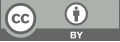
Framework for Lung CT Image Segmentation Based on UNet++
- 1 Cardiff University
- 2 Northeastern University
- 3 Florida College of Integrative Medicine
* Author to whom correspondence should be addressed.
Abstract
Recently, the state-of-art models for medical image segmentation is U-Net and their variants. These networks, though succeeding in deriving notable results, ignore the practical problem hanging over the medical segmentation field: overfitting and small dataset. The over- complicated deep neural networks unnecessarily extract meaningless information, and a majority of them are not suitable for lung slice CT image segmentation task. To overcome the two limitations, we proposed a new whole-process network merging advanced UNet++ model. The network comprises three main modules: data augmentation, optimized neural network, parameter fine-tuning. By incorporating diverse methods, the training results demonstrate a significant advantage over similar works, achieving leading accuracy of 98.03% with the lowest overfitting. potential. Our network is remarkable as one of the first to target on lung slice CT images.
Keywords
Lung segmentation, medical image segmentation, UNet++, overfitting
[1]. Atun R, et al. (2015). “Expanding global access to radiotherapy.” The Lancet Oncology, 16(10), pp. 1153–86. https://doi.org/10.1016/S1470-2045(15)00222-3
[2]. Long J, Shelhamer E and Darrell T (2015). “Fully convolutional networks for semantic segmentation.” Proc. IEEE Conf. Comput. Vis. Pattern Recognit., pp. 3431–40. https://doi.org/10.1109/CVPR.2015.7298965
[3]. Ronneberger O, Fischer P, Brox T (2015). “UNet: convolutional networks for biomedical image segmentation.” MICCAI 2015. LNCS, vol. 9351, Navab N, et al. (Cham: Springer), pp. 234–41. https://doi.org/10.1007/978-3-319-24574-4 28
[4]. M Drozdzal, E Vorontsov, G Chartrand, S Kadoury and C Pal (2016). “The importance of skip connections in biomedical image segmentation.” Deep Learning and Data Labeling for Medical Applications, vol. 10008, Carneiro G, et al. (Cham: Springer), pp. 179–87. https://doi.org/10.1007/978-3-319-46976-8_19
[5]. B Hariharan, P Arbelaez, R Girshick and J Malik (2015). “Hypercolumns for object segmentation and fine-grained localization.” Proc. IEEE Conf. Comput. Vis. Pattern Recognit., pp. 447–56. https://doi.org/10.1109/CVPR.2015.7298690
[6]. Yuan Y, et al (2024). Deep learning for medical image segmentation, in Machine Learning and Artificial Intelligence in Radiation Oncology, J. Kang, et al. (Amsterdam: Academic Press), chapter 5, pp. 107-135.
[7]. Oktay O, et al. (2018). “Attention UNet: learning where to look for the pancreas.” Preprint at arXiv:1804.03999
[8]. Ibtehaz N and Rahman M S (2020). “MultiResUNet: rethinking the UNet architecture for multimodal biomedical image segmentation.” Neural Netw., 121, pp. 74–87. https://doi.org/10.1016/j.neunet.2020.05.012
[9]. Z W Zhou, M M R Siddiquee, N Tajbakhsh and J M Liang (2018). “UNet++: A Nested UNet Architecture for Medical Image Segmentation.” Deep Learning in Medical Image Anylysis and Multimodal Learning for Clinical Decision Support, pp: 3–11. https://doi.org/10.1007/978-3-030-00889-5_1
[10]. M Z Khan, M K Gajendran, Y Lee and M A Khan (2021). “Deep Neural Architectures for Medical Image Semantic Segmentation: Review.” IEEE Access, 9, pp. 83002–24. https://doi.org/10.1109/ACCESS.2021.3086530
[11]. Isensee F, Kohl Simon A A, Petersen Jens, Maier-Hein Klaus H (2021). “nnU-Net: a self-configuring method for deep learning-based biomedical image segmentation.” Nature Methods. 18(2), pp. 203–11. https://doi.org/10.1038/s41592-020-01008-z
[12]. Weng Y, Zhou T, Li Y, Qiu X (2019). “Nas-unet: neural architecture search for medical image segmentation.” IEEE Access, 7, pp. 44247–57. https://doi.org/10.1109/access.2019.2902457
[13]. Luc P, Couprie C, Chintala S, Verbeek J (2016). “Semantic segmentation using adversarial networks.” Preprint at arXiv:161108408
[14]. Singh V K, et al. (2020). “Breast tumor segmentation and shape classification in mammograms using generative adversarial and convolutional neural net-work.” Expert Syst. Appl, 139, p. 112855. https://doi.org/10.1016/j.eswa.2019.112855
Cite this article
Hao,Z.;Zhang,J.;Li,L. (2025). Framework for Lung CT Image Segmentation Based on UNet++. Applied and Computational Engineering,133,1-7.
Data availability
The datasets used and/or analyzed during the current study will be available from the authors upon reasonable request.
Disclaimer/Publisher's Note
The statements, opinions and data contained in all publications are solely those of the individual author(s) and contributor(s) and not of EWA Publishing and/or the editor(s). EWA Publishing and/or the editor(s) disclaim responsibility for any injury to people or property resulting from any ideas, methods, instructions or products referred to in the content.
About volume
Volume title: Proceedings of the 5th International Conference on Signal Processing and Machine Learning
© 2024 by the author(s). Licensee EWA Publishing, Oxford, UK. This article is an open access article distributed under the terms and
conditions of the Creative Commons Attribution (CC BY) license. Authors who
publish this series agree to the following terms:
1. Authors retain copyright and grant the series right of first publication with the work simultaneously licensed under a Creative Commons
Attribution License that allows others to share the work with an acknowledgment of the work's authorship and initial publication in this
series.
2. Authors are able to enter into separate, additional contractual arrangements for the non-exclusive distribution of the series's published
version of the work (e.g., post it to an institutional repository or publish it in a book), with an acknowledgment of its initial
publication in this series.
3. Authors are permitted and encouraged to post their work online (e.g., in institutional repositories or on their website) prior to and
during the submission process, as it can lead to productive exchanges, as well as earlier and greater citation of published work (See
Open access policy for details).