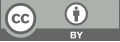
Exploration of the Application of Multimodal Model in Psychological Analysis
- 1 School of International Digital Economy, Minjiang University, Fuzhou, China
* Author to whom correspondence should be addressed.
Abstract
Multimodal sentiment analysis is one of the important research areas in the field of artificial intelligence today. Multimodal sentiment analysis is to extract features from various human modalities such as facial expressions, body movements, and voice information, perform modal fusion, and finally classify and predict emotions. This technology can be used in multiple scenarios such as stock prediction, product analysis, movie box office prediction, etc., especially psychological state analysis, and has important research significance. This paper introduces two important datasets in multimodal sentiment analysis, namely CMU-MOSEI and IEMOCAP. It also introduces the feature-level fusion, model-level fusion, decision-level fusion and other fusion methods in multimodal fusion methods, and also introduces the semantic feature fusion neural network and sentiment word perception fusion network in multimodal sentiment analysis related models. Finally, the application of multimodal sentiment analysis models in depression and other related mental illnesses and the challenges of multimodal sentiment analysis models in the future are introduced. This paper hopes that the above research will be helpful for multimodal sentiment analysis.
Keywords
Multimodal Model, Modal Fusion, Psychological Analysis
[1]. World Health Organization. (2021). Comprehensive mental health action plan 2013–2030. World Health Organization.
[2]. Zhu, T., Li, L., Yang, J., Zhao, S., Liu, H., & Qian, J. (2022). Multimodal sentiment analysis with image-text interaction network. IEEE transactions on multimedia, 25, 3375-3385.
[3]. Han, W., Chen, H., Gelbukh, A., Zadeh, A., Morency, L. P., & Poria, S. (2021, October). Bi-bimodal modality fusion for correlation-controlled multimodal sentiment analysis. In Proceedings of the 2021 international conference on multimodal interaction (pp. 6-15).
[4]. Huang, F., Zhang, X., Zhao, Z., Xu, J., & Li, Z. (2019). Image–text sentiment analysis via deep multimodal attentive fusion. Knowledge-Based Systems, 167, 26-37.
[5]. Li, Q., Gkoumas, D., Lioma, C., & Melucci, M. (2021). Quantum-inspired multimodal fusion for video sentiment analysis. Information Fusion, 65, 58-71.
[6]. Zadeh, A., Chen, M., Poria, S., Cambria, E., & Morency, L. P. (2017). Tensor fusion network for multimodal sentiment analysis. arXiv preprint arXiv:1707.07250.
[7]. Liu, Z., Shen, Y., Lakshminarasimhan, V. B., Liang, P. P., Zadeh, A., & Morency, L. P. (2018). Efficient low-rank multimodal fusion with modality-specific factors. arXiv preprint arXiv:1806.00064.
[8]. Fukui, A., Park, D. H., Yang, D., Rohrbach, A., Darrell, T., & Rohrbach, M. (2016). Multimodal compact bilinear pooling for visual question answering and visual grounding. arXiv preprint arXiv:1606.01847.
[9]. Poria, S., Chaturvedi, I., Cambria, E., & Hussain, A. (2016, December). Convolutional MKL based multimodal emotion recognition and sentiment analysis. In 2016 IEEE 16th international conference on data mining (ICDM) (pp. 439-448). IEEE.
[10]. Nie, W., Yan, Y., Song, D., & Wang, K. (2021). Multi-modal feature fusion based on multi-layers LSTM for video emotion recognition. Multimedia Tools and Applications, 80, 16205-16214.
[11]. Aziz, R. H. H., & Dimililer, N. (2020, December). Twitter sentiment analysis using an ensemble weighted majority vote classifier. In 2020 International Conference on Advanced Science and Engineering (ICOASE) (pp. 103-109). IEEE.
[12]. Wang, H., Meghawat, A., Morency, L. P., & Xing, E. P. (2017, July). Select-additive learning: Improving generalization in multimodal sentiment analysis. In 2017 IEEE International Conference on Multimedia and Expo (ICME) (pp. 949-954). IEEE.
[13]. Ma, F., Sun, B., & Li, S. (2021). Facial expression recognition with visual transformers and attentional selective fusion. IEEE Transactions on Affective Computing, 14(2), 1236-1248.
[14]. Wu, W., Wang, Y., Xu, S., & Yan, K. (2020, September). SFNN: semantic features fusion neural network for multimodal sentiment analysis. In 2020 5th International
[15]. Chen, M., & Li, X. (2020, December). Swafn: Sentimental words aware fusion network for multimodal sentiment analysis. In Proceedings of the 28th international conference on computational linguistics (pp. 1067-1077).
[16]. Busso, C., Bulut, M., Lee, C. C., Kazemzadeh, A., Mower, E., Kim, S., ... & Narayanan, S. S. (2008). IEMOCAP: Interactive emotional dyadic motion capture database. Language resources and evaluation, 42, 335-359.
[17]. Zadeh, A. B., Liang, P. P., Poria, S., Cambria, E., & Morency, L. P. (2018, July). Multimodal language analysis in the wild: Cmu-mosei dataset and interpretable dynamic fusion graph. In Proceedings of the 56th Annual Meeting of the Association for Computational Linguistics (Volume 1: Long Papers) (pp. 2236-2246).
[18]. Zhou, L., Liu, Z., Shangguan, Z., Yuan, X., Li, Y., & Hu, B. (2022). TAMFN: time-aware attention multimodal fusion network for depression detection. IEEE Transactions on Neural Systems and Rehabilitation Engineering, 31, 669-679.
[19]. Golovanevsky, M., Eickhoff, C., & Singh, R. (2022). Multimodal attention-based deep learning for Alzheimer’s disease diagnosis. Journal of the American Medical Informatics Association, 29(12), 2014-2022.
Cite this article
Wang,W. (2024). Exploration of the Application of Multimodal Model in Psychological Analysis. Applied and Computational Engineering,112,115-122.
Data availability
The datasets used and/or analyzed during the current study will be available from the authors upon reasonable request.
Disclaimer/Publisher's Note
The statements, opinions and data contained in all publications are solely those of the individual author(s) and contributor(s) and not of EWA Publishing and/or the editor(s). EWA Publishing and/or the editor(s) disclaim responsibility for any injury to people or property resulting from any ideas, methods, instructions or products referred to in the content.
About volume
Volume title: Proceedings of the 5th International Conference on Signal Processing and Machine Learning
© 2024 by the author(s). Licensee EWA Publishing, Oxford, UK. This article is an open access article distributed under the terms and
conditions of the Creative Commons Attribution (CC BY) license. Authors who
publish this series agree to the following terms:
1. Authors retain copyright and grant the series right of first publication with the work simultaneously licensed under a Creative Commons
Attribution License that allows others to share the work with an acknowledgment of the work's authorship and initial publication in this
series.
2. Authors are able to enter into separate, additional contractual arrangements for the non-exclusive distribution of the series's published
version of the work (e.g., post it to an institutional repository or publish it in a book), with an acknowledgment of its initial
publication in this series.
3. Authors are permitted and encouraged to post their work online (e.g., in institutional repositories or on their website) prior to and
during the submission process, as it can lead to productive exchanges, as well as earlier and greater citation of published work (See
Open access policy for details).