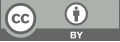
Deep learning for comment spam and scams
- 1 BASIS Bilingual School, Shenzhen, China
* Author to whom correspondence should be addressed.
Abstract
As the popularity of online social networks has increased, so has the manner in which people purchase online. Online reviews on the purchasing of items or the provision of services have become the primary source of user opinion. When a substantial body of knowledge assesses a product or service, that body's effect on the market is significant. Because of this, concerns have been raised among manufacturers and merchants, who frequently compose rubbish reviews to promote or denigrate the quality of specific items or services in order to make a profit or maintain their reputation. You may choose to promote or denigrate certain products or services that have been targeted. This kind of commentary is known as "trash remark." Comment spam violates the interests of enterprises to a significant level, and it possesses a defensive mechanism that combines the "Deepfake" of artificial intelligence technology, which renders conventional security measures inapplicable. Deep learning is the approach that will be most effective in resolving this issue. Researchers assess this research according on how they extract features from the commentary dataset and how they extract features from the commentary dataset, employing a variety of methodologies and strategies to find solutions to the problems. The authors also looked at the key deep learning technologies that have been suggested as a solution to the issue of spam detection in the primary machine, as well as the performance of various deep learning technologies. At the same time, the fact that there is not a lot of spam online review data makes this sort of research a highly significant one. The purpose of this article is to offer an insightful and all-encompassing analysis of comparative research on the topic of spam detection that has recently been conducted.
Keywords
Machine learning, Deep learning Big data, Deepfake, Social spam, Spambot, Spammer.
[1]. Crawford, M., Khoshgoftaar, T. M., Prusa, J. D., Richter, A. N., & Al Najada, H. (2015). Survey of review spam detection using machine learning techniques. Journal of Big Data, 2(1), 1-24.
[2]. Rao, S., Verma, A. K., & Bhatia, T. (2021). A review on social spam detection: challenges, open issues, and future directions. Expert Systems with Applications, 186, 115742.
[3]. Hussain, N., Turab Mirza, H., Rasool, G., Hussain, I., & Kaleem, M. (2019). Spam review detection techniques: A systematic literature review. Applied Sciences, 9(5), 987.
[4]. Asghar, M. Z., Ullah, A., Ahmad, S., & Khan, A. (2020). Opinion spam detection framework using hybrid classification scheme. Soft computing, 24(5), 3475-3498.
[5]. Alom, Z., Carminati, B., & Ferrari, E. (2020). A deep learning model for Twitter spam detection. Online Social Networks and Media, 18, 100079.
[6]. You, L., Peng, Q., Xiong, Z., He, D., Qiu, M., & Zhang, X. (2020). Integrating aspect analysis and local outlier factor for intelligent review spam detection. Future Generation Computer Systems, 102, 163-172.
[7]. Jain, G., Sharma, M., & Agarwal, B. (2019). Optimizing semantic LSTM for spam detection. International Journal of Information Technology, 11(2), 239-250.
[8]. Granik, M., & Mesyura, V. (2017, May). Fake news detection using naive Bayes classifier. In 2017 IEEE first Ukraine conference on electrical and computer engineering (UKRCON) (pp. 900-903). IEEE.
[9]. Xue, H., Li, F., Seo, H., & Pluretti, R. (2015, August). Trust-aware review spam detection. In 2015 IEEE Trustcom/BigDataSE/ISPA (Vol. 1, pp. 726-733). IEEE.
[10]. Chakraborty, M., Pal, S., Pramanik, R., & Chowdary, C. R. (2016). Recent developments in social spam detection and combating techniques: A survey. Information Processing & Management, 52(6), 1053-1073.
Cite this article
Liu,J. (2023). Deep learning for comment spam and scams. Applied and Computational Engineering,6,18-23.
Data availability
The datasets used and/or analyzed during the current study will be available from the authors upon reasonable request.
Disclaimer/Publisher's Note
The statements, opinions and data contained in all publications are solely those of the individual author(s) and contributor(s) and not of EWA Publishing and/or the editor(s). EWA Publishing and/or the editor(s) disclaim responsibility for any injury to people or property resulting from any ideas, methods, instructions or products referred to in the content.
About volume
Volume title: Proceedings of the 3rd International Conference on Signal Processing and Machine Learning
© 2024 by the author(s). Licensee EWA Publishing, Oxford, UK. This article is an open access article distributed under the terms and
conditions of the Creative Commons Attribution (CC BY) license. Authors who
publish this series agree to the following terms:
1. Authors retain copyright and grant the series right of first publication with the work simultaneously licensed under a Creative Commons
Attribution License that allows others to share the work with an acknowledgment of the work's authorship and initial publication in this
series.
2. Authors are able to enter into separate, additional contractual arrangements for the non-exclusive distribution of the series's published
version of the work (e.g., post it to an institutional repository or publish it in a book), with an acknowledgment of its initial
publication in this series.
3. Authors are permitted and encouraged to post their work online (e.g., in institutional repositories or on their website) prior to and
during the submission process, as it can lead to productive exchanges, as well as earlier and greater citation of published work (See
Open access policy for details).