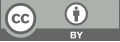
Research on big data privacy protection technology
- 1 Wuhan Sannew School, Wuhan, Hubei, China, 430090
* Author to whom correspondence should be addressed.
Abstract
The extensive application of big data technology makes data burst with unprecedented value and vitality. However, due to the large amount of data, many data sources, and complex data access relationships, the current development of privacy protection technology is seriously lagging behind the development of big data technology, which restricts the application and promotion of big data. At present, it is urgent to sort out the development status of big data privacy protection technology, so as to provide a reference for the research and breakthrough of key issues of big data privacy protection. This paper analyzes k-anonymity and differential privacy protection, and points out that a protection method that can reduce the cost of high-dimensional data processing and ignore the background knowledge requirements of attackers is urgently needed for privacy protection in big data scenarios. At the same time, this paper also puts forward suggestions for the application and future development of these technologies.
Keywords
Big Data, Privacy Protection, Data Privacy
[1]. Sweeney L. k-anonymity: a model for protecting privacy. Int J Unc Fuzz Knowl Based Syst, 2002, 10: 557–570
[2]. Bu Y, Fu A W C, Wong R C W, et al. Privacy preserving serial data publishing by role composition. Proc VLDB Endow, 2008, 1: 845–856
[3]. Fu Y Y, Zhang M, Feng D G, et al. Attribute privacy preservation in social networks based on node anatomy. J Sotfw, 2014, 25: 768–780
[4]. Yuan M X, Chen L, Philip S Y, et al. Protecting sensitive labels in social network data anonymization. IEEE Trans Knowl Data Eng, 2013, 25: 633–647
[5]. Gruteser M, Grunwald D. Anonymous usage of location-based services through spatial and temporal cloaking. In: Proceedings of the 1st International Conference on Mobile Systems, Applications and Services, San Francisco, 2003. 31–42
[6]. Huo Z, Meng X F. A trajectory data publication method under differential privacy. Chin J Comput, 2018, 41: 400–412
[7]. Dwork C. Differential privacy. In: Proceedings of the 33rd International Colloquium on Automata, Languages and Programming, Venice, 2006. 1–12
[8]. Friedman A, Schuster A. Data mining with differential privacy. In: Proceedings of the 16th ACM SIGKDD Interna- tional Conference on Knowledge Discovery and Data Mining, Washington, 2010. 493–502
[9]. Warner S L. Randomized response: a survey technique for eliminating evasive answer bias. J Am Stat Assoc, 1965, 60: 63–69
Cite this article
Lu,T. (2023). Research on big data privacy protection technology. Applied and Computational Engineering,4,642-644.
Data availability
The datasets used and/or analyzed during the current study will be available from the authors upon reasonable request.
Disclaimer/Publisher's Note
The statements, opinions and data contained in all publications are solely those of the individual author(s) and contributor(s) and not of EWA Publishing and/or the editor(s). EWA Publishing and/or the editor(s) disclaim responsibility for any injury to people or property resulting from any ideas, methods, instructions or products referred to in the content.
About volume
Volume title: Proceedings of the 3rd International Conference on Signal Processing and Machine Learning
© 2024 by the author(s). Licensee EWA Publishing, Oxford, UK. This article is an open access article distributed under the terms and
conditions of the Creative Commons Attribution (CC BY) license. Authors who
publish this series agree to the following terms:
1. Authors retain copyright and grant the series right of first publication with the work simultaneously licensed under a Creative Commons
Attribution License that allows others to share the work with an acknowledgment of the work's authorship and initial publication in this
series.
2. Authors are able to enter into separate, additional contractual arrangements for the non-exclusive distribution of the series's published
version of the work (e.g., post it to an institutional repository or publish it in a book), with an acknowledgment of its initial
publication in this series.
3. Authors are permitted and encouraged to post their work online (e.g., in institutional repositories or on their website) prior to and
during the submission process, as it can lead to productive exchanges, as well as earlier and greater citation of published work (See
Open access policy for details).