001 and a dropout rate of 0.5. The model demonstrates optimal performance in avoiding overfitting when the number of training epochs is set to 10. Additionally, although batch size has a relatively minor effect on overall model optimization, a slight improvement in performance was observed when the batch size was set to 32.
Keywords
Convolutional Neural Networks(CNNs), Image Feature Extraction, Hyperparameters, Learning Rate, Dropout Rate.
[1]. Amir Ghasem, Nasrin Bayat, Fatemeh Mottaghian & Akram Bayat (2023). Improving Performance of Object Detection Using the Mechanisms of Visual Recognition in Humans. arXiv preprint, 2301.09667.Retrieved from https://doi.org/10.48550/arXiv.2301.09667
[2]. Siqi Lv, Wangli He, Feng Qian&Jinde Cao(2018). Leaderless synchronization of coupled neural networks with the event-triggered mechanism. Neural Networks, 105, 316-327.Retrieved from https://doi.org/10.1016/j.neunet.2018.05.012
[3]. Simonyan, K., & Zisserman, A. (2015). Very Deep Convolutional Networks for Large-Scale Image Recognition. International Conference on Learning Representations (ICLR). Retrieved from https://doi.org/10.48550/arXiv.1409.1556.
[4]. Y. Bengio, P. Simard, & P. Frasconi (1994). "Learning Long-Term Dependencies with Gradient Descent is Difficult." IEEE Transactions on Neural Networks, 5(2), 157-166.Retrieved from https://ieeexplore.ieee.org/document/279181
[5]. Loshchilov, I., & Hutter, F. (2017). "SGDR: Stochastic Gradient Descent with Warm Restarts." In Proceedings of the International Conference on Learning Representations (ICLR). Retrieved from https://doi.org/10.48550/arXiv.1608.03983
[6]. Kaichao You, Mingsheng Long, Jianmin Wang, & Michael I. Jordan.(2019). How Does Learning Rate Decay Help Modern Neural Networks?
[7]. Smith, L. N. (2017). Cyclical Learning Rates for Training Neural Networks. In Proceedings of the IEEE Conference on Computer Vision and Pattern Recognition (CVPR) (pp. 184-190). https://ieeexplore.ieee.org/abstract/document/7926641
[8]. Srivastava, N., Hinton, G. E., Krizhevsky, A., Sutskever, I., & Salakhutdinov, R. (2014). Dropout: A technique to prevent overfitting in neural networks. Journal of Machine Learning Research, 15, 1929-1958. http://www.jmlr.org/papers/volume15/srivastava14a/srivastava14a.pdf
[9]. Zhang, Y. (2018). Impact of dropout rates on overfitting and model training. International Journal of Machine Learning and Computing, 8(5), 478-484. https://doi.org/10.18178/ijmlc.2018.8.5.686
[10]. Li, Q., & Ke, W. (2024). Investigating the Synergistic Effects of Dropout and Residual Connections on Language Model Training. https://arxiv.org/pdf/2410.01019
[11]. Srivastava, N., Hinton, G., Krizhevsky, A., Sutskever, I., & Salakhutdinov, R. (2014). Dropout: A simple way to prevent neural networks from overfitting. *Journal of Machine Learning Research,15*,1929-1958.https://www.jmlr.org/papers/volume15/srivastava14a/srivastava14a.pdf
[12]. Ajayi, O. G., & Ashi, J. (2023). Effect of varying training epochs of a Faster Region-Based Convolutional Neural Network on the Accuracy of an Automatic Weed Classification Scheme. Smart Agricultural Technology, 3, 100128. https://www.sciencedirect.com/science/article/pii/S2772375522000934
[13]. Keskar, N. S., Mudigere, D., Nocedal, J., Smelyanskiy, M., & Tang, P. T. P. (2016). On Large-Batch Training for Deep Learning: Generalization Gap and Sharp Minima. arXiv preprint arXiv:1609.04836. https://arxiv.org/abs/1609.04836
[14]. Wilson, D. R., & Martinez, T. R. (2003). The general inefficiency of batch training for gradient descent learning. Neural Networks, 16(10), 1429-1451. https://www.sciencedirect.com/science/article/abs/pii/S0893608003001382?via%3Dihub
[15]. Santurkar, S., Tsipras, D., Ilyas, A., & Madry, A. (2019). “How Does Batch Normalization Help Optimization?” arXiv preprint arXiv: 1805.11604. Retrieved from https://arxiv.org/abs/1805.11604
Cite this article
Xiang,S. (2024). Research on Image Feature Extraction Based on Convolutional Neural Network. Applied and Computational Engineering,107,24-33.
Data availability
The datasets used and/or analyzed during the current study will be available from the authors upon reasonable request.
Disclaimer/Publisher's Note
The statements, opinions and data contained in all publications are solely those of the individual author(s) and contributor(s) and not of EWA Publishing and/or the editor(s). EWA Publishing and/or the editor(s) disclaim responsibility for any injury to people or property resulting from any ideas, methods, instructions or products referred to in the content.
About volume
Volume title: Proceedings of the 2nd International Conference on Machine Learning and Automation
© 2024 by the author(s). Licensee EWA Publishing, Oxford, UK. This article is an open access article distributed under the terms and
conditions of the Creative Commons Attribution (CC BY) license. Authors who
publish this series agree to the following terms:
1. Authors retain copyright and grant the series right of first publication with the work simultaneously licensed under a Creative Commons
Attribution License that allows others to share the work with an acknowledgment of the work's authorship and initial publication in this
series.
2. Authors are able to enter into separate, additional contractual arrangements for the non-exclusive distribution of the series's published
version of the work (e.g., post it to an institutional repository or publish it in a book), with an acknowledgment of its initial
publication in this series.
3. Authors are permitted and encouraged to post their work online (e.g., in institutional repositories or on their website) prior to and
during the submission process, as it can lead to productive exchanges, as well as earlier and greater citation of published work (See
Open access policy for details).