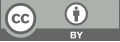
A Study on ChatGPT-Based Code Translation from Python to Java
- 1 Beijing University of Posts and Telecommunications, Beijing, 100876, China
* Author to whom correspondence should be addressed.
Abstract
Programming language translation is essential in modern software development, facilitating cross-platform compatibility and the adaptation of legacy systems. This study examines the performance of large language models (LLMs), such as ChatGPT, in Python-to-Java code translation. Using a dataset of ten diverse algorithmic problems and advanced prompt engineering techniques, we evaluate the models’ effectiveness in maintaining computational accuracy (CA) and preserving method correctness (PMC). Results indicate that LLMs perform well on standard tasks but encounter challenges in complex scenarios involving advanced data structures and recursion. These findings uncover the potential of LLMs in code translation while highlighting the need for improved prompt strategies and domain-specific fine-tuning for complex tasks.
Keywords
Large Language Models, Code Translation, Python-to-Java, Prompt Engineering, ChatGPT
[1]. Zhang, Z., Chen, C., Liu, B., Liao, C., Gong, Z., Yu, H., Li, J., & Wang, R. (2023). Unifying the Perspectives of NLP and Software Engineering: A Survey on Language Models for Code. arXiv preprint arXiv:2311.07989. Retrieved from https://arxiv.org/abs/2311.07989
[2]. Li, M., Mishra, A., & Mujumdar, U. (2024). Bridging the Language Gap: Enhancing Multilingual Prompt-Based Code Generation in LLMs via Zero-Shot Cross-Lingual Transfer. arXiv preprint arXiv:2408.09701. Retrieved from https://arxiv.org/abs/2408.09701
[3]. Roziere, B., Lachaux, M.-A., Chanussot, L., & Lample, G. (2020). Unsupervised Translation of Programming Languages. arXiv preprint arXiv:2006.03511. Retrieved from https://arxiv.org/abs/2006.035114.
[4]. Chen, M., Tworek, J., Jun, H., Yuan, Q., Dehghani, M., Flores, G., ... & Zoph, B. (2021). Evaluating Large Language Models Trained on Code. arXiv preprint arXiv:2107.03374. Retrieved from https://arxiv.org/abs/2107.033745.
[5]. B. Roziere, M.-A. Lachaux, M. Szafraniec, and G. Lample, “DOBF: A Deobfuscation Pre-Training Objective for Programming Languages, ” arXiv preprint arXiv:2102.07492, 2021. Retrieved from https://arxiv.org/abs/2102.07492.
[6]. Vaswani, A., Shazeer, N., Parmar, N., Uszkoreit, J., Jones, L., Gomez, A. N., Kaiser, Ł., & Polosukhin, I. (2017). Attention Is All You Need. In Proceedings of the 31st International Conference on Neural Information Processing Systems (NIPS 2017) (pp. 6000–6010). arXiv preprint arXiv:1706.03762. Retrieved from https://arxiv.org/abs/1706.03762.
[7]. Feng, Z., Guo, D., Tang, D., Duan, N., Feng, X., Gong, M., ... & Zhou, M. (2020). CodeBERT: A Pre-Trained Model for Programming and Natural Languages. arXiv preprint arXiv:2002.08155. Retrieved from https://arxiv.org/abs/2002.08155.
[8]. Ahmad, W. U., Chakraborty, S., Ray, B., & Chang, K. W. (2021). Unified Pre-training for Program Understanding and Generation. arXiv preprint arXiv:2103.06333. Retrieved from https://arxiv.org/abs/2103.06333.
[9]. Weisz, J. D., Muller, M., Ross, S. I., et al. Better together? An evaluation of AI-supported code translation. Proceedings of the 27th International Conference on Intelligent User Interfaces. 2022: 369-391.Retrieved from https://research.ibm.com/publications/better-together-an-evaluation-of-ai-supported-code-translation.
[10]. Tufano, M., Watson, C., Bavota, G., Di Penta, M., White, M., & Poshyvanyk, D. (2019). An Empirical Study on Learning Bug-Fixing Patches in the Wild via Neural Machine Translation. ACM Transactions on Software Engineering and Methodology (TOSEM), 28(4), 1-29. Retrieved from https://doi.org/10.1145/3340544
[11]. Guo, D., Xu, S., Zhao, Z., Li, Z., Wang, H., & Hu, X. (2022). GraphCodeBERT: Pre-trained Code Representation Learning with Data Flow. arXiv preprint arXiv:2009.08366. Retrieved from https://arxiv.org/abs/2009.08366
[12]. Allamanis, M., Barr, E. T., Bird, C., & Sutton, C. (2018). A Survey of Machine Learning for Big Code and Naturalness. ACM Computing Surveys (CSUR), 51(4), 1-37. Retrieved from https://doi.org/10.1145/3212695
[13]. Raffel, C., Shazeer, N., Roberts, A., Lee, K., Narang, S., Matena, M., ... & Liu, P. J. (2020). Exploring the Limits of Transfer Learning with a Unified Text-to-Text Transformer. Journal of Machine Learning Research, 21(140), 1-67. Retrieved from https://arxiv.org/abs/1910.10683
Cite this article
Gao,X. (2025). A Study on ChatGPT-Based Code Translation from Python to Java. Applied and Computational Engineering,108,26-34.
Data availability
The datasets used and/or analyzed during the current study will be available from the authors upon reasonable request.
Disclaimer/Publisher's Note
The statements, opinions and data contained in all publications are solely those of the individual author(s) and contributor(s) and not of EWA Publishing and/or the editor(s). EWA Publishing and/or the editor(s) disclaim responsibility for any injury to people or property resulting from any ideas, methods, instructions or products referred to in the content.
About volume
Volume title: Proceedings of the 5th International Conference on Signal Processing and Machine Learning
© 2024 by the author(s). Licensee EWA Publishing, Oxford, UK. This article is an open access article distributed under the terms and
conditions of the Creative Commons Attribution (CC BY) license. Authors who
publish this series agree to the following terms:
1. Authors retain copyright and grant the series right of first publication with the work simultaneously licensed under a Creative Commons
Attribution License that allows others to share the work with an acknowledgment of the work's authorship and initial publication in this
series.
2. Authors are able to enter into separate, additional contractual arrangements for the non-exclusive distribution of the series's published
version of the work (e.g., post it to an institutional repository or publish it in a book), with an acknowledgment of its initial
publication in this series.
3. Authors are permitted and encouraged to post their work online (e.g., in institutional repositories or on their website) prior to and
during the submission process, as it can lead to productive exchanges, as well as earlier and greater citation of published work (See
Open access policy for details).