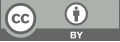
Analyzing Diffusion Models: Transformer Integration and Prompt Optimization for Text-To-Image Generation
- 1 Courant institute of Mathematical Sciences, New York University, New York, USA
* Author to whom correspondence should be addressed.
Abstract
This paper delves into the analysis of various diffusion models, focusing on their utilization of text-conditional sampling and assessment using the contrastive language-image pre-training (CLIP) score as a metric. Leveraging Bidirectional Transformers (BERT) and Vision Transformers (ViT) within the CLIP model framework, it crucially compares models incorporating transformer blocks with those that do not. Specifically, it examines the structures of latent diffusion models and diffusion transformer models, emphasizing the use of Unet and Transformer block decoders, respectively. Additionally, the study explores different prompts to generate similar images, aiming to optimize conditioning training for text-to-image generation. Furthermore, the paper investigates the performance of the Latent Diffusion Model (LDM) and the DiT model in object generation, analyzing their interaction with text embeddings and prompt structures. The findings underscore the significance of prompt structures on model performance, with implications for future research into multimodal models and prompt optimization.
Keywords
Diffusion models, Transformer integration, Prompt optimization, Text-to-image generation
[1]. Li A. C, Prabhudesai, M., Duggal, S., Brown, E., & Pathak, D. (2023). Your diffusion model is secretly a zero-shot classifier. In Proceedings of the IEEE/CVF International Conference on Computer Vision (pp. 2206-2217).
[2]. Bond-Taylor S, Leach A, Long Y, & Willcocks C. G. (2021). Deep generative modelling: A comparative review of vaes, gans, normalizing flows, energy-based and autoregressive models. IEEE transactions on pattern analysis and machine intelligence, 44(11), 7327-7347.
[3]. Ho J, Jain A, & Abbeel P. (2020). Denoising diffusion probabilistic models. Advances in neural information processing systems, 33, 6840-6851.
[4]. Rombach R, Blattmann A, Lorenz D, Esser P, & Ommer B. (2022). High-resolution image synthesis with latent diffusion models. In Proceedings of the IEEE/CVF conference on computer vision and pattern recognition (pp. 10684-10695).
[5]. Vaswani A, Shazeer N, Parmar N, Uszkoreit J, Jones L, Gomez A. N, ... & Polosukhin I (2017). Attention is all you need. Advances in neural information processing systems, 30.
[6]. Peebles W, & Xie S (2023). Scalable diffusion models with transformers. In Proceedings of the IEEE/CVF International Conference on Computer Vision (pp. 4195-4205).
[7]. Radford A, Kim J. W, Hallacy C, Ramesh A, Goh G, Agarwal S, ... & Sutskever I. (2021, July). Learning transferable visual models from natural language supervision. In International conference on machine learning (pp. 8748-8763). PMLR.
[8]. Hessel J, Holtzman A, Forbes M, Bras R. L, & Choi Y (2021). Clipscore: A reference-free evaluation metric for image captioning. arXiv preprint arXiv:2104.08718.
[9]. Devlin J, Chang M. W, Lee K, & Toutanova K (2018). Bert: Pre-training of deep bidirectional transformers for language understanding. arXiv preprint arXiv:1810.04805.
[10]. Dosovitskiy A, Beyer L, Kolesnikov A, Weissenborn D, Zhai X, Unterthiner T, ... & Houlsby N (2020). An image is worth 16x16 words: Transformers for image recognition at scale. arXiv preprint arXiv:2010.11929.
[11]. Krizhevsky A, & Hinton G (2009). Learning multiple layers of features from tiny images.
[12]. Deng J, Dong W, Socher R, Li L. J, Li K, & Li F (2009, June). Imagenet: A large-scale hierarchical image database. In 2009 IEEE conference on computer vision and pattern recognition (pp. 248-255). Ieee.
Cite this article
Tang,Q. (2025). Analyzing Diffusion Models: Transformer Integration and Prompt Optimization for Text-To-Image Generation. Applied and Computational Engineering,108,175-181.
Data availability
The datasets used and/or analyzed during the current study will be available from the authors upon reasonable request.
Disclaimer/Publisher's Note
The statements, opinions and data contained in all publications are solely those of the individual author(s) and contributor(s) and not of EWA Publishing and/or the editor(s). EWA Publishing and/or the editor(s) disclaim responsibility for any injury to people or property resulting from any ideas, methods, instructions or products referred to in the content.
About volume
Volume title: Proceedings of the 5th International Conference on Signal Processing and Machine Learning
© 2024 by the author(s). Licensee EWA Publishing, Oxford, UK. This article is an open access article distributed under the terms and
conditions of the Creative Commons Attribution (CC BY) license. Authors who
publish this series agree to the following terms:
1. Authors retain copyright and grant the series right of first publication with the work simultaneously licensed under a Creative Commons
Attribution License that allows others to share the work with an acknowledgment of the work's authorship and initial publication in this
series.
2. Authors are able to enter into separate, additional contractual arrangements for the non-exclusive distribution of the series's published
version of the work (e.g., post it to an institutional repository or publish it in a book), with an acknowledgment of its initial
publication in this series.
3. Authors are permitted and encouraged to post their work online (e.g., in institutional repositories or on their website) prior to and
during the submission process, as it can lead to productive exchanges, as well as earlier and greater citation of published work (See
Open access policy for details).